A New Method Of Online Fault Diagnosis Based On Incremental Continuous Attribute Naive Bayesian
2018 IEEE INTERNATIONAL CONFERENCE ON PROGNOSTICS AND HEALTH MANAGEMENT (ICPHM)(2018)
摘要
Diagnosing faults accurately and updating diagnosis model timely are the two key points for the online fault diagnosis. Considering the higher diagnostic accuracy and the greater model updating efficiency, this paper proposes an improved incremental Gaussian Naive Bayesian learning algorithm (I-IGNB) to facilitate the online fault diagnosis. A crucial assumption in I-IGNB theory, i.e., the continuous characteristic data is generated by a single Gaussian distribution, where the information loss caused by discretization can be efficiently avoided in online fault diagnosis of continuous attributes. The updating mechanism of the proposed I-IGNB is to update the important parameters of the diagnosis model, the prior probability and the conditional probability with several explicit equations, which is quite computationally efficient. Moreover, when a new fault mode occurs in the online diagnosis, it can be accurately identified by the I-IGNB, by which the whole diagnostic accuracy can be further improved. To verify the effectiveness, a double second-order RC active filter circuit is designed to obtain fault data and test the performance of I-IGNB. Experimental results show that the I-IGNB is able to update diagnosis model quite fast and improve the diagnostic accuracy obviously with the model updating.
更多查看译文
关键词
online diagnosis, incremental learning, Gaussian, Naive Bayesian, continuous attributes
AI 理解论文
溯源树
样例
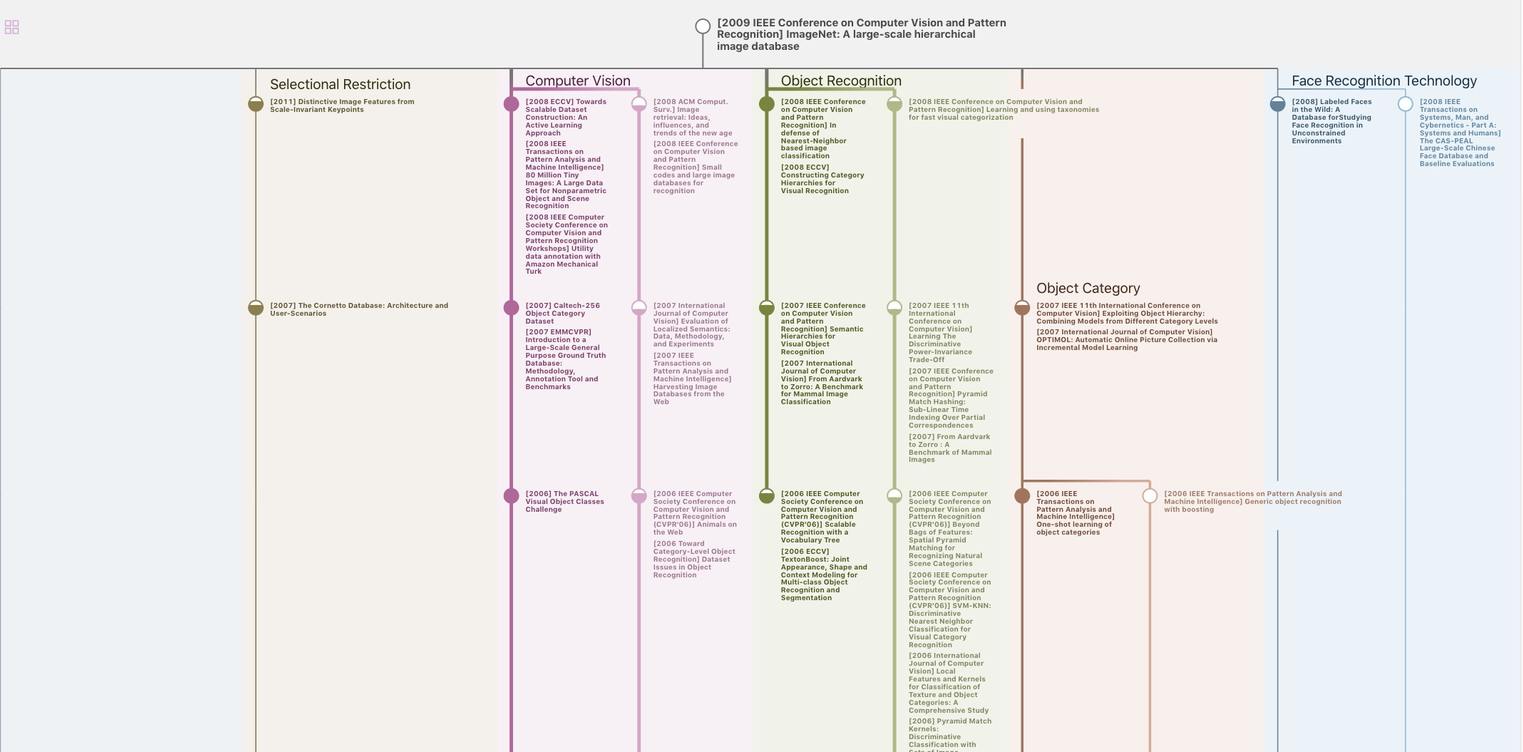
生成溯源树,研究论文发展脉络
Chat Paper
正在生成论文摘要