An Intrusion Detection System Using a Deep Neural Network With Gated Recurrent Units.
IEEE ACCESS(2018)
摘要
To improve the performance of network intrusion detection systems (IDS), we applied deep learning theory to intrusion detection and developed a deep network model with automatic feature extraction. In this paper, we consider the characteristics of the time-related intrusion and propose a novel IDS that consists of a recurrent neural network with gated recurrent units (GRU), multilayer perceptron (MLP), and softmax module. Experiments on the well-known KDD 99 and NSL-KDD data sets show that the system has leading performance. The overall detection rate was 99.42% using KDD 99 and 99.31% using NSL-KDD with false positive rates as low as 0.05% and 0.84%, respectively. In particular, for detecting the denial of service attacks, the system achieved detection rates of 99.98% and 99.55%, respectively. Comparative experiments showed that the GRU is more suitable as a memory unit for IDS than LSTM, and proved that it is an effective simplification and improvement of LSTM. Moreover, the bidirectional GRU can reach the best performance compared with the recently published methods.
更多查看译文
关键词
Intrusion detection,deep learning,recurrent neural network,gated recurrent unit
AI 理解论文
溯源树
样例
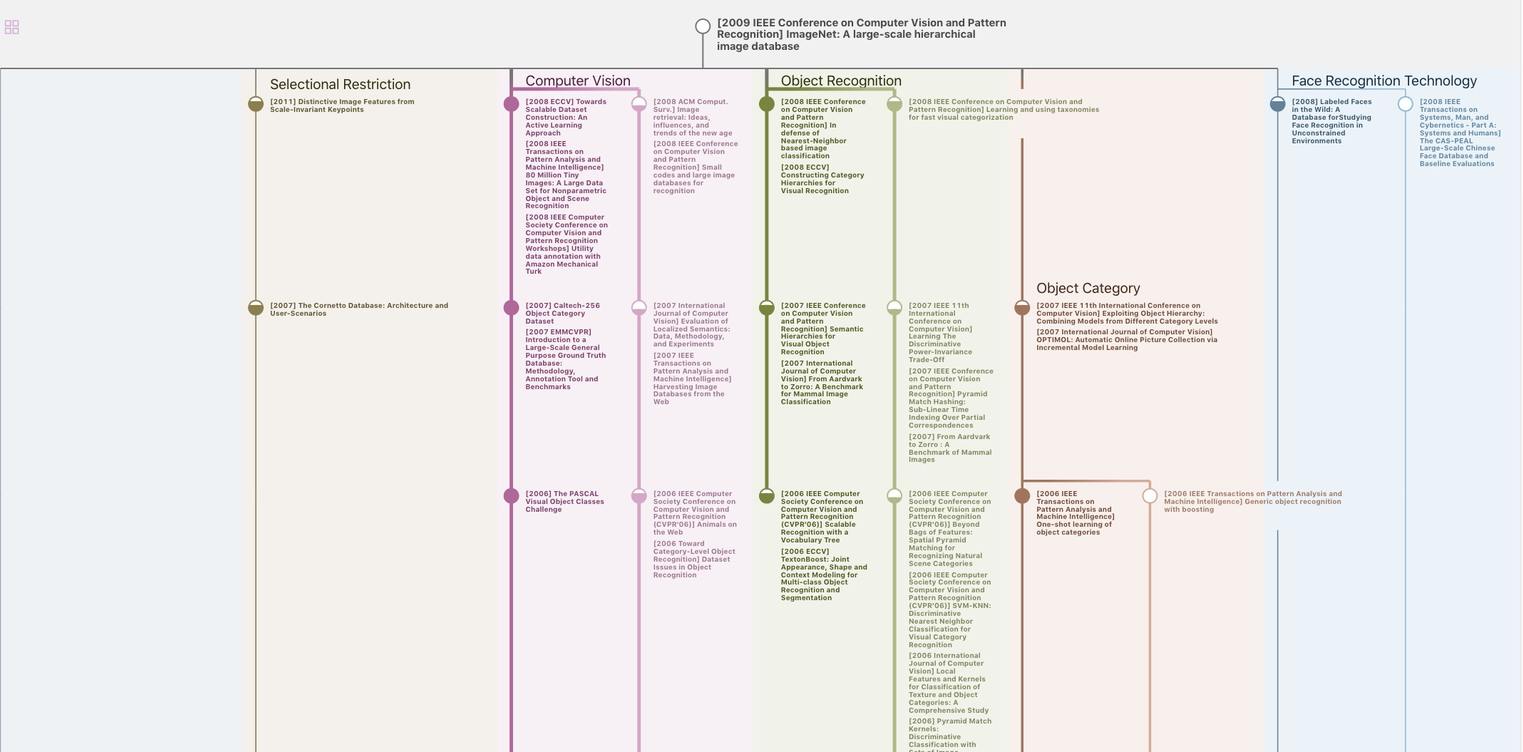
生成溯源树,研究论文发展脉络
Chat Paper
正在生成论文摘要