Scalable Energy Disaggregation Via Successive Submodular Approximation
2018 IEEE INTERNATIONAL CONFERENCE ON ACOUSTICS, SPEECH AND SIGNAL PROCESSING (ICASSP)(2018)
摘要
Energy disaggregation is the task of decomposing the aggregated power consumption readings of a household into its constituent parts. In this paper, we propose a supervised, non-parametric framework for energy disaggregation. We demonstrate that the problem is equivalent to maximizing a set-function subject to combinatorial constraints, which is NP-hard in its general form. A simple polynomial-time successive approximation algorithm which exploits submodularity per set-block to iteratively maximize a sequence of global lower bounds of the objective function is proposed for obtaining approximate solutions. Experiments on real data indicate the superior disaggregation performance and scalability of our approach over a state-of-the-art parametric Factorial Hidden Markov Model based framework employing convex relaxation.
更多查看译文
关键词
scalable energy disaggregation,aggregated power consumption readings,combinatorial constraints,parametric factorial hidden Markov model based framework,submodular approximation,polynomial-time successive approximation algorithm
AI 理解论文
溯源树
样例
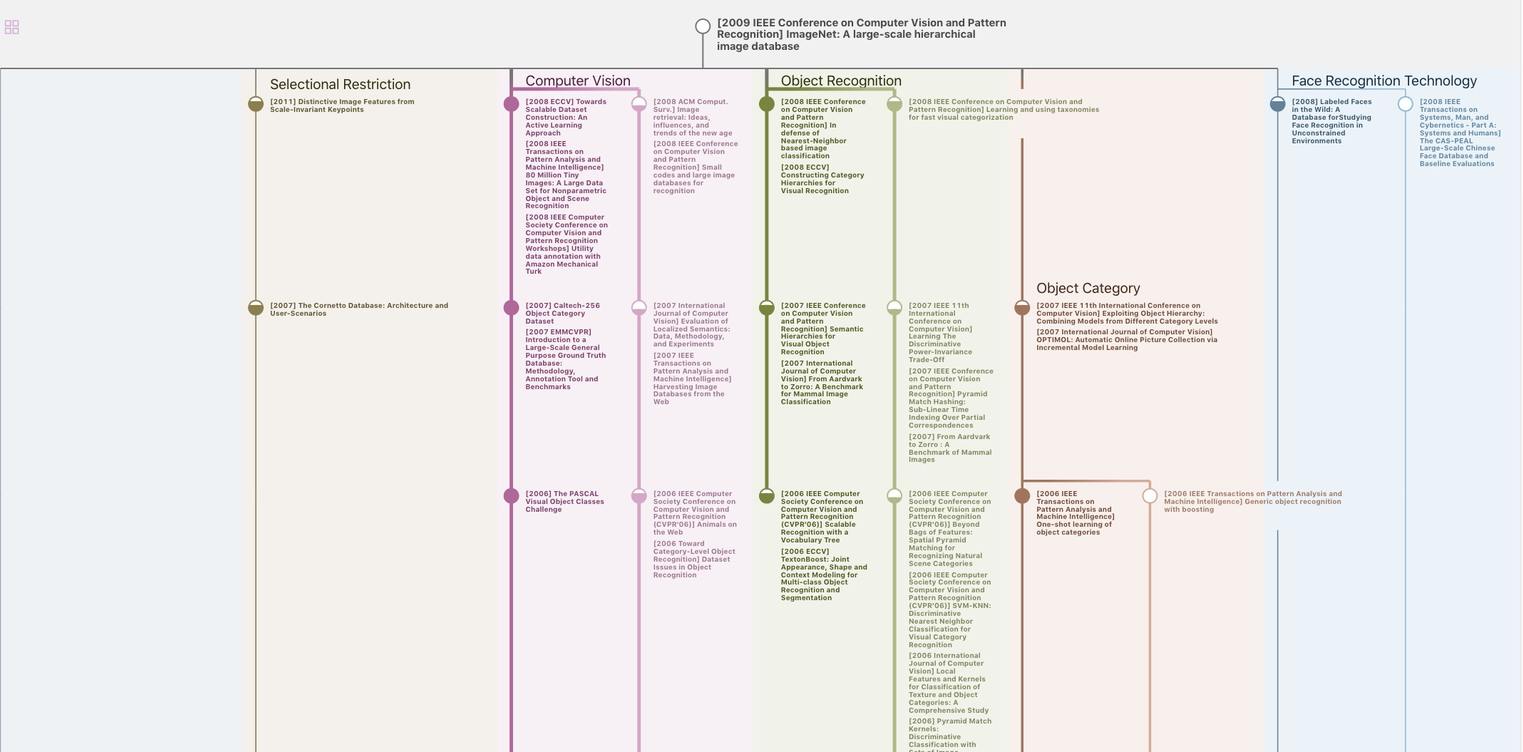
生成溯源树,研究论文发展脉络
Chat Paper
正在生成论文摘要