Fast and Accurate Estimation of Quality of Results in High-Level Synthesis with Machine Learning
2018 IEEE 26th Annual International Symposium on Field-Programmable Custom Computing Machines (FCCM)(2018)
摘要
While high-level synthesis (HLS) offers sophisticated techniques to optimize designs for area and performance, HLS-estimated resource usage and timing often deviate significantly from actual quality of results (QoR) achieved by FPGA-targeted designs. Inaccurate HLS estimates prevent designers from performing meaningful design space exploration without resorting to the time-consuming downstream implementation process. To address this challenge, we first build a large collection of C-to-FPGA results from a diverse set of realistic HLS applications and identify relevant features from HLS reports for estimating post-implementation metrics. We then leverage these features and data to train and compare a number of promising machine learning models to effectively and efficiently bridge the accuracy gap. Experiments demonstrate that our proposed approach is able to dramatically reduce the estimation errors for different families of FPGA devices. By extracting domain-specific insights from our experiments, we explore the implications of our models and predictive influence of various features for enabling fast and accurate QoR estimation in HLS. We have released our dataset to springboard future efforts in this area.
更多查看译文
关键词
high level synthesis,field programmable gate array,machine learning,quality of results
AI 理解论文
溯源树
样例
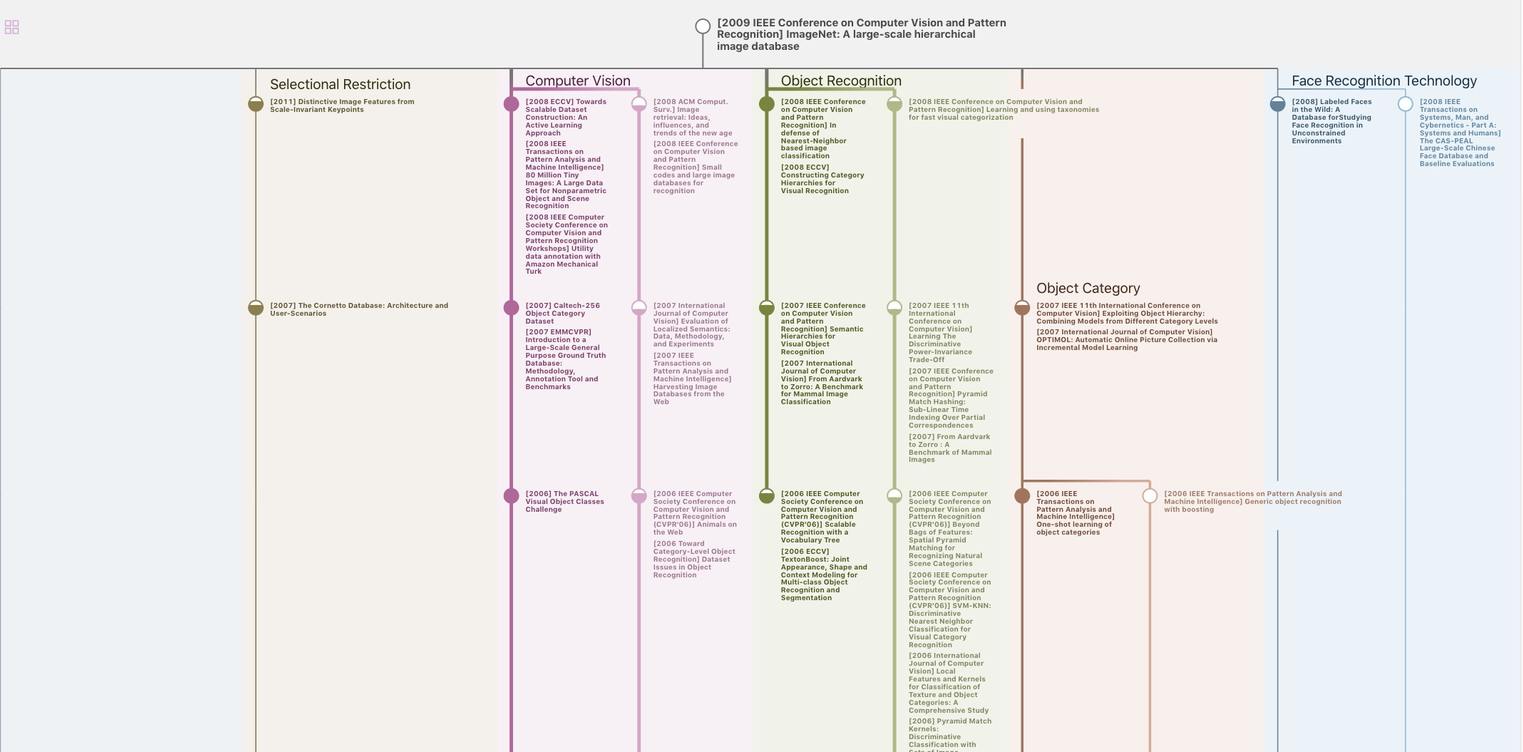
生成溯源树,研究论文发展脉络
Chat Paper
正在生成论文摘要