Aspect Term Extraction Based On Mfe-Crf
INFORMATION(2018)
摘要
This paper is focused on aspect term extraction in aspect-based sentiment analysis (ABSA), which is one of the hot spots in natural language processing (NLP). This paper proposes MFE-CRF that introduces Multi-Feature Embedding (MFE) clustering based on the Conditional Random Field (CRF) model to improve the effect of aspect term extraction in ABSA. First, Multi-Feature Embedding (MFE) is proposed to improve the text representation and capture more semantic information from text. Then the authors use kmeans++ algorithm to obtain MFE and word clustering to enrich the position features of CRF. Finally, the clustering classes of MFE and word embedding are set as the additional position features to train the model of CRF for aspect term extraction. The experiments on SemEval datasets validate the effectiveness of this model. The results of different models indicate that MFE-CRF can greatly improve the Recall rate of CRF model. Additionally, the Precision rate also is increased obviously when the semantics of text is complex.
更多查看译文
关键词
sentiment analysis, aspect term extraction, MFE, CRF, kmeans++ algorithm
AI 理解论文
溯源树
样例
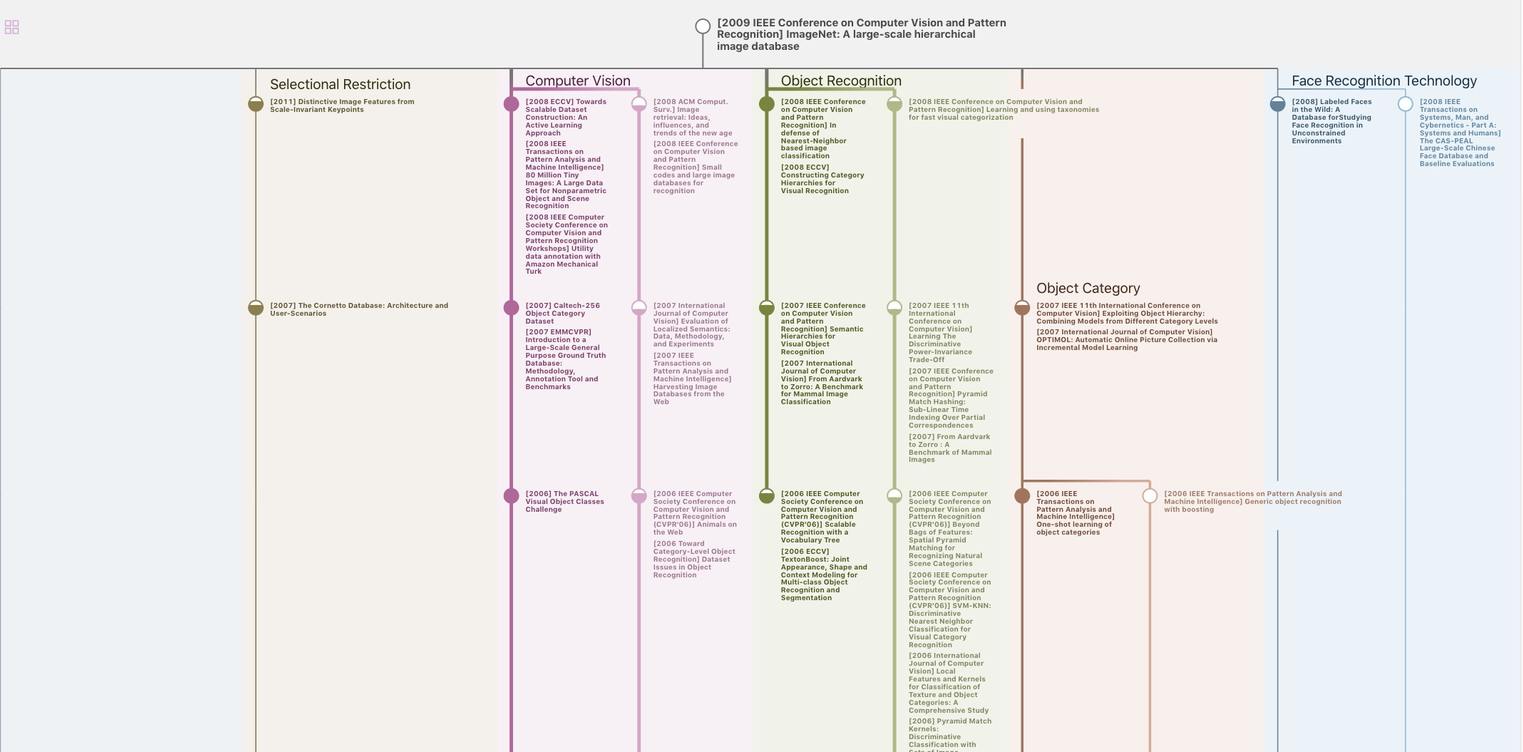
生成溯源树,研究论文发展脉络
Chat Paper
正在生成论文摘要