Self-supervised GAN for Image Generation by Correlating Image Channels.
ADVANCES IN MULTIMEDIA INFORMATION PROCESSING - PCM 2018, PT II(2018)
摘要
Current most GAN-based methods directly generate all channels of a color image as a whole, while digging self-supervised information from the correlation between image channels for improving image generation has not been investigated. In this paper, we consider that a color image could be split into multiple sets of channels in terms of channels' semantic, and these sets of channels are closely related rather than completely independent. By leveraging this characteristic of color images, we introduce self-supervised learning into the GAN framework, and propose a generative model called Self-supervised GAN. Specifically, we explicitly decompose the generation process as follows: (1) generate image channels, (2) correlate image channels, (3) concatenate image channels into the whole image. Based on these operations, we not only perform a basic adversarial learning task for generating images, but also construct an auxiliary self-supervised learning task for further regularizing generation procedures. Experimental results demonstrate that the proposed method can improve image generation compared with representative methods and possess capabilities of image colorization and image texturization.
更多查看译文
关键词
Image generation,GAN,Self-supervised learning
AI 理解论文
溯源树
样例
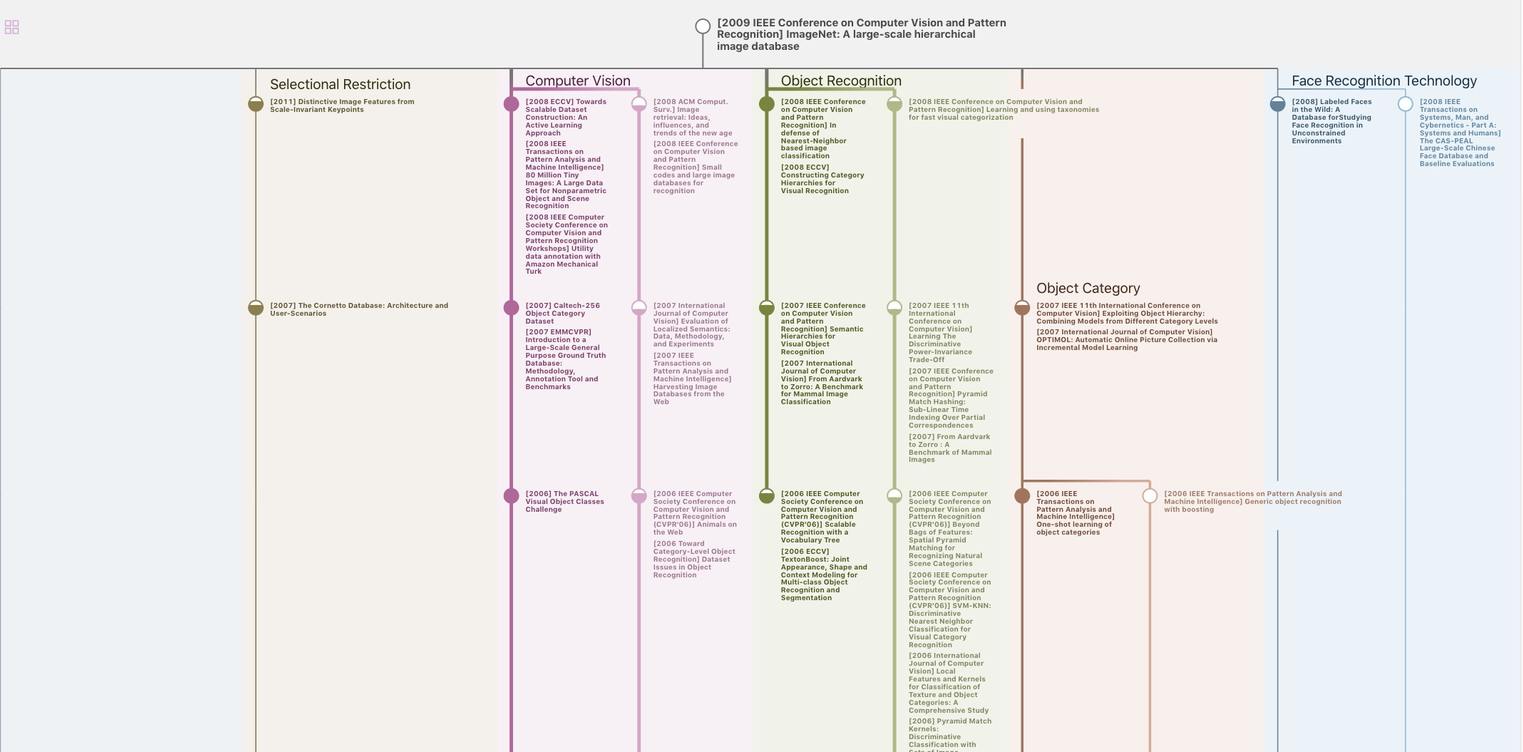
生成溯源树,研究论文发展脉络
Chat Paper
正在生成论文摘要