Transfer Learning For Segmentation Of Injured Lungs Using Coarse-To-Fine Convolutional Neural Networks
IMAGE ANALYSIS FOR MOVING ORGAN, BREAST, AND THORACIC IMAGES(2018)
摘要
Deep learning using convolutional neural networks (ConvNets) achieves high accuracy across many computer vision tasks, with the ability to learn multi-scale features and generalize across a variety of input data. In this work, we propose a deep learning framework that utilizes a coarse-to-fine cascade of 3D ConvNet models for segmentation of lung structures obtained from computed tomographic (CT) images. Deep learning requires a large number of training datasets, which may be challenging in medical imaging, especially for rare diseases. In the present study, transfer learning is utilized for lung segmentation of CT scans in large animal models of the acute respiratory distress syndrome (ARDS) using only 13 subjects. The method was quantitatively evaluated on a human dataset, consisting of 395 3D CT scans from 153 subjects, and an animal dataset consisting of 148 3D CT images from 5 porcine subjects. The human dataset achieved an average Jacaard index of 0.99, and an average symmetric surface distance (ASSD) of 0.29 mm. The animal dataset had an average Jacaard index of 0.94, and an ASSD of 0.99 mm.
更多查看译文
关键词
Segmentation, Computed tomography, Deep learning
AI 理解论文
溯源树
样例
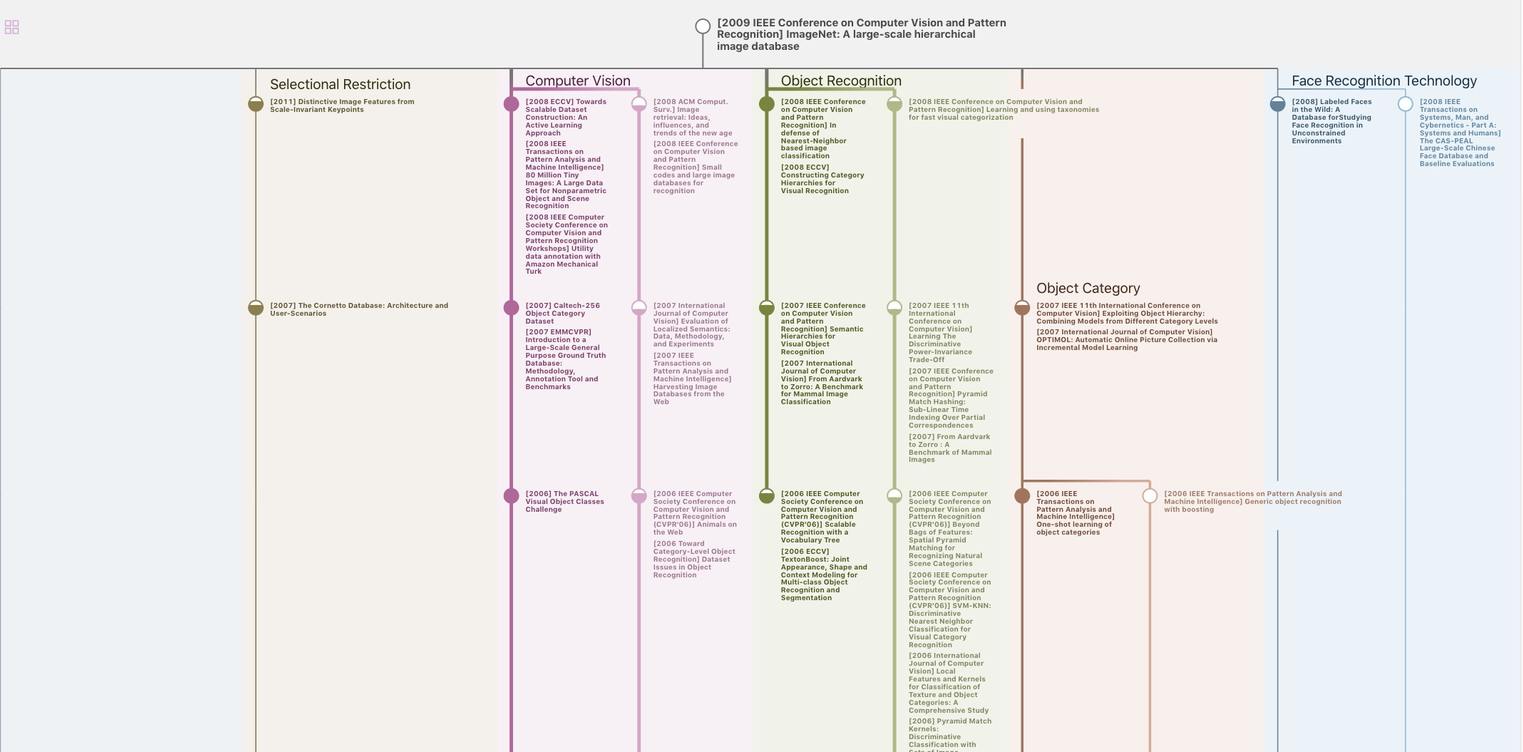
生成溯源树,研究论文发展脉络
Chat Paper
正在生成论文摘要