Anomaly Detection using Resource Behaviour Analysis for Autoscaling systems
2018 4th IEEE Conference on Network Softwarization and Workshops (NetSoft)(2018)
摘要
In a cloud environment, autoscaling systems alleviate applications when additional resources are required. However, an illegitimate or malicious workload may force the system to automatically provision resources when they are not needed, thus leading to two key problems: economic denial of sustainability (eDoS) and wastage of resources. In this paper, we propose an anomaly detection mechanism using resource behaviour analysis to prevent these issues. We build univariate autoregressive statistical models to analyze resource behaviours for each microservice on the platform. The use of multiple models helps us discern unusual anomalies rather than a sudden increase in certain properties. We implemented the anomaly detection for the Elascale autoscaling engine on SAVI Testbed and evaluated the detection mechanisms against different attacks. From the results, we conclude that the models can accurately detect anomalous behaviour for applications (with cyclical trends) on the autoscaling platform.
更多查看译文
关键词
Elascale autoscaling engine,unusual anomalies,univariate autoregressive statistical models,anomaly detection mechanism,provision resources,malicious workload,illegitimate workload,additional resources,cloud environment,autoscaling systems,resource behaviour analysis,autoscaling platform,anomalous behaviour,detection mechanisms
AI 理解论文
溯源树
样例
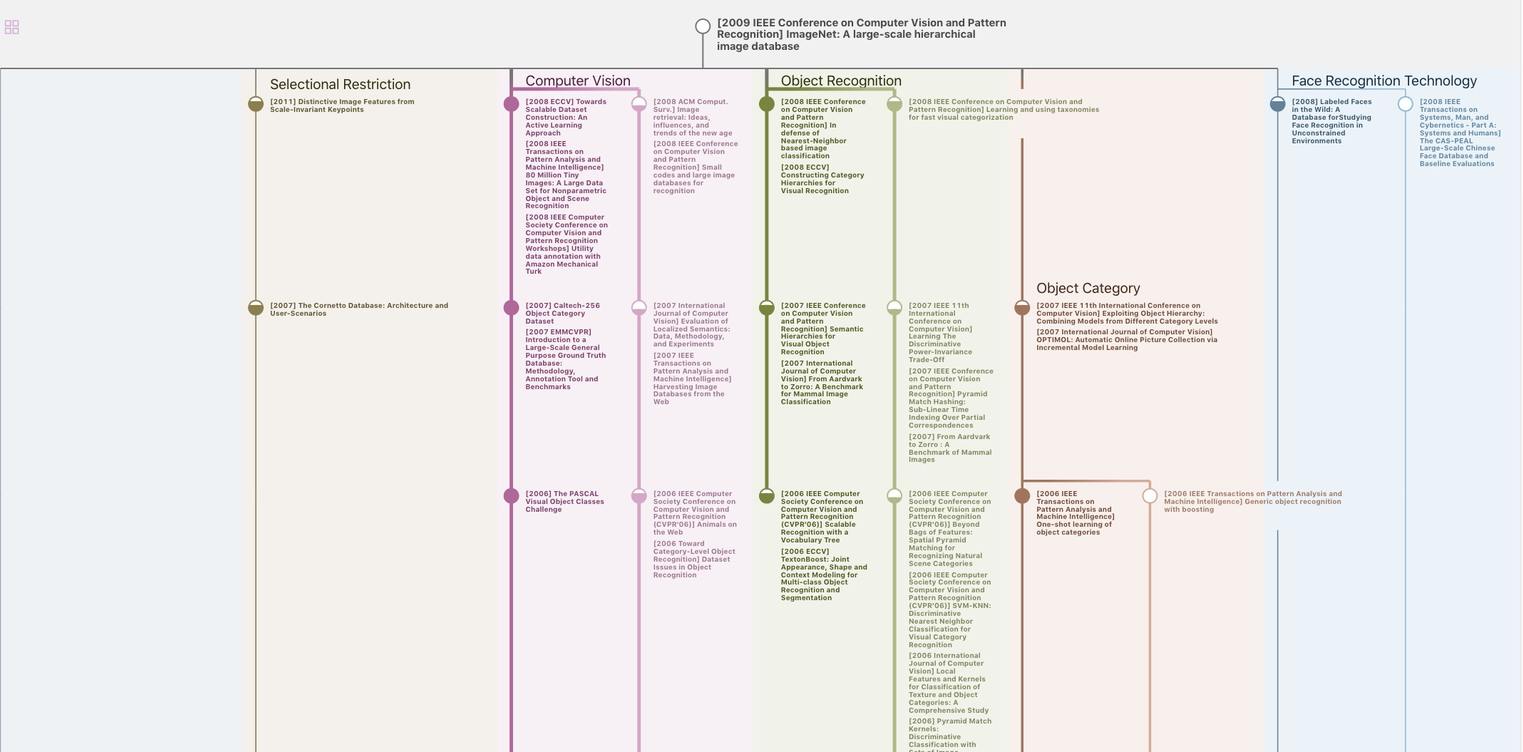
生成溯源树,研究论文发展脉络
Chat Paper
正在生成论文摘要