Improving Multichannel Speech Recognition With Generalized Cross Correlation Inputs And Multitask Learning
2018 IEEE INTERNATIONAL CONFERENCE ON ACOUSTICS, SPEECH AND SIGNAL PROCESSING (ICASSP)(2018)
摘要
Acoustic signals from microphone arrays are used to improve performance in distant speech recognition due to the availability of spatial information. And multichannel automatic speech recognition (ASR) systems often separate speech enhancement module from acoustic modeling, which may be not optimal for improving recognition accuracy. In this work, we propose to improve multichannel speech recognition by supplying the generalized cross correlation (GCC) between microphones, which encodes spatial information, as input features to a long short-term memory (LSTM) acoustic model in parallel with the regular acoustic features. Moreover, multitask learning architecture is incorporated and shows its ability to improve the robustness of the model. We performed experiments on the AMI and ICSI meeting corpora, with results indicating that the proposed model outperforms the model trained directly on the concatenation of multiple microphone outputs and the model trained on a beam-formed channel.
更多查看译文
关键词
speech recognition, microphone array, acoustic model, generalized cross correlation, multitask learning
AI 理解论文
溯源树
样例
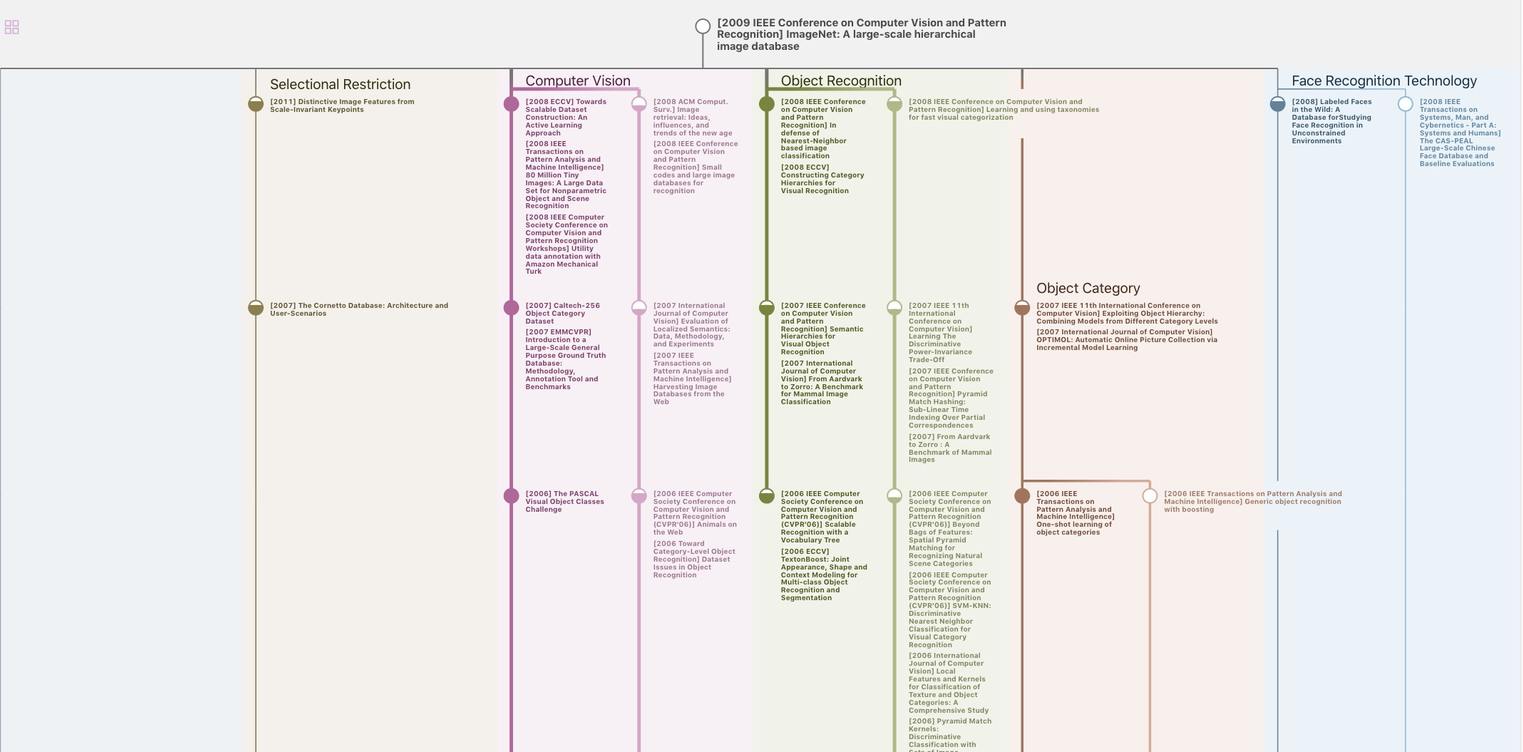
生成溯源树,研究论文发展脉络
Chat Paper
正在生成论文摘要