The Landscape Of Non-Convex Quadratic Feasibility
2018 IEEE INTERNATIONAL CONFERENCE ON ACOUSTICS, SPEECH AND SIGNAL PROCESSING (ICASSP)(2018)
摘要
Motivated by applications such as ordinal embedding and collaborative ranking, we formulate homogeneous quadratic feasibility as an unconstrained, non-convex minimization problem. Our work aims to understand the landscape (local minimizers and global minimizers) of the non-convex objective, which corresponds to hinge losses arising from quadratic constraints. Under certain assumptions, we give necessary conditions for non-global, local minimizers of our objective and additionally show that in two dimensions, every local minimizer is a global minimizer. Empirically, we demonstrate that finding feasible points by solving the unconstrained optimization problem with stochastic gradient descent works reliably by utilizing large initializations.
更多查看译文
关键词
Non-Convex Optimization, Preference Learning
AI 理解论文
溯源树
样例
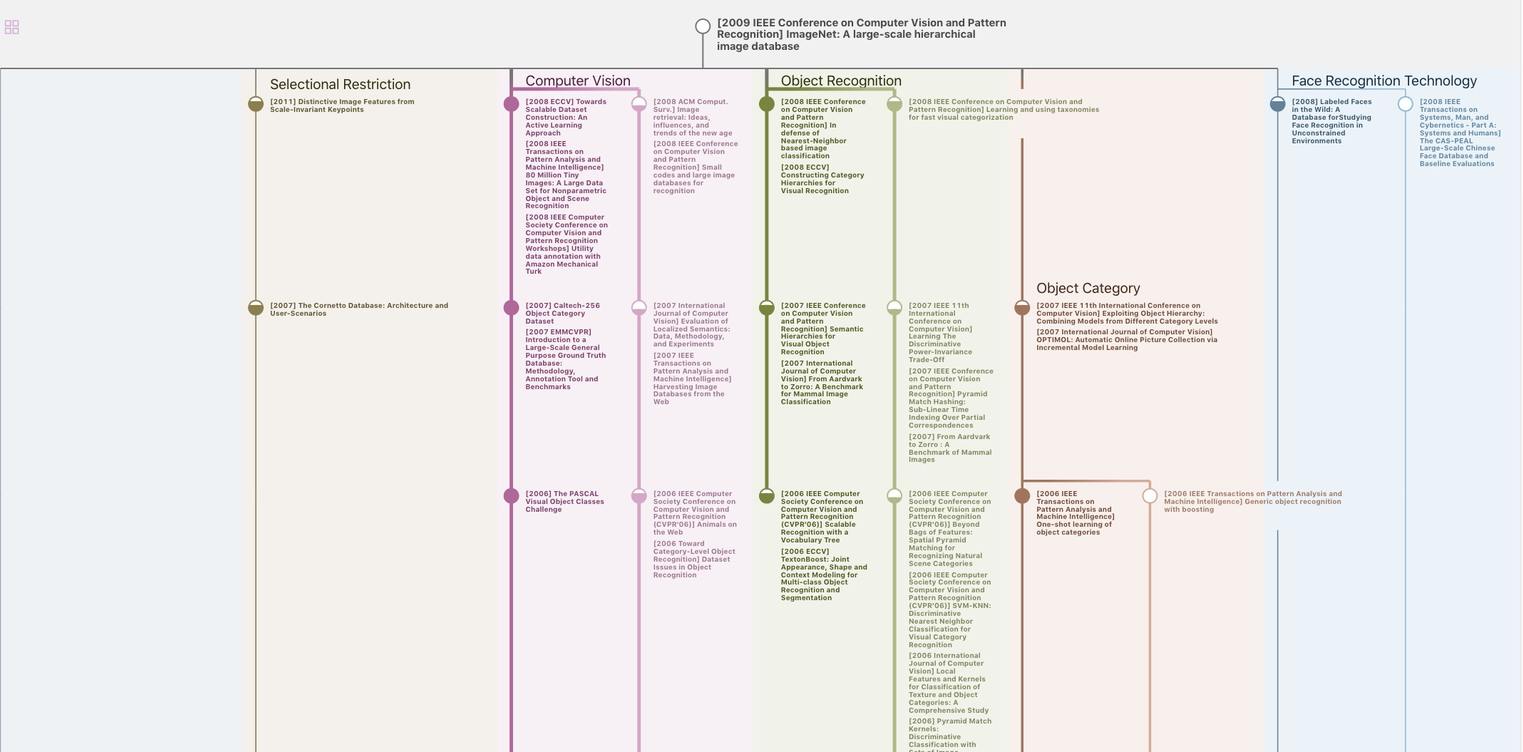
生成溯源树,研究论文发展脉络
Chat Paper
正在生成论文摘要