Efficient Worker Assignment In Crowdsourced Data Labeling Using Graph Signal Processing
2018 IEEE INTERNATIONAL CONFERENCE ON ACOUSTICS, SPEECH AND SIGNAL PROCESSING (ICASSP)(2018)
摘要
The first step in solving a classification problem is to collect and label a sufficient amount of training data. Given the time and cost associated to data labeling, crowdsourcing systems (e.g., Amazon Mechanical Turk) are often used. However, one of the key disadvantages of crowdsourcing systems is the presence of spammers or workers who are not as skilled or careful, thus leading to many false labels being assigned. This paper addresses this problem by proposing a novel algorithm based on graph signal sampling theory, which optimally assigns data to different workers for labeling by taking into account the expected quality of labeling provided by each worker. Our simulation of the labeling process using these schemes shows that the classification error can be reduced significantly with respect to a random assignment of workers.
更多查看译文
关键词
Crowdsourcing, resource allocation, labeling, Graph Signal Processing
AI 理解论文
溯源树
样例
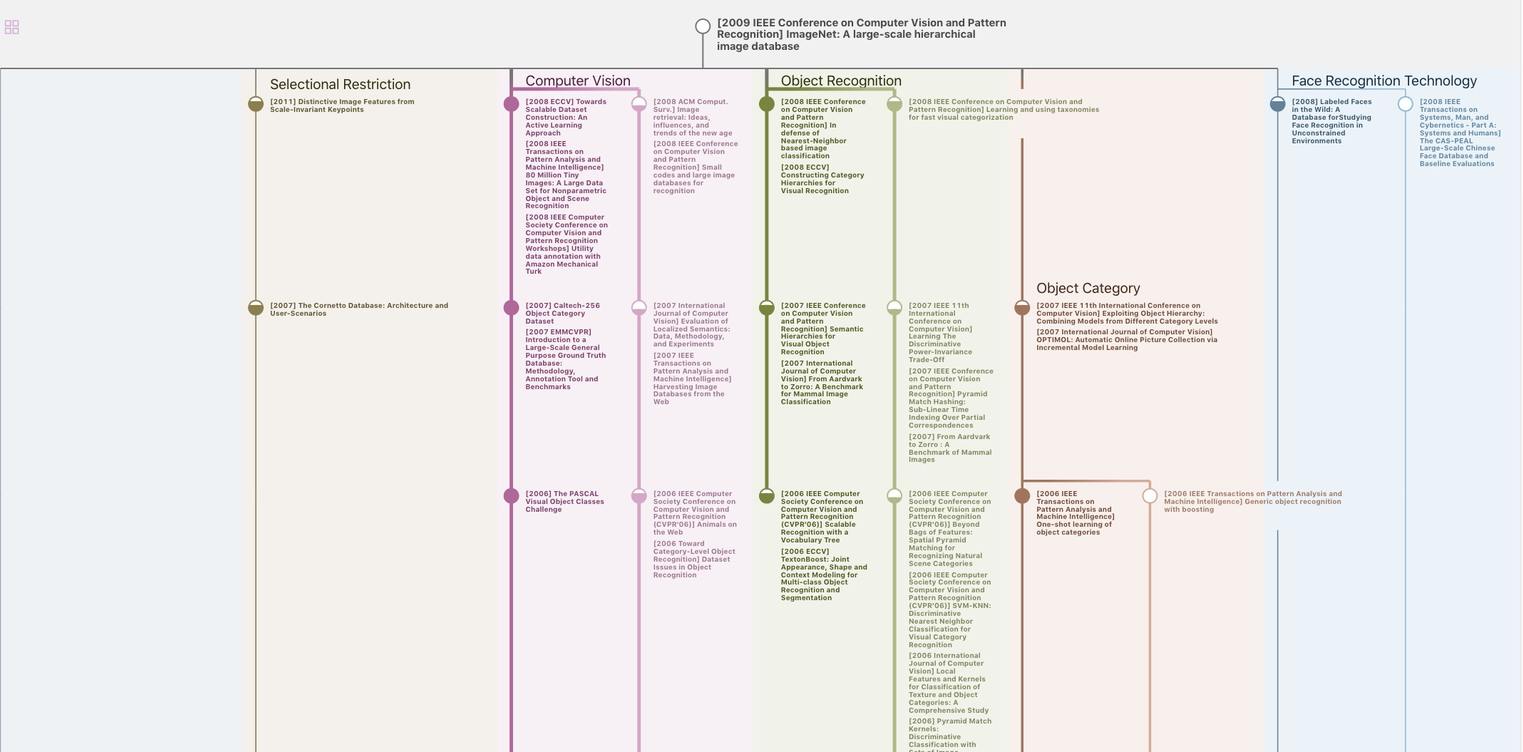
生成溯源树,研究论文发展脉络
Chat Paper
正在生成论文摘要