Large-Scale Regularized Sumcor Gcca Via Penalty-Dual Decomposition
2018 IEEE INTERNATIONAL CONFERENCE ON ACOUSTICS, SPEECH AND SIGNAL PROCESSING (ICASSP)(2018)
摘要
The sum-of-correlations (SUMCOR) generalized canonical correlation analysis (GCCA) aims at producing low-dimensional representations of multiview data via enforcing pairwise similarity of the reduced-dimension views. SUMCOR has been applied to a large variety of applications including blind separation, multilingual word embedding, and cross-modality retrieval. Despite the NP-hardness of SUMCOR, recent work has proposed effective algorithms for handling it at very large scale. However, the existing scalable algorithms are not easy to extend to incorporate structural regularization and prior information - which are critical for real-world applications where outliers and modeling mismatches are present. In this work, we propose a new computational framework for large-scale SUMCOR GCCA. The algorithm can easily incorporate a suite of structural regularizers which are frequently used in data analytics, has lightweight updates and low memory complexity, and can be easily implemented in a parallel fashion. The proposed algorithm is also guaranteed to converge to a Karush-Kuhn-Tucker (KKT) point of the regularized SUMCOR problem. Carefully designed simulations are employed to demonstrate the effectiveness of the proposed algorithm.
更多查看译文
关键词
Generalized canonical correlation analysis, SUMCOR, multi-view analysis, regularization, feature extraction
AI 理解论文
溯源树
样例
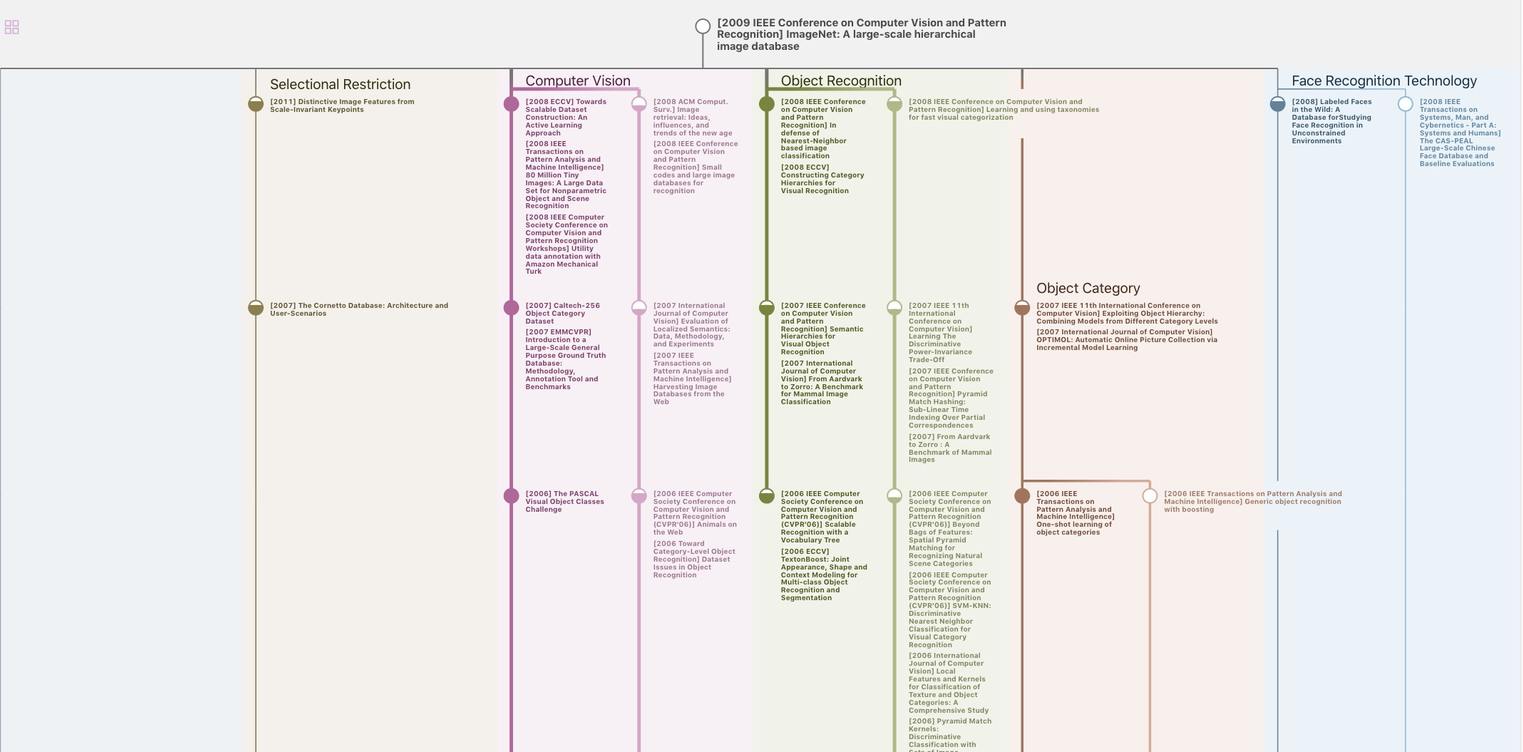
生成溯源树,研究论文发展脉络
Chat Paper
正在生成论文摘要