Time-Frequency Masking-Based Speech Enhancement Using Generative Adversarial Network
2018 IEEE INTERNATIONAL CONFERENCE ON ACOUSTICS, SPEECH AND SIGNAL PROCESSING (ICASSP)(2018)
摘要
The success of time-frequency (T-F) mask-based approaches is dependent on the accuracy of predicted mask given the noisy spectral features. The state-of-the-art methods in T-F masking-based enhancement employ Deep Neural Network (DNN) to predict mask. Recently, Generative Adversarial Networks (GAN) are gaining popularity instead of maximum likelihood (ML)-based optimization of deep learning architectures. In this paper, we propose to exploit GAN in TF masking-based enhancement framework. We present the viable strategy to use GAN in such application by modifying the existing approach. To achieve this, we use a method that learns the mask implicitly while predicting the clean TF representation. Moreover, we show the failure of vanilla GAN in predicting the accurate mask and propose a regularized objective function with the use of Mean Square Error (MSE) between predicted and target spectrum to overcome it. The objective evaluation of the proposed method shows the improvement in the accurate mask prediction, as against the state-of-the-art ML-based optimization techniques. The proposed system significantly improves over a recent GAN-based speech enhancement system in improving speech quality, while maintaining a better trade-off between less speech distortion and more effective removal of background interferences present in the noisy mixture.
更多查看译文
关键词
Task-dependent masking, speech enhancement, generative adversarial networks
AI 理解论文
溯源树
样例
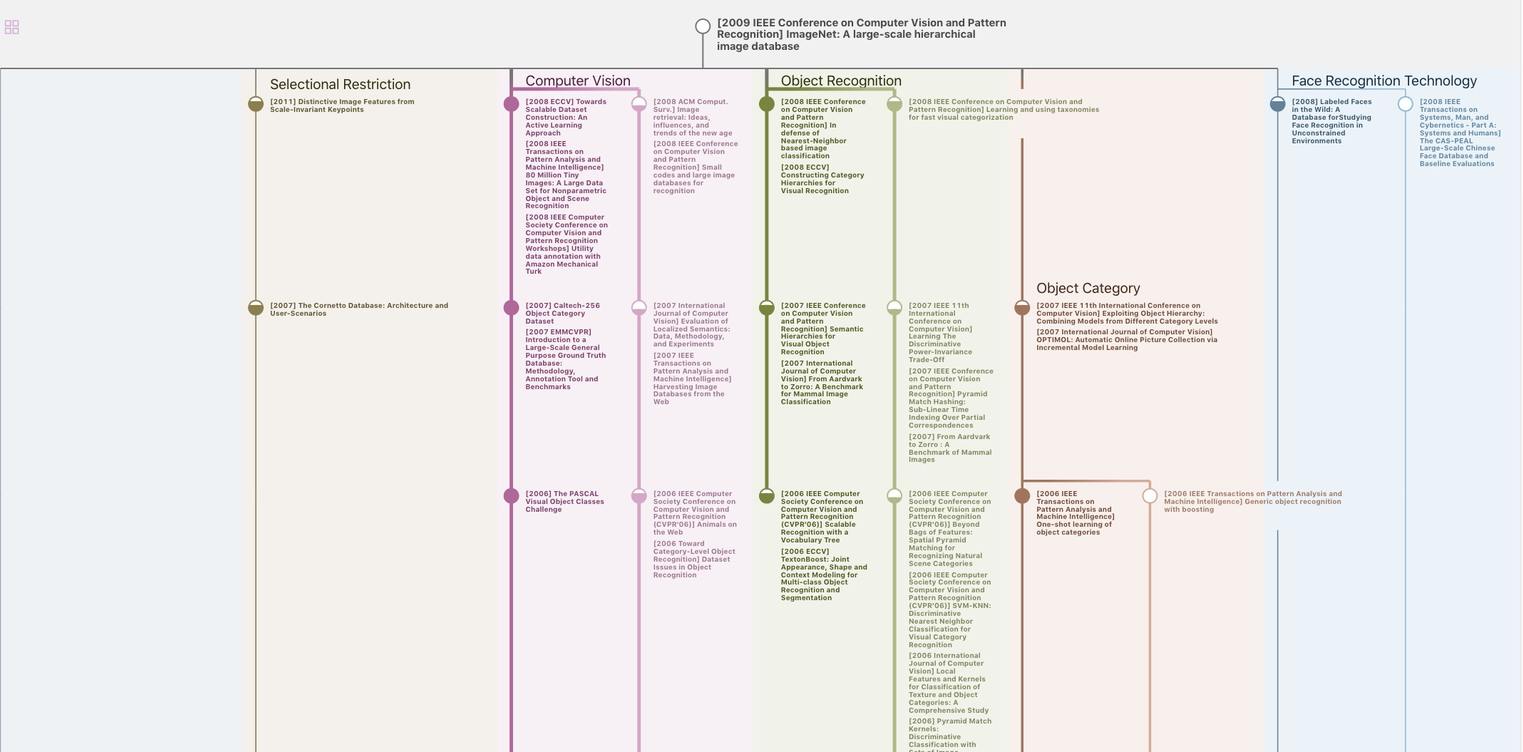
生成溯源树,研究论文发展脉络
Chat Paper
正在生成论文摘要