Symmetric low-rank representation with adaptive distance penalty for semi-supervised learning.
Neurocomputing(2018)
摘要
Graph-based approaches have been successfully used in semi-supervised learning (SSL) by weighting the affinity between the corresponding pairs of samples. Low-rank representation (LRR) is one of the state-of-the-art methods, has been extensively employed in the existing graph-based learning models. In graph-based SSL, a weighted affinity graph induced by LRR coefficients is used to reflect the relationships among data samples. Constructing a robust and discriminative graph to discover the intrinsic structures of the data is critical for SSL, because the central idea behind the graph-based SSL approaches is to explore the pairwise affinity between data samples to infer those unknown labels. However, most of existing LRR-based approaches fail to guarantee weight consistency for each pair of data samples, which is beneficial to preserve the subspace structures of high dimensional data. In this paper, we propose a symmetric LRR with adaptive distance penalty (SLRRADP) method for the small sample size (SSS) recognition problem. The graph identified by SLRRADP can not only preserve global and local structures of data, but also exploit intrinsic correlation information of data samples. Extensive experimental results on semi-supervised classification tasks demonstrate the effectiveness of the proposed method and its superiority in performance over the state-of-the-art graph construction approaches.
更多查看译文
关键词
Low-rank representation,Semi-supervised learning,Graph construction,Image classification
AI 理解论文
溯源树
样例
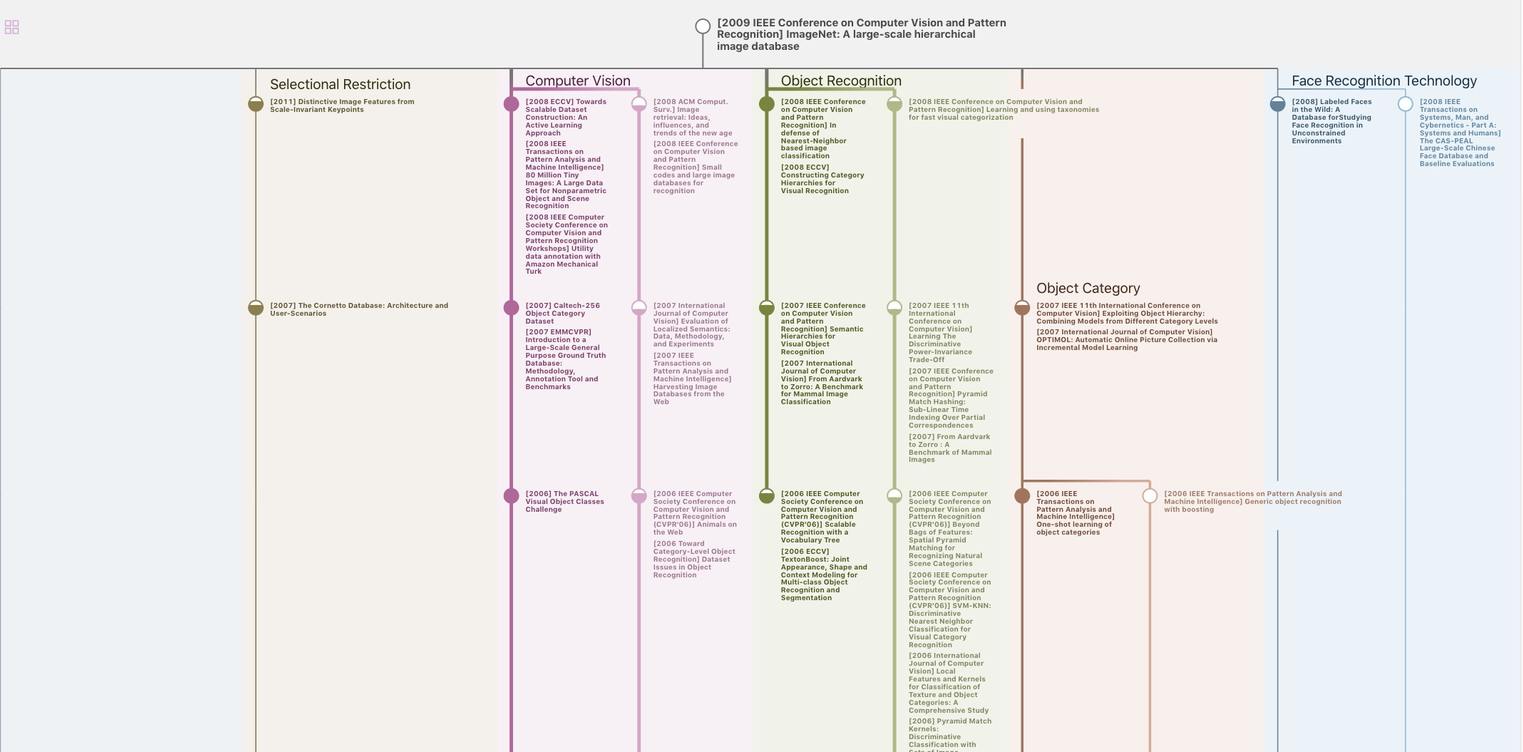
生成溯源树,研究论文发展脉络
Chat Paper
正在生成论文摘要