Learning-Based Modelized Combination of Evidence
2018 21ST INTERNATIONAL CONFERENCE ON INFORMATION FUSION (FUSION)(2018)
摘要
Evidence combination is typical uncertainty reasoning or information fusion in the theory of belief functions, which combines bodies of evidence stemming from different information sources. In traditional applications of evidence combination (e.g., pattern classification), given a sample, the basic belief assignments (BBAs) of different information sources are generated first, and then they are combined by a rule, e.g., Dempster's rule. In this paper, we propose a new modelized method for evidence combination. By just inputting the sample into the learned model of combination, a “combined” BBA is obtained. That is, it does not need to generate multiple BBAs for each sample for the combination. In our proposed modelized combination, we can generate different combination models with different combination rules. Experimental results and related analyses validate the rationality and efficiency of our proposed method.
更多查看译文
关键词
Belief functions, evidence combination, learning, modelized combination, uncertainty
AI 理解论文
溯源树
样例
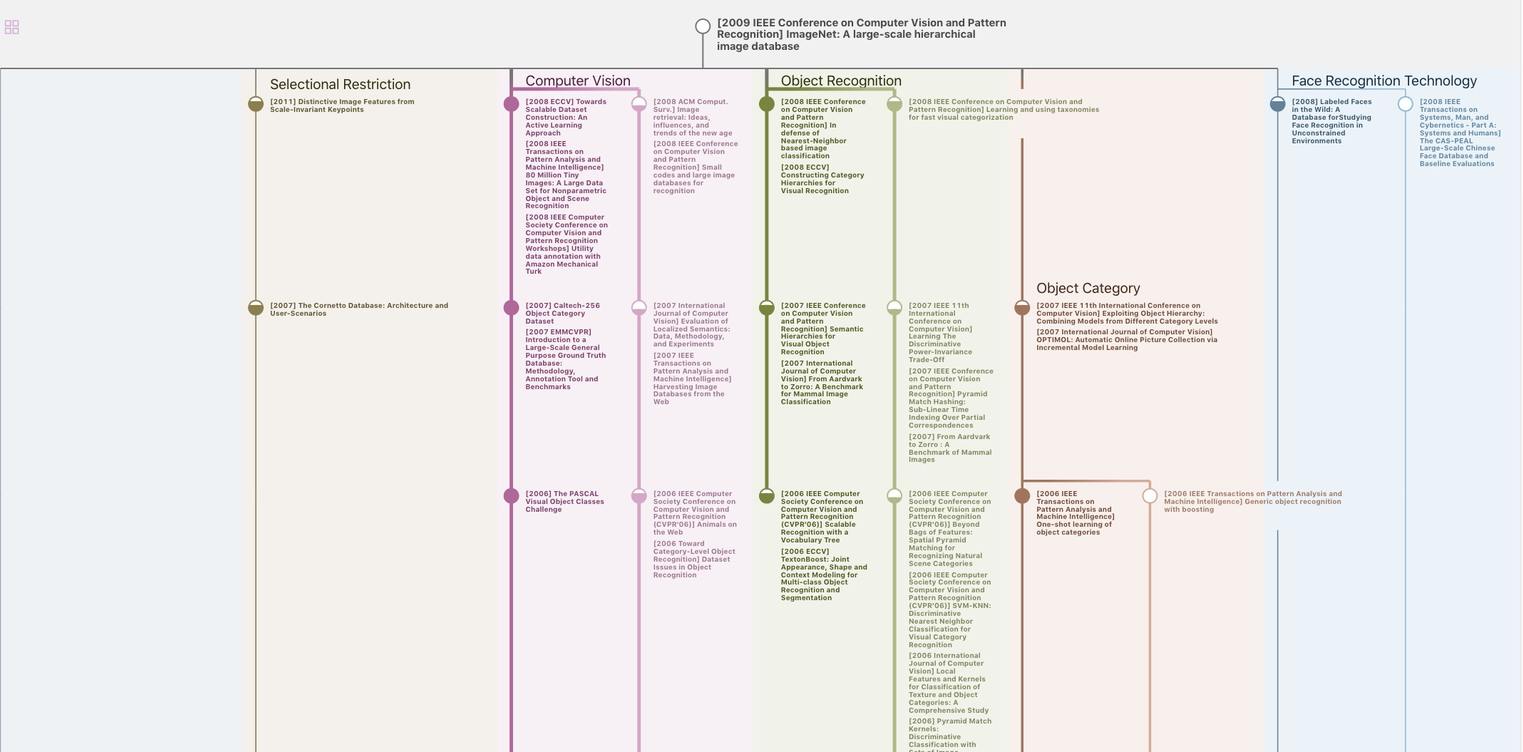
生成溯源树,研究论文发展脉络
Chat Paper
正在生成论文摘要