A Deep Top-K Relevance Matching Model For Ad-Hoc Retrieval
INFORMATION RETRIEVAL, CCIR 2018(2018)
摘要
In this paper, we propose a novel model named DTMM, which is specifically designed for ad-hoc retrieval. Given a query and a document, DTMM firstly builds an word-level interaction matrix based on word embeddings from query and document. At the same time, we also compress the embeddings of both document word and query word into a small dimension, to learn the importance of each word. Specifically, the compressed query word embedding is projected into the term gating network, and the compressed document word embedding is concatenated into the interaction matrix. Then, we apply the top-k pooling layer (i. e., ordered k-max pooling) on the interaction matrix, and get the essential top relevance signals. The top relevance signals is associated with each query term, and projected into a multi-layer perceptron neural network to obtain the query term level matching score. Finally, the query term level matching scores are aggregated with the term gating network to produce the final relevance score. We have tested our model on two representative benchmark datasets. Experimental results show that our model can significantly outperform existing baseline models.
更多查看译文
关键词
Deep learning, Relevance matching, Ad-hoc retrieval
AI 理解论文
溯源树
样例
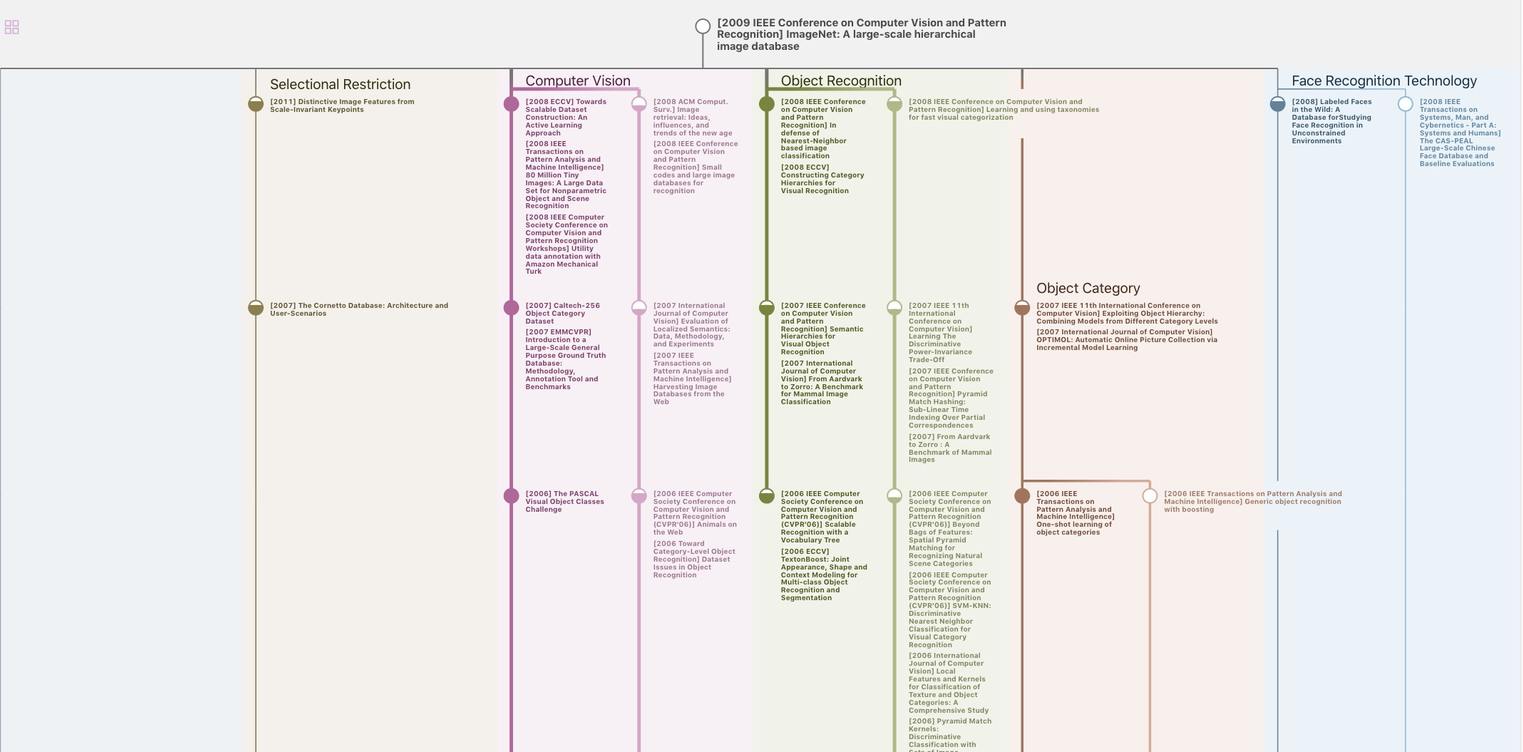
生成溯源树,研究论文发展脉络
Chat Paper
正在生成论文摘要