Robust Spoken Language Understanding With Unsupervised Asr-Error Adaptation
2018 IEEE INTERNATIONAL CONFERENCE ON ACOUSTICS, SPEECH AND SIGNAL PROCESSING (ICASSP)(2018)
摘要
Robustness to errors produced by automatic speech recognition (ASR) is essential for Spoken Language Understanding (SLU). Traditional robust SLU typically needs ASR hypotheses with semantic annotations for training. However, semantic annotation is very expensive, and the corresponding ASR system may change frequently. Here, we propose a novel unsupervised ASR-error adaptation method, obviating the need of annotated ASR hypotheses. It only requires semantically annotated transcripts for the slot-tagging task and the transcripts paired with hypotheses for an input sentence reconstruction task. In this method, feature encoders which share part of the parameters are exploited to enforce the tasks in a similar feature space. Therefore, the transcript side slot-tagging model can be transferred to ASR hypotheses side easily. Experiments show that the proposed approach can yield significant improvement over strong baselines, and achieve performance very close to the oracle system.
更多查看译文
关键词
Spoken Language understanding, ASR-error robustness, adversarial adaptation
AI 理解论文
溯源树
样例
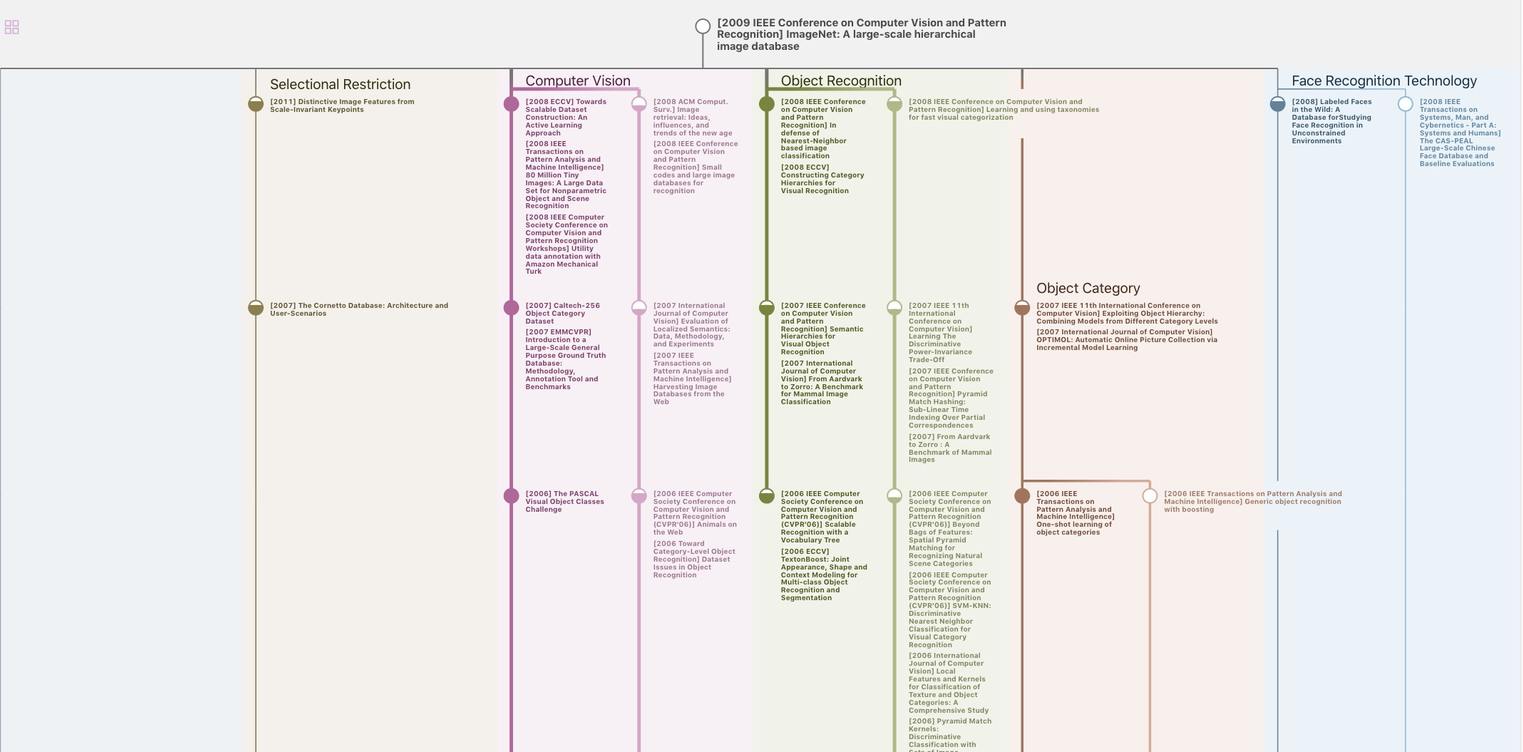
生成溯源树,研究论文发展脉络
Chat Paper
正在生成论文摘要