Random Angular Projection for Fast Nearest Subspace Search.
ADVANCES IN MULTIMEDIA INFORMATION PROCESSING, PT I(2018)
摘要
Finding the nearest subspace is a fundamental problem in many tasks such as recognition, retrieval and optimization. This hard topic has been seldom touched in the literature, except a very few studies that address it using the locality sensitive hashing for subspaces. The existing solutions severely suffer from poor scaling with expensive computational cost or unsatisfying accuracy, when the subspaces originally distribute with arbitrary dimensions. To address these problems, this paper proposes a new and efficient family of locality sensitive hash for linear subspaces of arbitrary dimension. It preserves the angular distances among subspaces by randomly projecting their orthonormal basis and further encoding them with binary codes. The proposed method enjoys fast computation and meanwhile keeps the strong collision probability. Moreover, it owns flexibility to easily balance the performance between the accuracy and efficiency. The experimental results in the tasks of face recognition and video de-duplication demonstrate that the proposed method significantly outperforms the state-of-the-art vector and subspace hashing methods, in terms of both accuracy and efficiency (up to 16x speedup).
更多查看译文
关键词
Nearest subspace search,Locality sensitive hash,Linear subspace,Subspace hashing
AI 理解论文
溯源树
样例
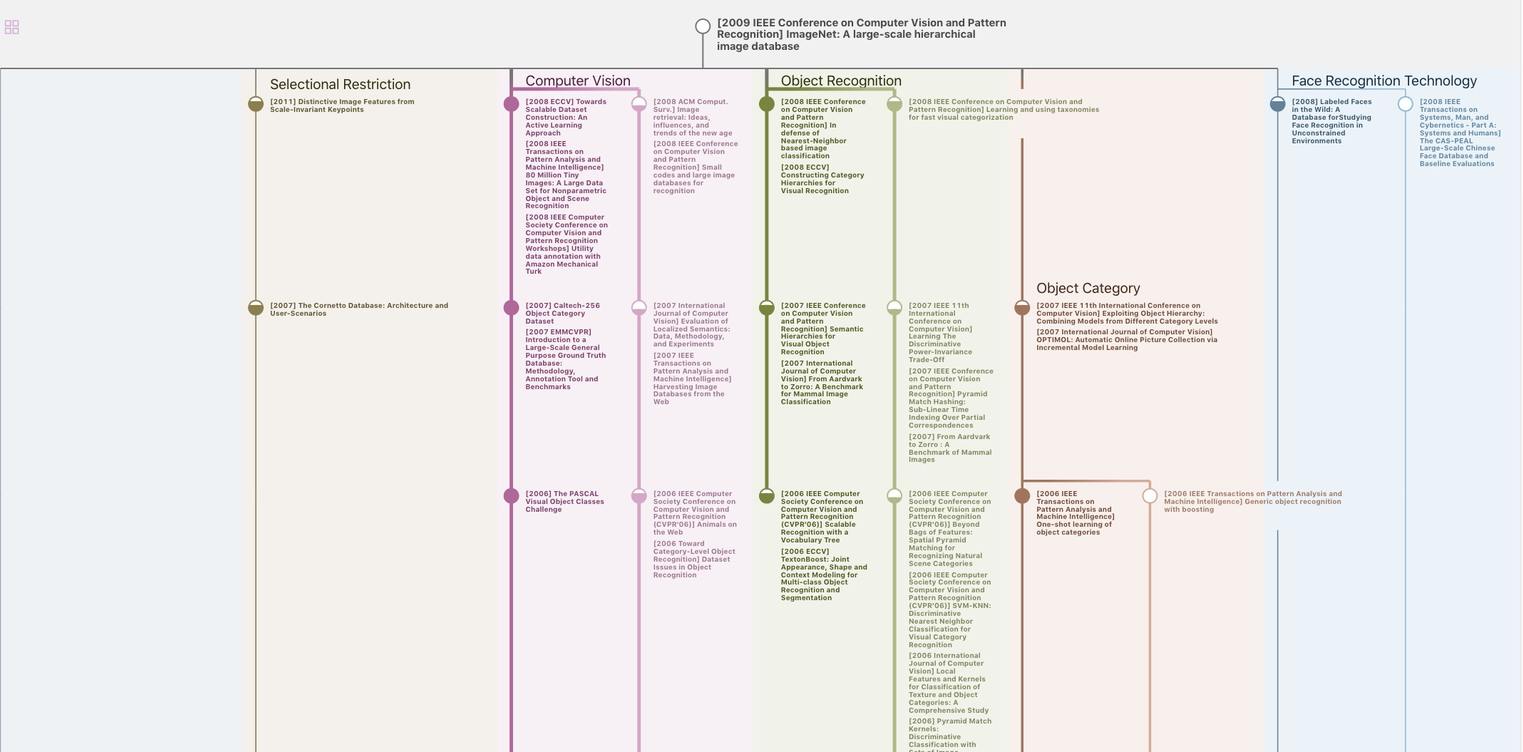
生成溯源树,研究论文发展脉络
Chat Paper
正在生成论文摘要