The Application of Genetic Algorithms to Data Synthesis: A Comparison of Three Crossover Methods.
PSD(2018)
摘要
Data synthesis is a data confidentiality method which is applied to microdata to prevent leakage of sensitive information about respondents. Instead of publishing real data, data synthesis produces an artificial dataset that does not contain the real records of respondents. This, in particular, offers significant protection against reidentification attacks. However, effective data synthesis requires retention of the key statistical properties of (and respecting the multiple utilities of) the original data. In previous work, we demonstrated the value of matrix genetic algorithms in data synthesis [4]. The current paper compares three crossover methods within a matrix GA: parallelised (two-point) crossover, matrix crossover, and parametric uniform crossover. The crossover methods are applied to three different datasets and are compared on the basis of how well they reproduce the relationships between variables in the original datasets.
更多查看译文
关键词
Genetic algorithms, Data synthesis, Data privacy
AI 理解论文
溯源树
样例
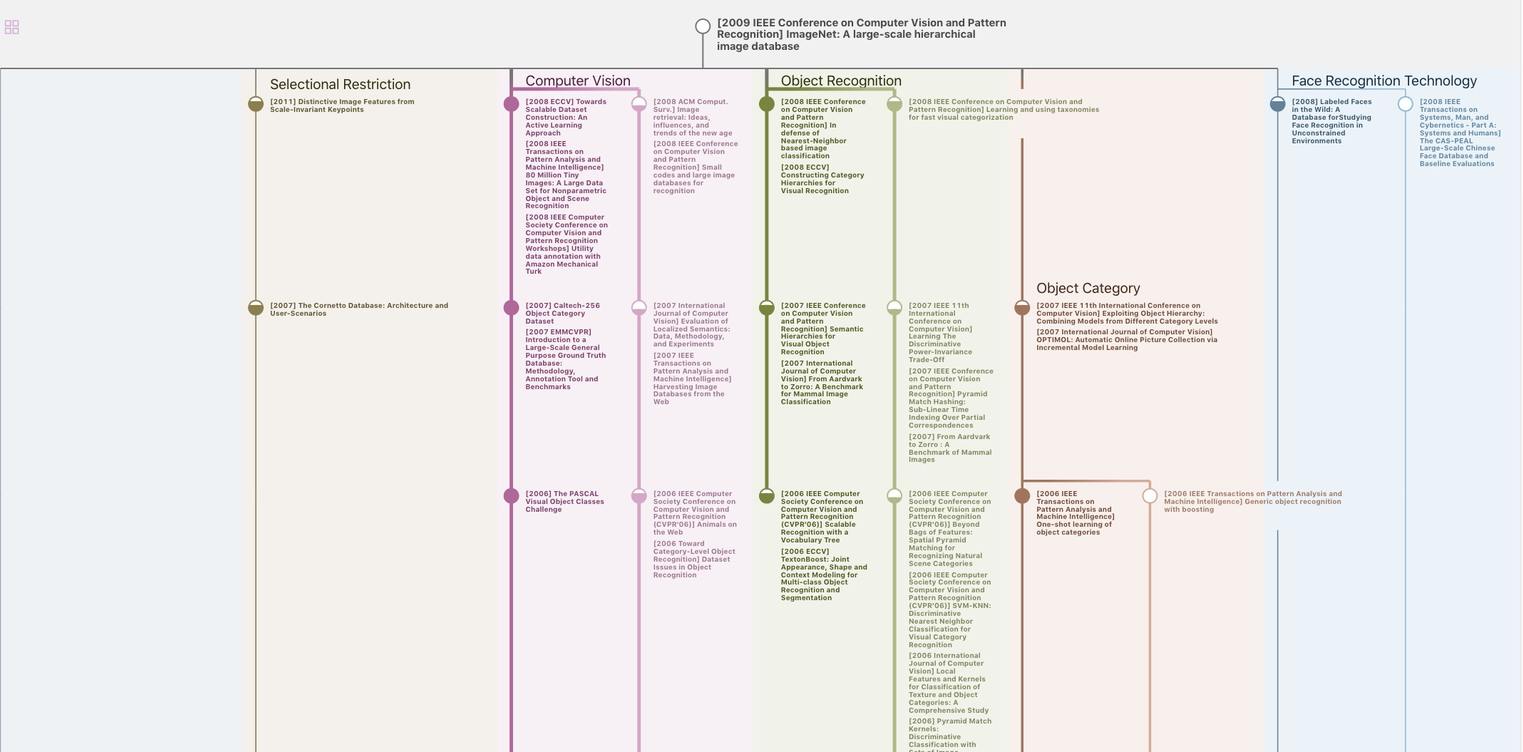
生成溯源树,研究论文发展脉络
Chat Paper
正在生成论文摘要