Cross-Modal Retrieval with Discriminative Dual-Path CNN.
ADVANCES IN MULTIMEDIA INFORMATION PROCESSING, PT III(2018)
摘要
Cross-modal retrieval aims at searching semantically similar examples in one modality by using a query from another modality. Its typical applications including image-based text retrieval (IBTR) and text-based image retrieval (TBIR). Due to the rapid growth of multimodal data and the success of deep learning, cross-modal retrieval has received increasing attention and achieved significant progress in recent years. Dual-path CNN is a novel framework in this domain, which yields competitive performance by utilizing instance loss and inter-modal loss. However, it is still less discriminative in modeling the intra-modal relationship, which is also important in bridging a more discriminative cross-modal embedding network. To this end, we propose to incorporate an additional intra-modal loss into the framework to remedy this problem by preserving the intra-modal structure. Further, we develop a novel batch flexible sampling approach to train the entire network effectively and efficiently. Our approach, named Discriminative Dual-Path CNN (DDPC), achieves the state-of-the-art results on the MS-COCO dataset, improving IBTR by 4.9% and TBIR by 5.9% based on Recall@1 on the 5K test set.
更多查看译文
关键词
Cross-modal retrieval,Convolutional neural network,Intra-modal,Inter-modal,Embedding space
AI 理解论文
溯源树
样例
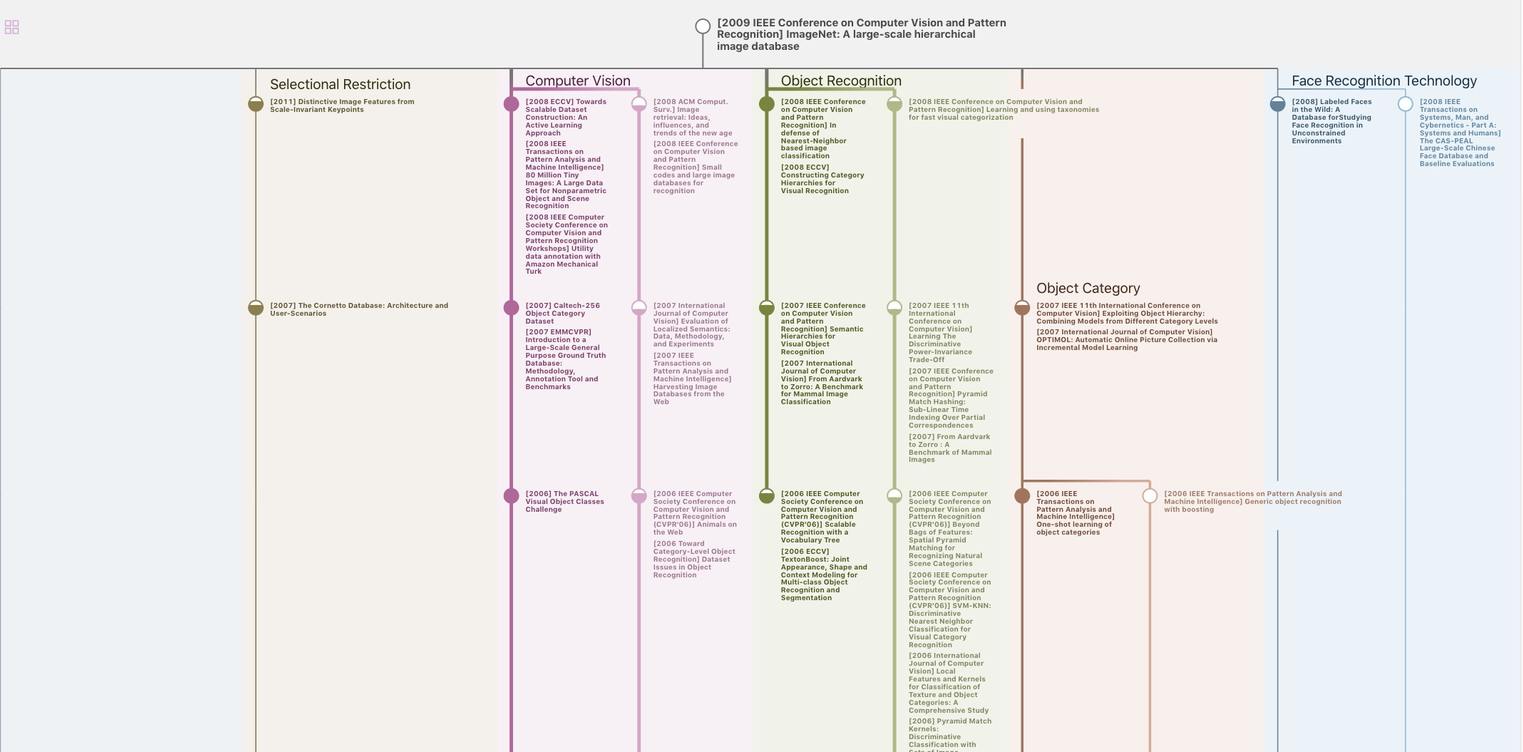
生成溯源树,研究论文发展脉络
Chat Paper
正在生成论文摘要