Granular Computing And Parameters Tuning In Imbalanced Data Preprocessing
COMPUTER INFORMATION SYSTEMS AND INDUSTRIAL MANAGEMENT, CISIM 2018(2018)
摘要
Selective preprocessing, representing data-level approach to the imbalanced data problem, is one of the most successful methods. This paper introduces novel algorithm combining this kind of technique with the filtering phase. The information granules are formed to distinguish specific types of positive examples that should be adequately treated. Three modes of oversampling, dedicated to minority class instances placed in specific areas of the feature space, are available. The rough set theory is applied to filter and remove inconsistencies from the generated positive samples. The experimental study shows that proposed method in most cases obtains better or similar performance of standard classifiers, such as C4.5 decision tree, in comparison with other techniques. Additionally, multiple values of algorithm's parameters are evaluated. It is experimentally proven that two of the examined parameters values are the most appropriate to various applications. However, the automatic parameters tuning, based on the specific requirements of different data distributions, is recommended.
更多查看译文
关键词
Data preprocessing, Imbalanced data, Rough sets, Oversampling, Parameters tuning, Information granules
AI 理解论文
溯源树
样例
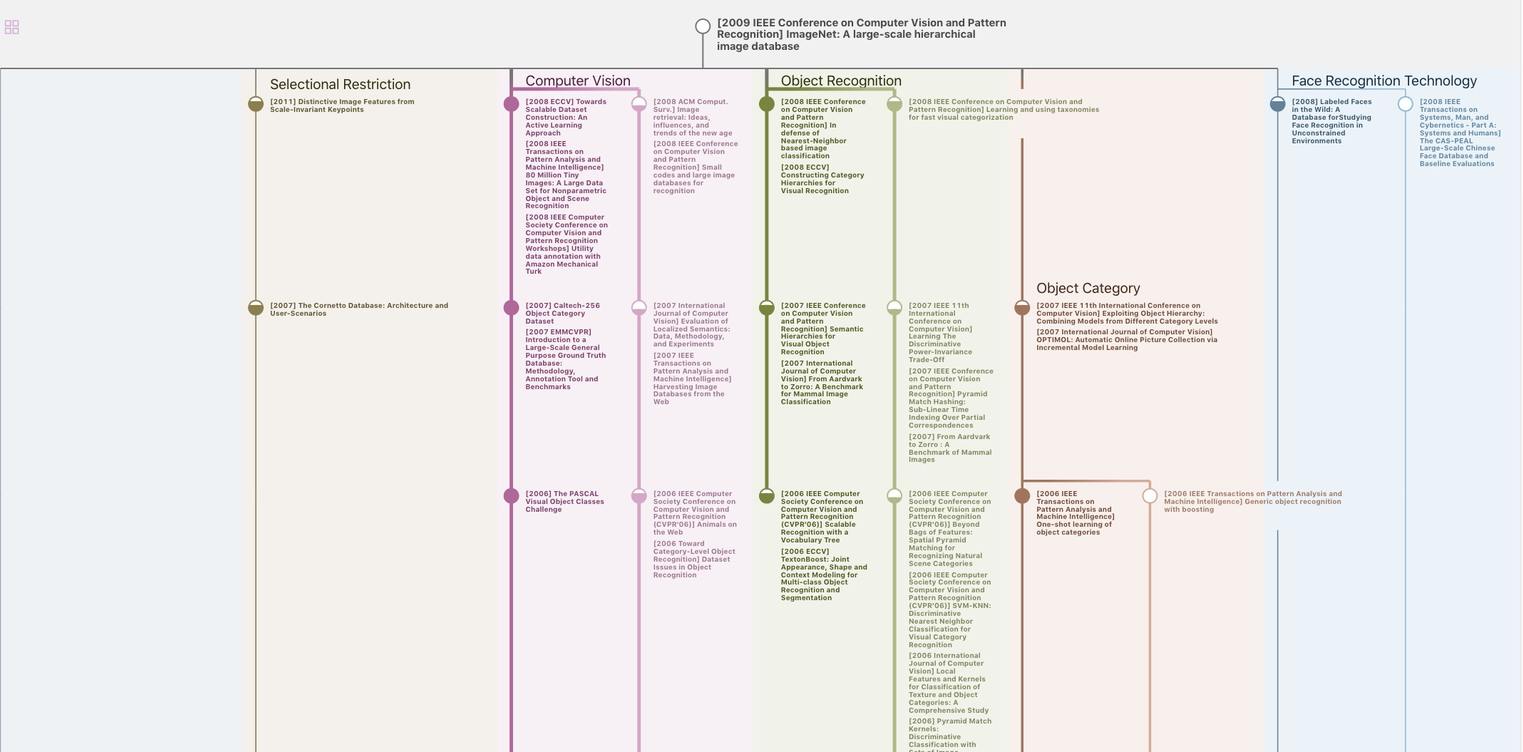
生成溯源树,研究论文发展脉络
Chat Paper
正在生成论文摘要