A General Framework and Metrics for Longitudinal Data Anonymization.
PSD(2018)
摘要
The bulk of methods in statistical disclosure control primarily deal with individual data from a cross-sectional perspective, i.e. data where individuals are observed at one single point in time. However, nowadays longitudinal data, i.e. individuals observed over multiple periods, are increasingly collected. Such data enhance undoubtedly the possibility of statistical analysis compared to cross-sectional data, but also come with some additional layers of information that have to remain practically useful in a privacy-preserving way. Building on the recently proposed permutation paradigm as an overarching approach to data anonymization, this paper establishes a general framework for the formulation of longitudinal data anonymization and proposes some universal metrics for the assessment of disclosure risk and information loss. We illustrate the application of these new tools using an empirical example.
更多查看译文
关键词
Statistical disclosure control, Longitudinal data, Permutation paradigm
AI 理解论文
溯源树
样例
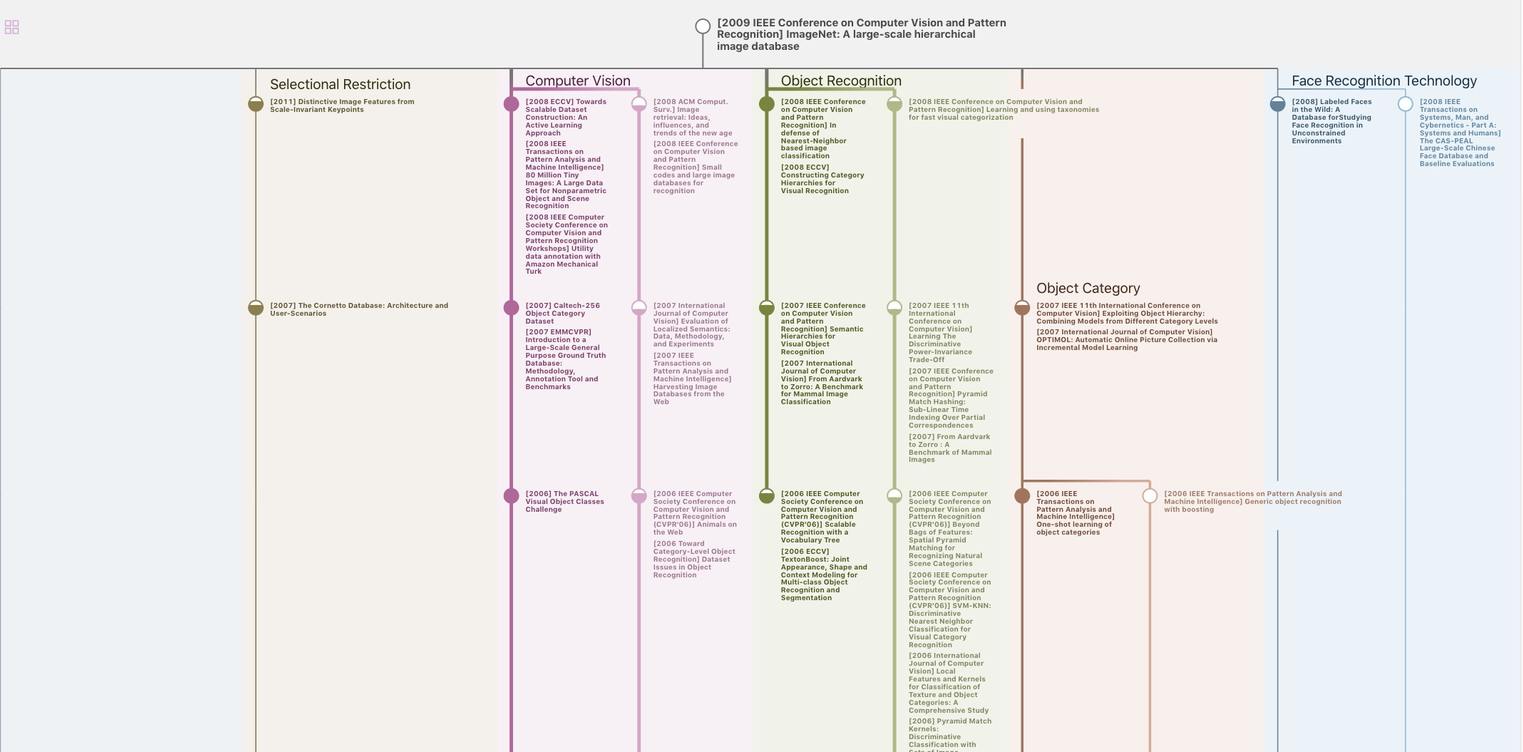
生成溯源树,研究论文发展脉络
Chat Paper
正在生成论文摘要