Joint License Plate Super-Resolution And Recognition In One Multi-Task Gan Framework
2018 IEEE INTERNATIONAL CONFERENCE ON ACOUSTICS, SPEECH AND SIGNAL PROCESSING (ICASSP)(2018)
摘要
License plate recognition (LPR) plays an important role in intelligent transport systems. The existed LPR systems are mostly based on hand-crafted methods for detection, segmentation, and recognition, which cannot accurately recognize the license plate in unconstrained surveillance environments. In this paper, we propose a Multi-Task Generative Adversarial Network (MTGAN) based LPR system, which combines the license plate super-resolution and recognition in one end-toend framework. In the proposed MTGAN, we design a Fully Connected Network (FCN) as generative network (GN), which can combine knowledge from data distribution and domain prior knowledge of license plate to generate the spatial corresponding and high-resolution plate images in the synthesis pipeline. More important, a multi-task discriminative network is designed in MTGAN to combine the super-resolution and recognition in an adversarial manner to enhance each other. The experiments on the built real-world license plate dataset show that the proposed LPR system can generate high-resolution license plates as well as recognize them with higher accuracy than state-of-the-art LPR systems.
更多查看译文
关键词
Generative Adversarial Network, Super-Resolution, License Plate Recognition, Multi-Task
AI 理解论文
溯源树
样例
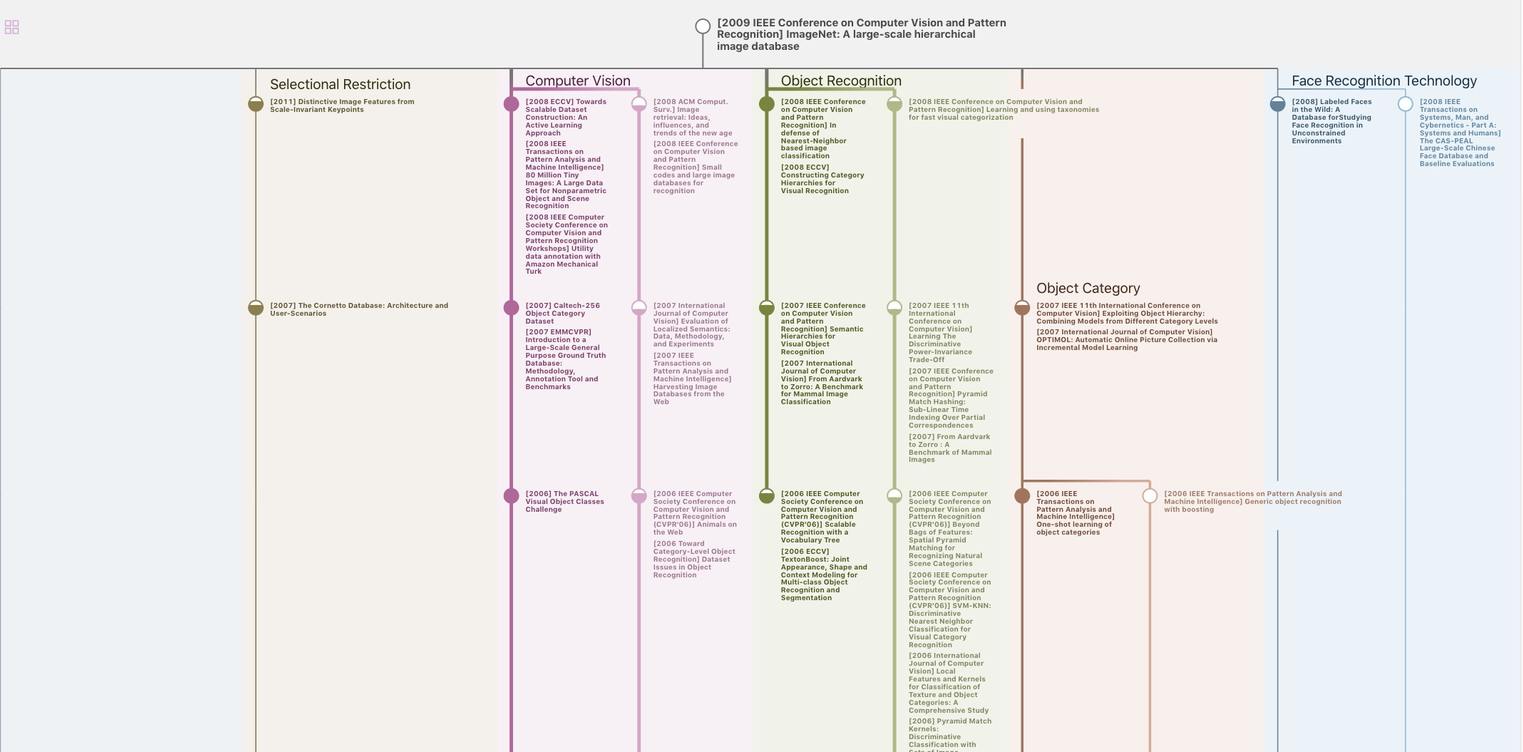
生成溯源树,研究论文发展脉络
Chat Paper
正在生成论文摘要