Generative Paragraph Vector
INFORMATION RETRIEVAL, CCIR 2018(2018)
摘要
The recently introduced Paragraph Vector (PV) is an efficient method for learning high-quality distributed representations for texts. However, from the probabilistic view, PV is not a complete model since it only models the generation of words but not texts, leading to two major limitations. Firstly, without a text-level model, PV assumes the independence between texts and thus cannot leverage the corpus-wide information to help text representation learning. Secondly, without the generation model of texts, the inference of text representations outside of the training set becomes difficult. Although PV makes itself as an optimization problem so that one can obtain representations for new texts anyway, it loses the sound probabilistic interpretability in that way. To tackle these problems, we first introduce a Generative Paragraph Vector, an extension of the Distributed Bag of Words version of Paragraph Vector with a complete generative process. By defining the generation model over texts, we further incorporate text labels into the model and turn it into a supervised version, namely Supervised Generative Paragraph Vector. Experiments on five text classification benchmark collections show that both unsupervised and supervised model architectures can yield superior classification performance against the state-of-the-art counterparts.
更多查看译文
关键词
Paragraph Vector (PV), Complete Generation Process, Corporate-wide Information, Text Representation Learning, Word Version
AI 理解论文
溯源树
样例
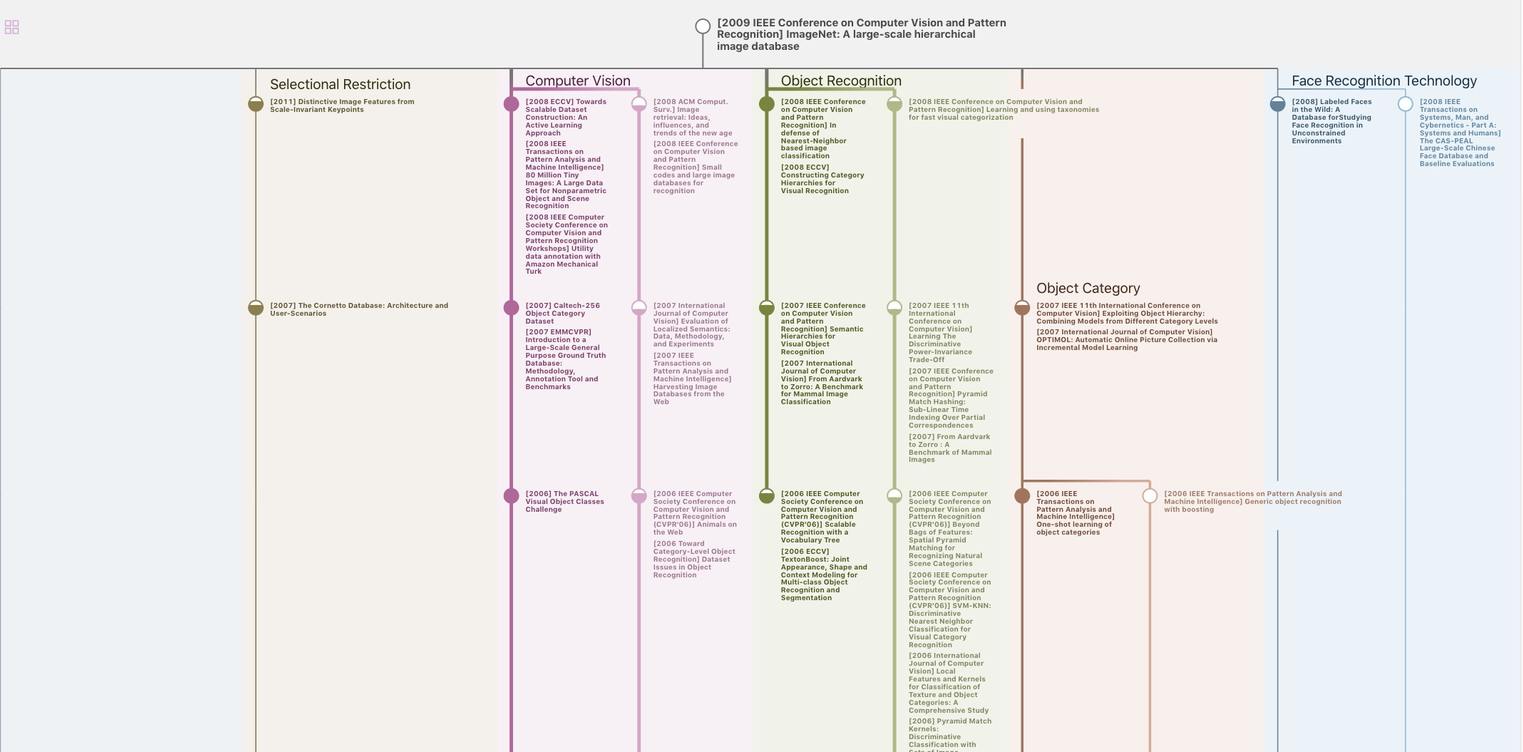
生成溯源树,研究论文发展脉络
Chat Paper
正在生成论文摘要