Denoising And Raw-Waveform Networks For Weakly-Supervised Gender Identification On Noisy Speech
19TH ANNUAL CONFERENCE OF THE INTERNATIONAL SPEECH COMMUNICATION ASSOCIATION (INTERSPEECH 2018), VOLS 1-6: SPEECH RESEARCH FOR EMERGING MARKETS IN MULTILINGUAL SOCIETIES(2018)
摘要
This paper presents a raw-waveform neural network and uses it along with a denoising network for clustering in weakly supervised learning scenarios under extreme noise conditions. Specifically, we consider language independent Automatic Gender Recognition (AGR) on a set of varied noise conditions and Signal to Noise Ratios (SNRs). We formulate the denoising problem as a source separation task and train the system using a discriminative criterion in order to enhance output SNRs. A denoising Recurrent Neural Network (RNN) is first trained on a small subset (roughly one-fifth) of the data for learning a speech specific mask. The denoised speech signal is then directly fed as input to a raw-waveform convolutional neural network (CNN) trained with denoised speech. We evaluate the standalone performance of denoiser in terms of various signal-to-noise measures and discuss its contribution towards robust AGR. An absolute improvement of 11.06% and 13.33% is achieved by the combined pipeline over the i-vector SVM baseline system for 0 dB and -5 dB SNR conditions, respectively. We further analyse the information captured by the first CNN layer in both noisy and denoised speech.
更多查看译文
关键词
speech enhancement, Automatic Gender Recognition, convolutional neural network, recurrent neural network
AI 理解论文
溯源树
样例
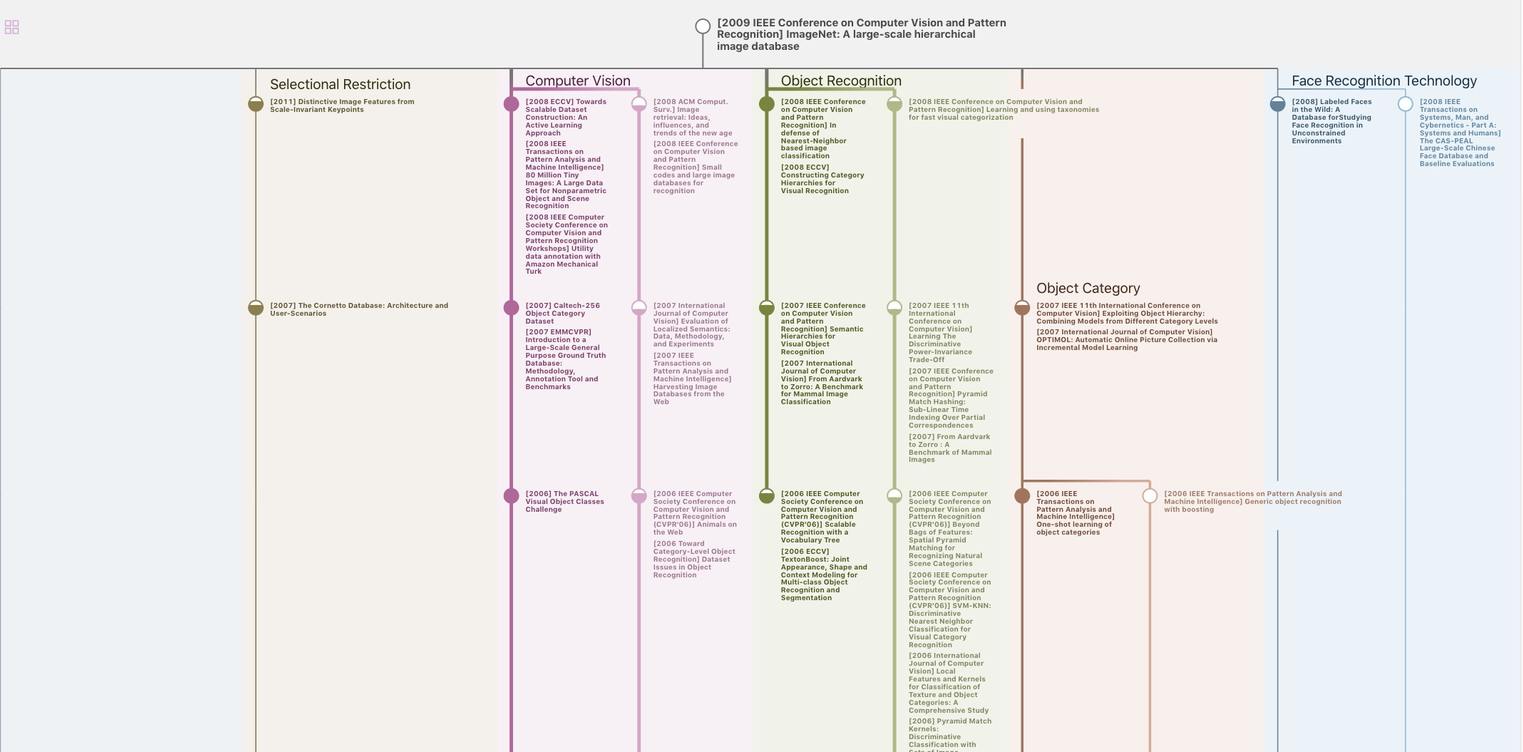
生成溯源树,研究论文发展脉络
Chat Paper
正在生成论文摘要