Pedestrian Attributes Recognition in Surveillance Scenarios with Hierarchical Multi-task CNN Models.
ADVANCES IN MULTIMEDIA INFORMATION PROCESSING - PCM 2018, PT II(2018)
摘要
Pedestrian attributes recognition is a very important problem in video surveillance and video forensics. Traditional methods assume the pedestrian attributes are independent and design handcraft features for each one. In this paper, we propose a joint hierarchical multi-task learning algorithm to learn the relationships among attributes for better recognizing the pedestrian attributes in still images using convolutional neural networks (CNN). We divide the attributes into local and global ones according to spatial and semantic relations, and then consider learning semantic attributes through a hierarchical multi-task CNN model where each CNN in the first layer will predict each group of such local attributes and CNN in the second layer will predict the global attributes. Our multi-task learning framework allows each CNN model to simultaneously share visual knowledge among different groups of attribute categories. Extensive experiments are conducted on two popular and challenging benchmarks in surveillance scenarios, namely, the PETA and RAP pedestrian attributes datasets. On both benchmarks, our framework achieves superior results over the state-of-the-art methods by 88.2% on PETA and 83.25% on RAP, respectively.
更多查看译文
关键词
Attributes recognition,CNN,Multi-task learning
AI 理解论文
溯源树
样例
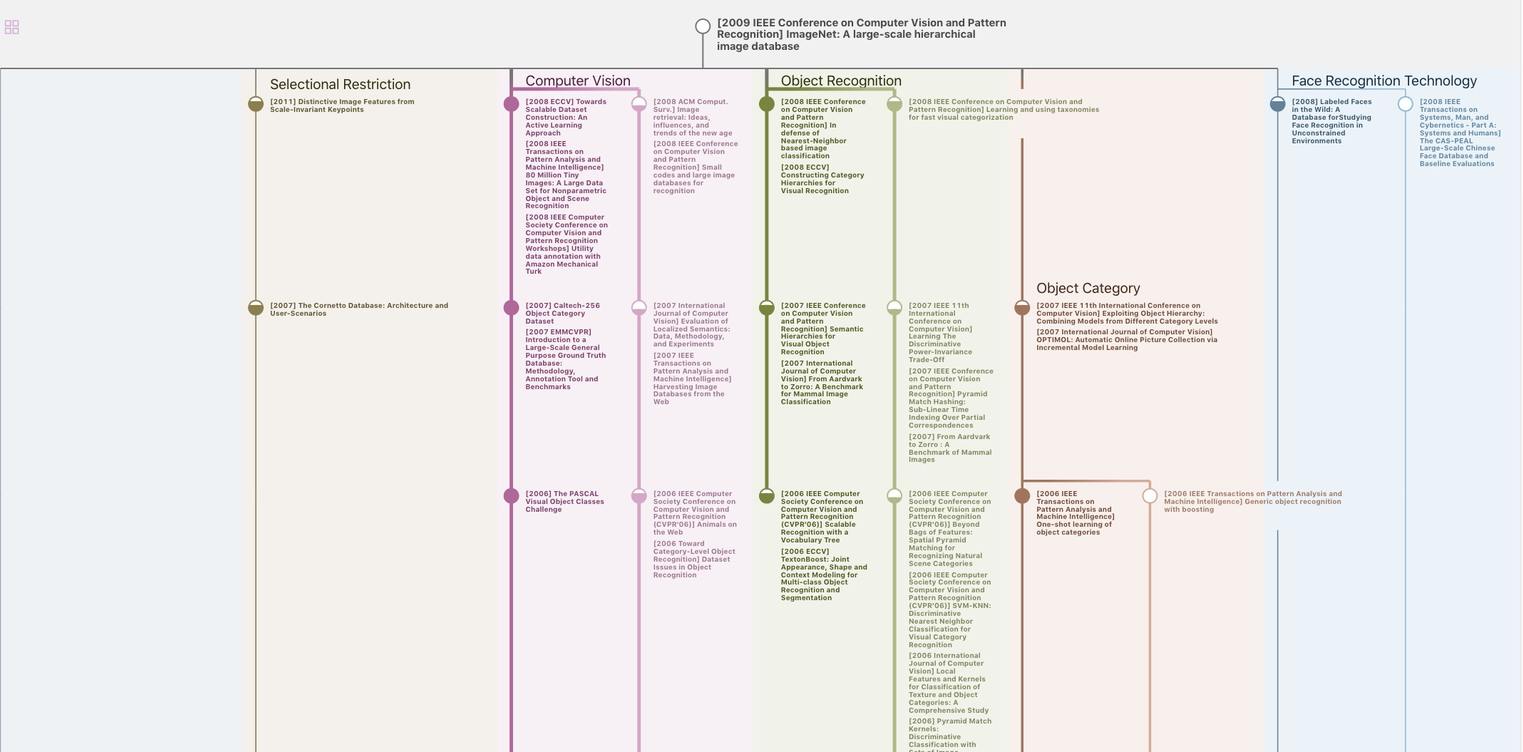
生成溯源树,研究论文发展脉络
Chat Paper
正在生成论文摘要