Deep Learning-Based Sinogram Completion For Low-Dose Ct
PROCEEDINGS 2018 IEEE 13TH IMAGE, VIDEO, AND MULTIDIMENSIONAL SIGNAL PROCESSING WORKSHOP (IVMSP)(2018)
摘要
Patient radiation dose associated with X-ray CT is a significant concern in the medical community. One of the ways to reduce patient dose is to acquire projection data at fewer angles. Using conventional reconstruction methods with such sparsely sampled data introduces severe streaking artifacts in the reconstructions, that reduces their diagnostic utility. Conventional methods in this domain have focused on post-processing the artifact-filled images or the use of model-based inversion techniques with image-domain priors. In this work, we examine the potential of a deep-learning-based method to construct a mapping from the observed, sparsely sampled, CT projection data to a set of densely sampled projection estimates directly in the original projection domain. We cast the problem as one of sinogram in-painting and focus on completing the projection data prior to reconstructing the images. As compared to existing work, we focus on "correcting" the data rather than the subsequent images and avoid costly iterative tomographic inversion. Our initial results on a simulated dataset demonstrate the potential effectiveness of this new approach in suppressing artifacts.
更多查看译文
关键词
Low-dose, Sparse-view, Convolutional neural networks, Deep learning, Computed tomography
AI 理解论文
溯源树
样例
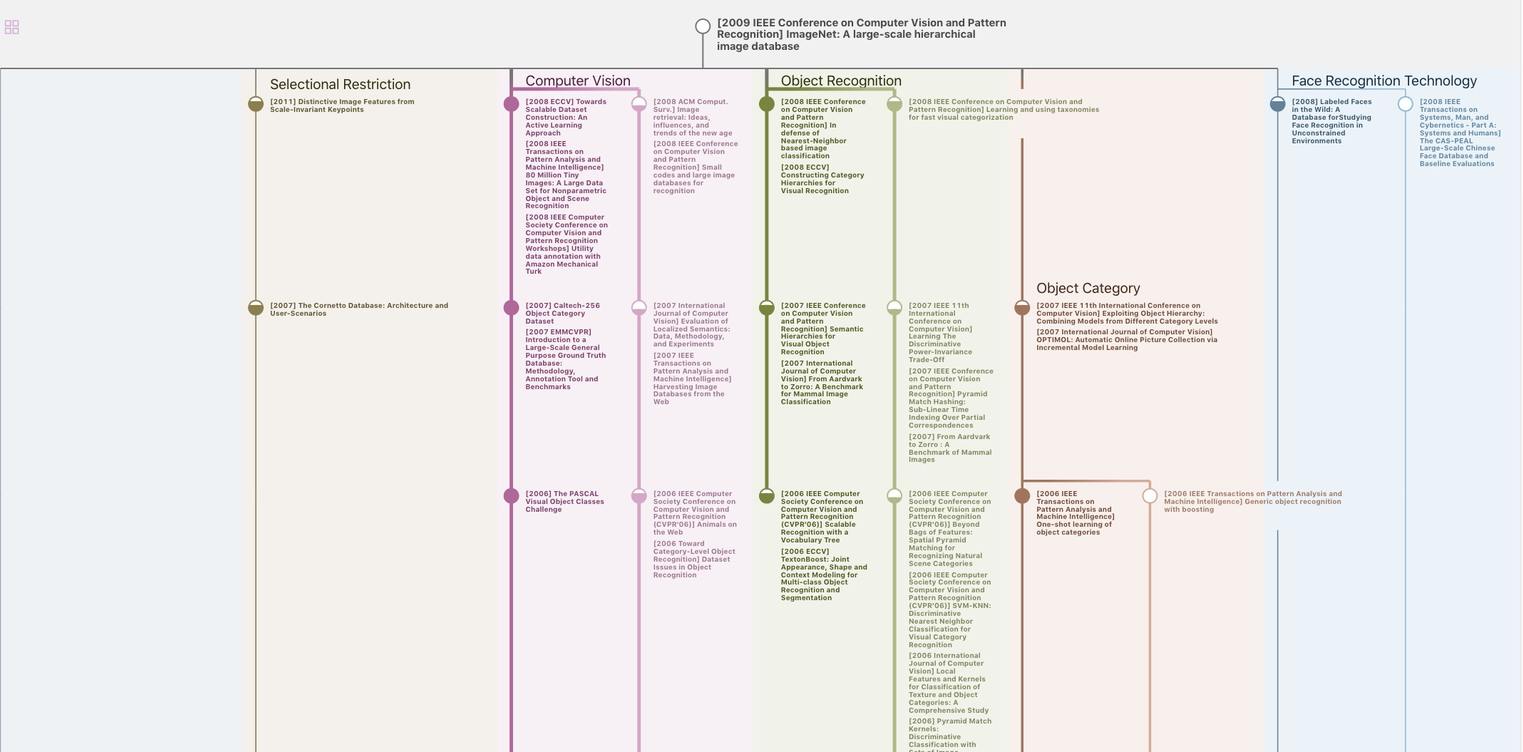
生成溯源树,研究论文发展脉络
Chat Paper
正在生成论文摘要