Integrating Deformable Modeling With 3d Deep Neural Network Segmentation
DEEP LEARNING IN MEDICAL IMAGE ANALYSIS AND MULTIMODAL LEARNING FOR CLINICAL DECISION SUPPORT, DLMIA 2018(2018)
摘要
Convolutional neural networks have advanced the state of the art in medical image segmentation. However, there are two challenges in 3D deep learning segmentation networks. First, the segmentation masks from deep learning networks lack shape constraints, often resulting in the need for post-processing. Second, the training and deployment of 3D networks require substantial memory resources. The memory requirement becomes an issue especially when the target organs cover a large footprint. Commonly down-sampling and up-sampling operations are needed before and after the network. To address the post-processing requirement, we present a new loss function that incorporates the level set based smoothing loss together with multi Dice loss to avoid an additional post processing step. The formulation is general and can accommodate other deformable shape models. Further, we propose a way to integrate the down-and up-sampling in the network such that the input of the deep learning network can work directly on the original image without a significant increase in the memory usage. The 3D segmentation network with the proposed loss and sampling approach shows promising results on a dataset of 48 chest CT angiography images with 16 target anatomies. We obtained average Dice of 79.5% in 4 fold cross validation.
更多查看译文
AI 理解论文
溯源树
样例
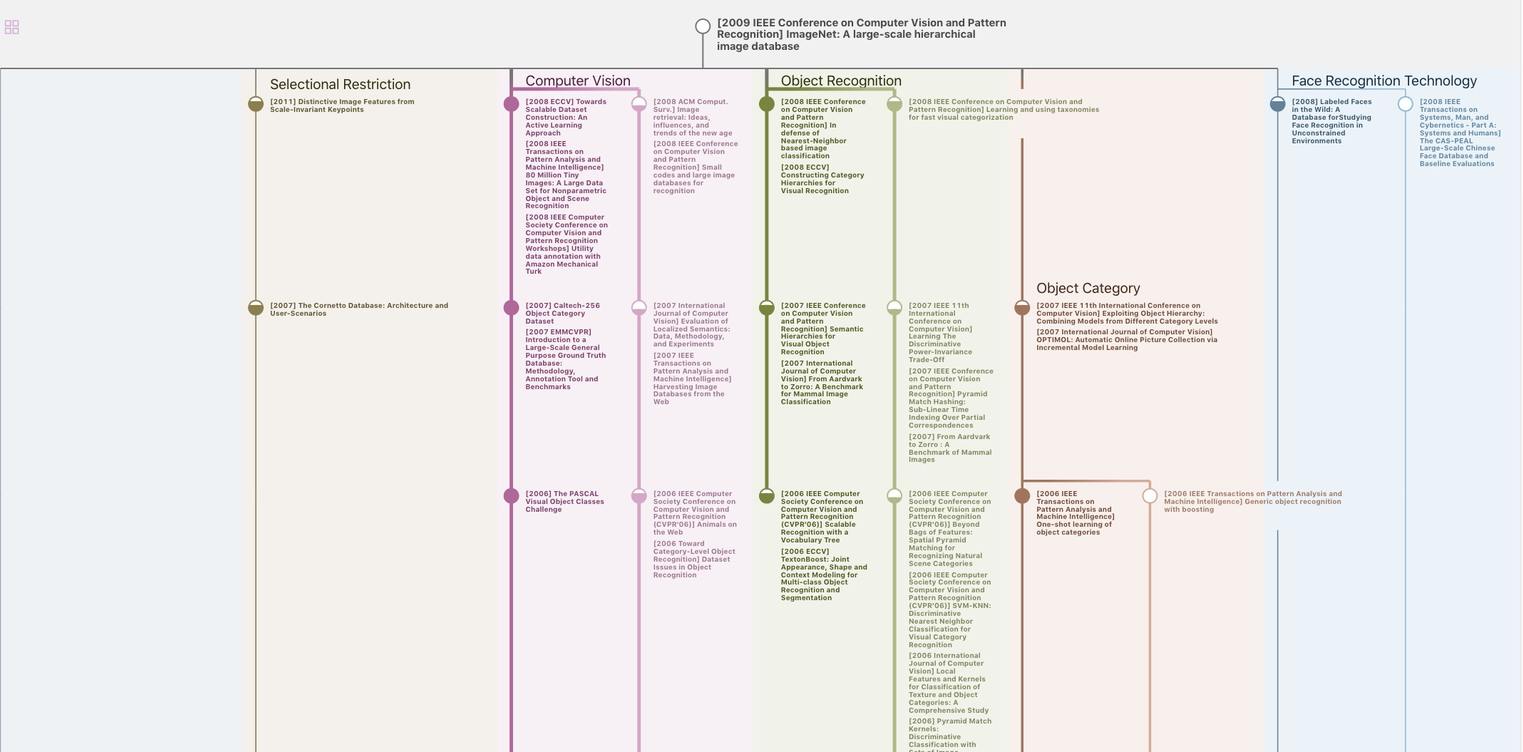
生成溯源树,研究论文发展脉络
Chat Paper
正在生成论文摘要