Random Walks With Restarts For Graph-Based Classification: Teleportation Tuning And Sampling Design
2018 IEEE INTERNATIONAL CONFERENCE ON ACOUSTICS, SPEECH AND SIGNAL PROCESSING (ICASSP)(2018)
摘要
The present work introduces methods for sampling and inference for the purpose of semi-supervised classification over the nodes of a graph. The graph may be given or constructed using similarity measures among nodal features. Leveraging the graph for classification builds on the premise that relation among nodes can be modeled via stationary distributions of a certain class of random walks. The proposed classifier builds on existing scalable random-walk-based methods and improves accuracy and robustness by automatically adjusting a set of parameters to the graph and label distribution at hand. Furthermore, a sampling strategy tailored to random-walk-based classifiers is introduced. Numerical tests on benchmark synthetic and real labeled graphs demonstrate the performance of the proposed sampling and inference methods in terms of classification accuracy.
更多查看译文
关键词
Random Walks, Sampling on Graphs, PageRank, Semi-Supervised Learning, Seed Set Expansion
AI 理解论文
溯源树
样例
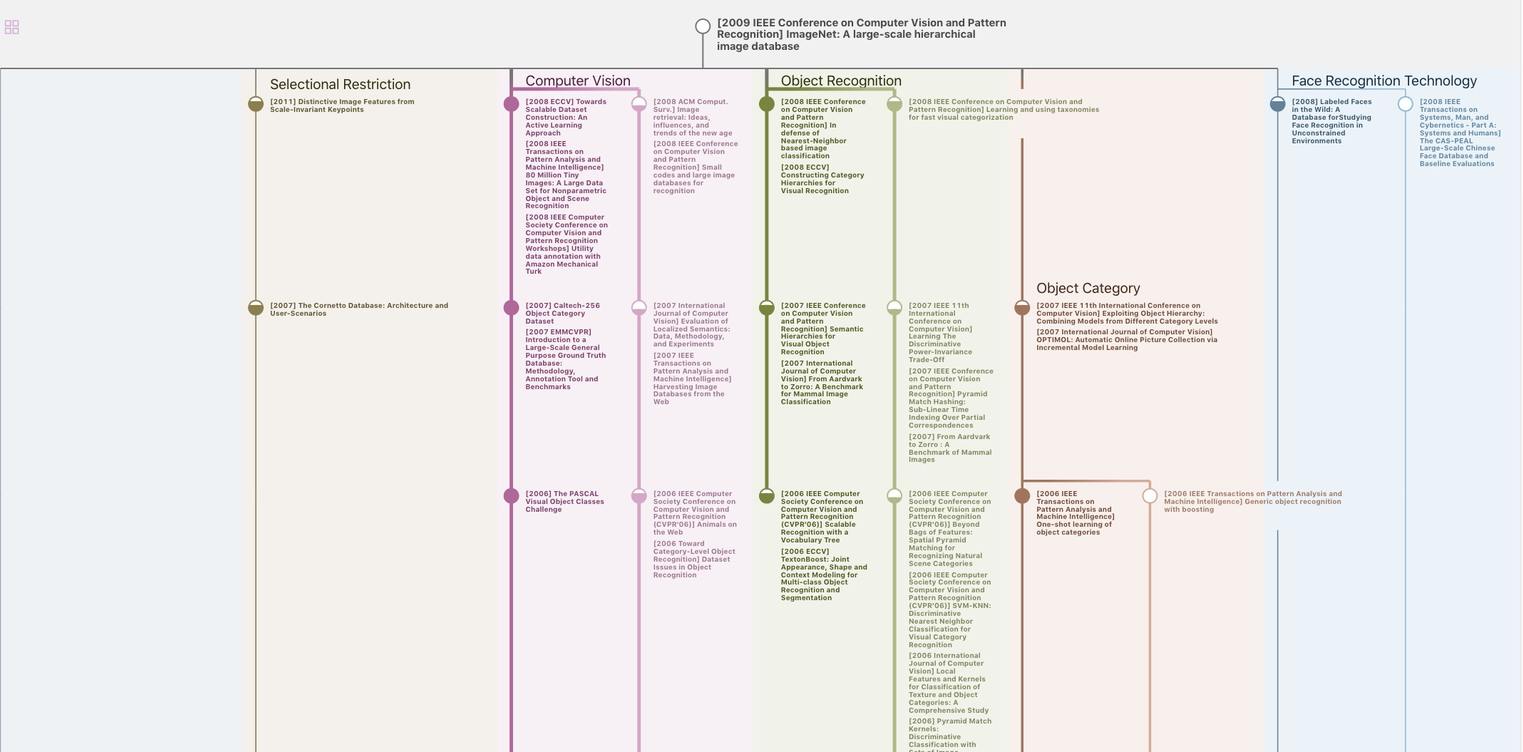
生成溯源树,研究论文发展脉络
Chat Paper
正在生成论文摘要