A Generative Adversarial Network Based Framework For Unsupervised Visual Surface Inspection
2018 IEEE INTERNATIONAL CONFERENCE ON ACOUSTICS, SPEECH AND SIGNAL PROCESSING (ICASSP)(2018)
摘要
Visual surface inspection is a challenging task due to the highly inconsistent appearance of the target surfaces and the abnormal regions. Most of the state-of-the-art methods are highly dependent on the labelled training samples, which are difficult to collect in practical industrial applications. To address this problem, we propose a generative adversarial network based framework for unsupervised surface inspection. The generative adversarial network is trained to generate the fake images analogous to the normal surface images. It implies that a well-trained GAN indeed learns a good representation of the normal surface images in a latent feature space. And consequently, the discriminator of GAN can serve as a naturally one-class classifier. We use the first three conventional layer of the discriminator as the feature extractor, whose response is sensitive to the abnormal regions. Particularly, a multi-scale fusion strategy is adopted to fuse the responses of the three convolution layers and thus improve the segmentation performance of abnormal detection. Various experimental results demonstrate the effectiveness of our proposed method.
更多查看译文
关键词
visual surface inspection, unsupervised learning, generative adversarial networks, multi-scale fusion
AI 理解论文
溯源树
样例
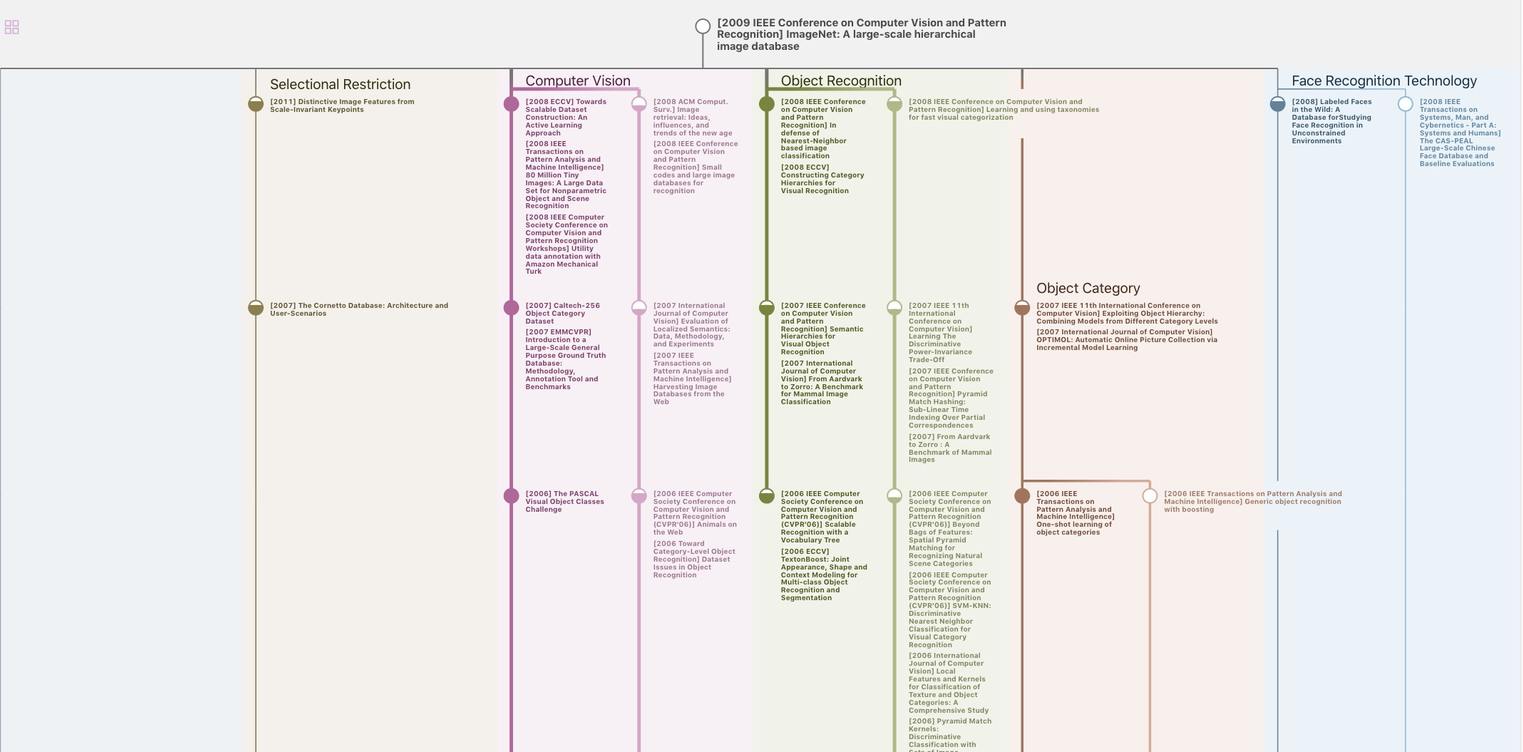
生成溯源树,研究论文发展脉络
Chat Paper
正在生成论文摘要