Cellular Community Detection For Tissue Phenotyping In Histology Images
COMPUTATIONAL PATHOLOGY AND OPHTHALMIC MEDICAL IMAGE ANALYSIS(2018)
摘要
A primary aim of detailed analysis of multi-gigapixel histology images is assisting pathologists for better cancer grading and prognostication. Several methods have been proposed for the analysis of histology images in the literature. However, these methods are often limited to the classification of two classes i.e., tumor and stroma. Also, most existing methods are based on fully supervised learning and require a large amount of annotations, which are very difficult to obtain. To alleviate these challenges, we propose a novel community detection algorithm for the classification of tissue in Whole-slide Images (WSIs). The proposed algorithm uses a novel graph-based approach to the problem of detecting prevalent communities in a collection of histology images in an semi-supervised manner resulting the identification of six distinct tissue phenotypes in the multi-gigapixel image data. We formulate the problem of identifying distinct tissue phenotypes as the problem of finding network communities using the geodesic density gradient in the space of potential interaction between different cellular components. We show that prevalent communities found in this way represent distinct and biologically meaningful tissue phenotypes. Experiments on two independent Colorectal Cancer (CRC) datasets demonstrate that the proposed algorithm outperforms current state-of-the-art methods.
更多查看译文
关键词
Community detection, Tissue phenotyping
AI 理解论文
溯源树
样例
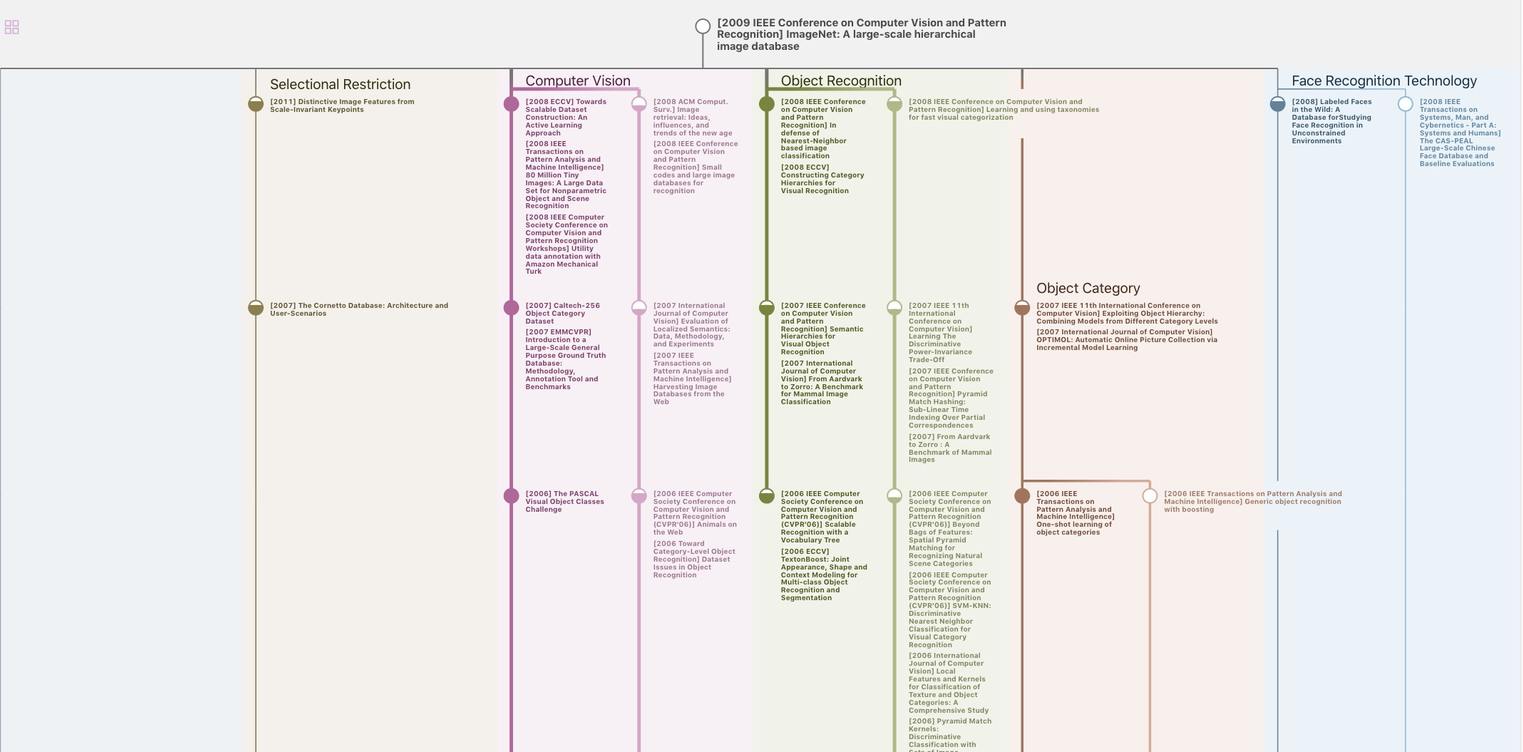
生成溯源树,研究论文发展脉络
Chat Paper
正在生成论文摘要