Power Missing Data Filling Based On Improved K-Means Algorithm And Rbf Neural Network
CLOUD COMPUTING AND SECURITY, PT V(2018)
摘要
Power data mainly comes from power generation, transmission, consumption, scheduling and statistics. However, in the process of power data acquisition, problems such as data missing seriously affect the further analysis. In this paper, we propose a missing data filling method based on improved k-Means clustering and Radial Basis Function neural network (kM-RBF) to solve the problem of missing power data. Firstly, the data samples are clustered by k-Means, and the clustering results are used as the parameters of RBF neural network. The RBF neural network is trained with the complete data samples, and then the missing values are predicted. In order to verify the effectiveness of the algorithm, we have chosen the power consumption and power generation metadata of each province in China for analysis and simulated the absence of data. Simulation results show that the kM-RBF can obtain higher accuracy of missing data filling.
更多查看译文
关键词
k-Means clustering algorithm, Missing data filling, Power system data, Self-adaptive weights, Radial basis function neural network
AI 理解论文
溯源树
样例
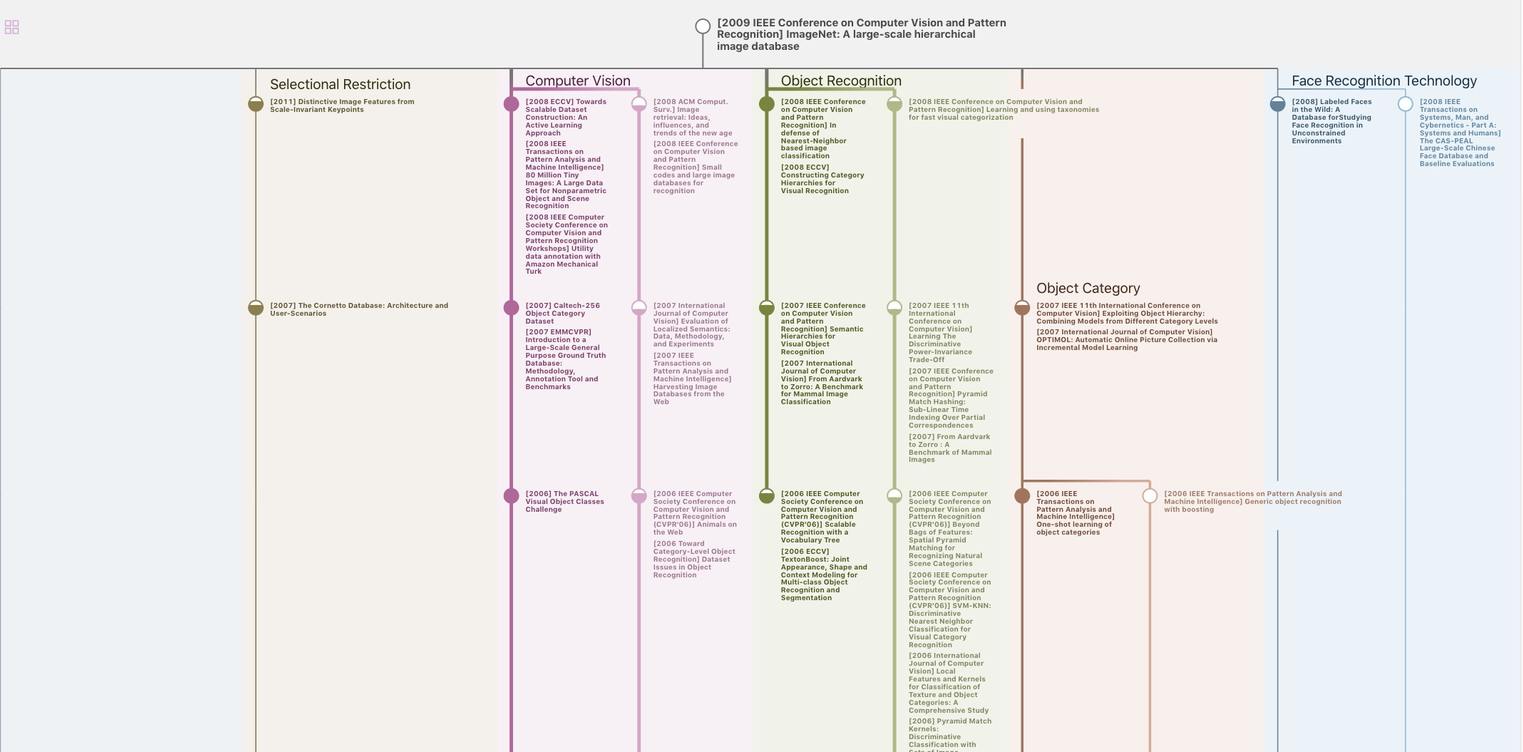
生成溯源树,研究论文发展脉络
Chat Paper
正在生成论文摘要