Unsupervised Domain Adaptation For Gender-Aware Plda Mixture Models
2018 IEEE INTERNATIONAL CONFERENCE ON ACOUSTICS, SPEECH AND SIGNAL PROCESSING (ICASSP)(2018)
摘要
Probabilistic linear discriminant analysis (PLDA) is a state-of-art back-end for i-vector based speaker verification. However, this back-end is still problematic when (1) the model is deployed to new environment (in-domain) that is very different from the training one (out-of-domain) and (2) there are insufficient labeled data from the new environment. To address these problems, this paper proposes using out-of-domain training data to pre-train a PLDA mixture model and applying the mixture model on the in-domain training data to compute a pairwise score matrix for spectral clustering. The hypothesized speaker labels produced by spectral clustering are then used for re-training the mixture model to fit the new environment. To refine the mixture model, the spectral clustering and re-training processes are repeated a number of times. To make the mixture model amenable to both genders, a deep neural network (DNN) is trained to produce gender posteriors given an i-vector. The gender posteriors then replace the posterior probabilities of the indicator variables in the PLDA mixture model. Evaluations based on NIST 2016 SRE suggest that at the end of the iterative re-training, the PLDA mixture model becomes fully adapted to the new domain. Results also show that the PLDA scores can be readily incorporated into spectral clustering, resulting in high quality speaker clusters that could not be possibly achieved by agglomerative hierarchical clustering.
更多查看译文
关键词
I-vectors, DNN-driven mixture of PLDA, spectral clustering, domain adaptation, speaker verification
AI 理解论文
溯源树
样例
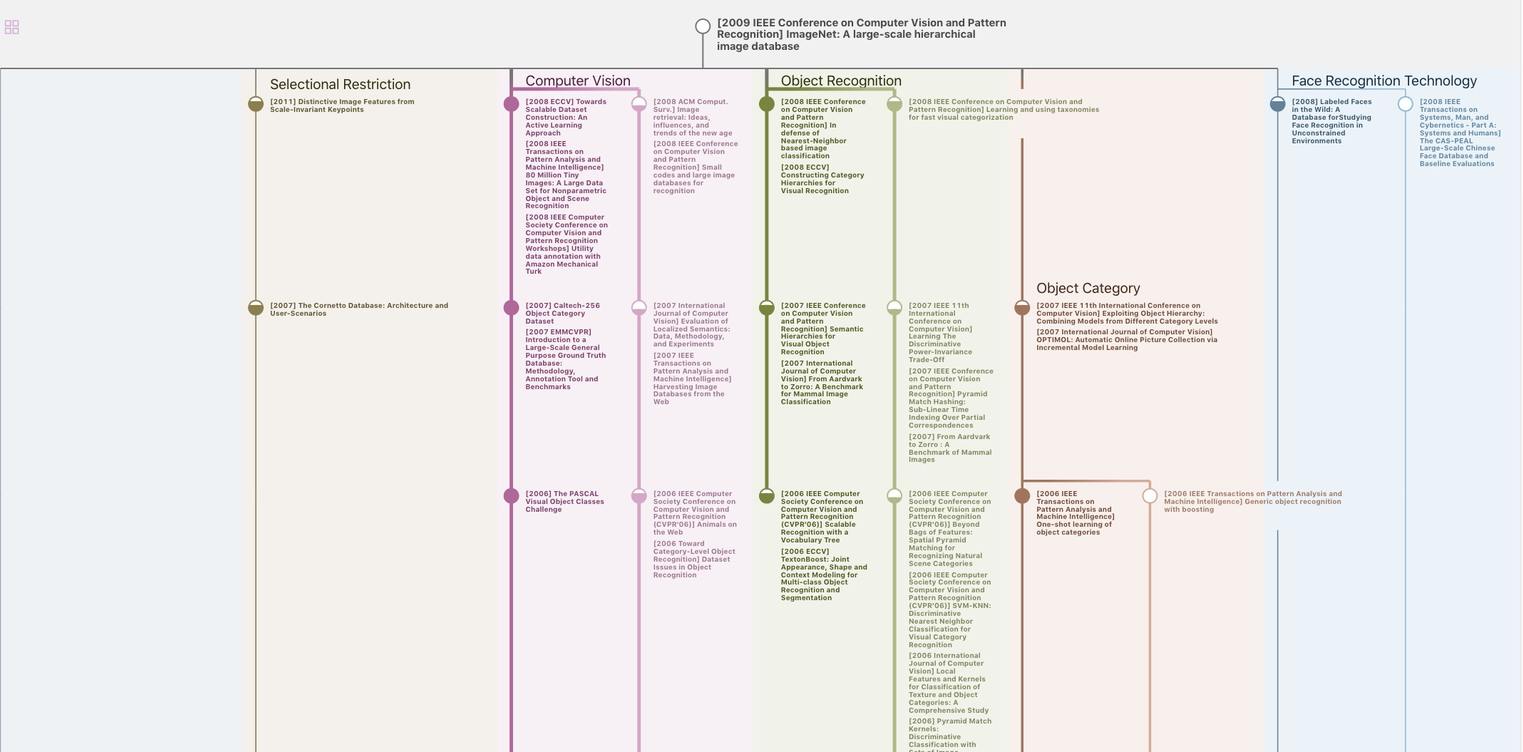
生成溯源树,研究论文发展脉络
Chat Paper
正在生成论文摘要