Mark Yourself: Road Marking Segmentation Via Weakly-Supervised Annotations From Multimodal Data
2018 IEEE INTERNATIONAL CONFERENCE ON ROBOTICS AND AUTOMATION (ICRA)(2018)
摘要
This paper presents a weakly-supervised learning system for real-time road marking detection using images of complex urban environments obtained from a monocular camera. We avoid expensive manual labelling by exploiting additional sensor modalities to generate large quantities of annotated images in a weakly-supervised way, which are then used to train a deep semantic segmentation network. At run time, the road markings in the scene are detected in real time in a variety of traffic situations and under different lighting and weather conditions without relying on any preprocessing steps or predefined models. We achieve reliable qualitative performance on the Oxford RobotCar dataset, and demonstrate quantitatively on the CamVid dataset that exploiting these annotations significantly reduces the required labelling effort and improves performance.
更多查看译文
关键词
road marking segmentation,weakly-supervised annotations,multimodal data,weakly-supervised learning system,complex urban environments,monocular camera,expensive manual labelling,annotated images,deep semantic segmentation network,road markings,traffic situations,weather conditions,sensor modalities,lighting,qualitative performance,real-time road marking detection,labelling effort,Oxford RobotCar dataset,CamVid dataset
AI 理解论文
溯源树
样例
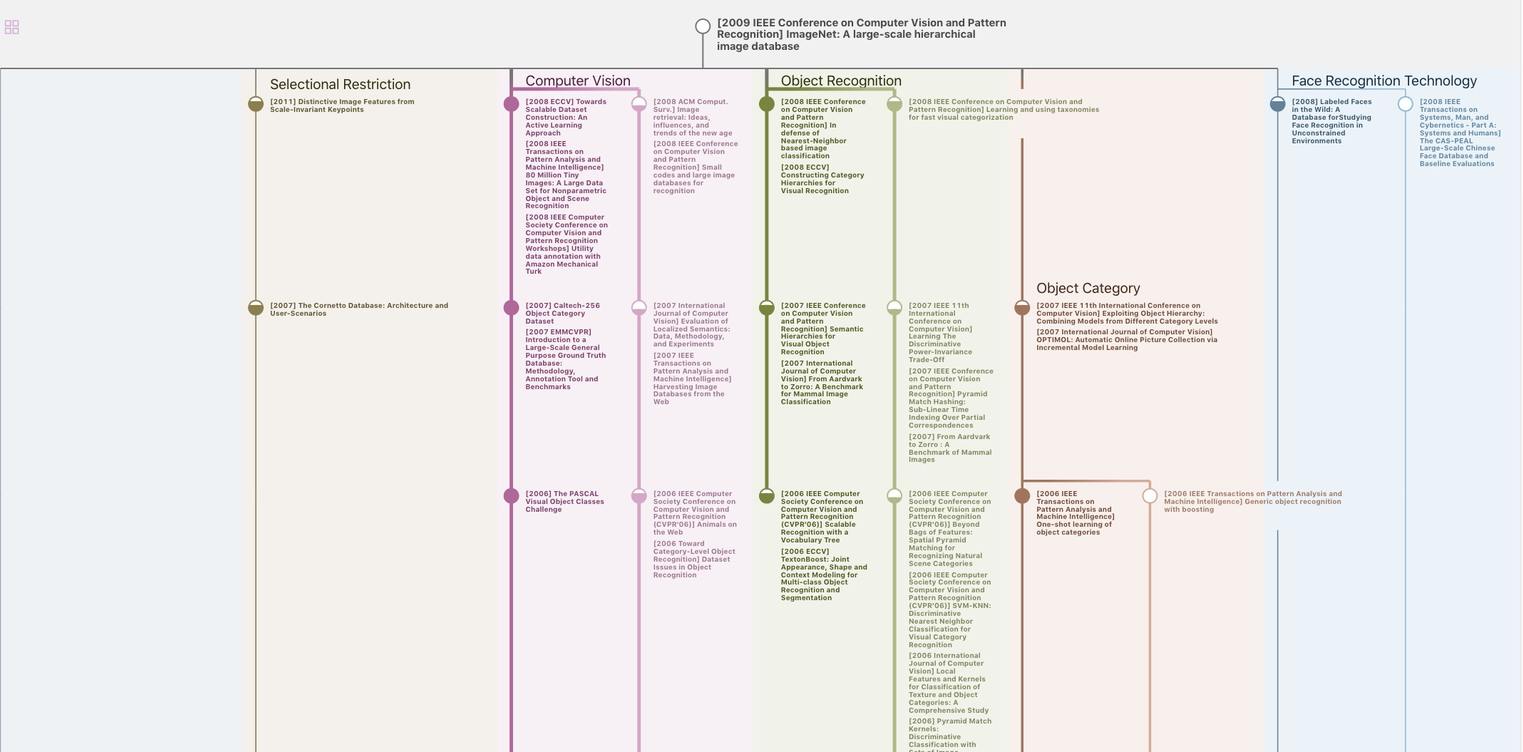
生成溯源树,研究论文发展脉络
Chat Paper
正在生成论文摘要