Super Wide Regression Network For Unsupervised Cross-Database Facial Expression Recognition
2018 IEEE INTERNATIONAL CONFERENCE ON ACOUSTICS, SPEECH AND SIGNAL PROCESSING (ICASSP)(2018)
摘要
Unsupervised cross-database facial expression recognition (FER) is a challenging problem, in which the training and testing samples belong to different facial expression databases. For this reason, the training (source) and testing (target) facial expression samples would have different feature distributions and hence the performance of lots of existing FER methods may decrease. To solve this problem, in this paper we propose a novel super wide regression network (SWiRN) model, which serves as the regression parameter to bridge the original feature space and the label space and herein in each layer the maximum mean discrepancy (MMD) criterion is used to enforce the source and target facial expression samples to share the same or similar feature distributions. Consequently, the learned SWiRN is able to predict the expression categories of the target samples although we have no access to any label information of target samples. We conduct extensive cross-database FER experiments on CK+, eNTERFACE, and Oulu-CASIA VIS facial expression databases to evaluate the proposed SWiRN. Experimental results show that our SWiRN model achieves more promising performance than recent proposed cross-database emotion recognition methods.
更多查看译文
关键词
Cross-database facial expression recognition, transfer learning, domain adaptation, super wide network
AI 理解论文
溯源树
样例
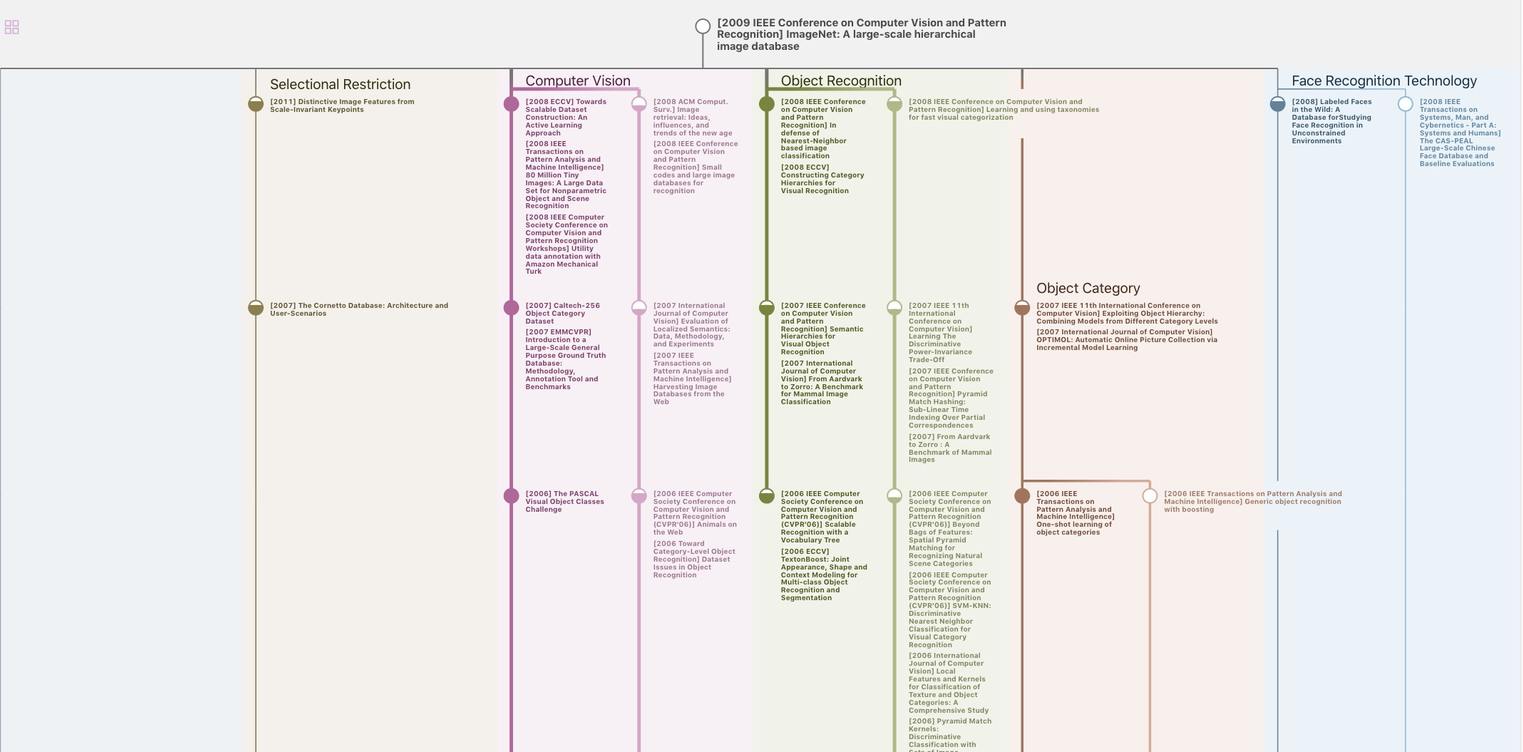
生成溯源树,研究论文发展脉络
Chat Paper
正在生成论文摘要