Time Series Sanitization with Metric-Based Privacy
2018 IEEE International Congress on Big Data (BigData Congress)(2018)
摘要
The increasing popularity of connected devices has given rise to the vast generation of time series data. Due to consumer privacy concerns, the data collected from individual devices must be sanitized before sharing with untrusted third-parties. However, existing time series privacy solutions do not provide provable guarantees for individual time series and may not extend to data from a wide range of application domains. In this paper, we adopt a generalized privacy notion based on differential privacy for individual time series sanitization and Discrete Cosine Transform to model the characteristics of time series data. We extend previously reported 2-dimensional results to arbitrary k-dimensional space. Empirical evaluation with various datasets demonstrates the applicability of our proposed method with the standard mean squared error (MSE) and in classification tasks.
更多查看译文
关键词
Time Series,Differential Privacy,Sampling
AI 理解论文
溯源树
样例
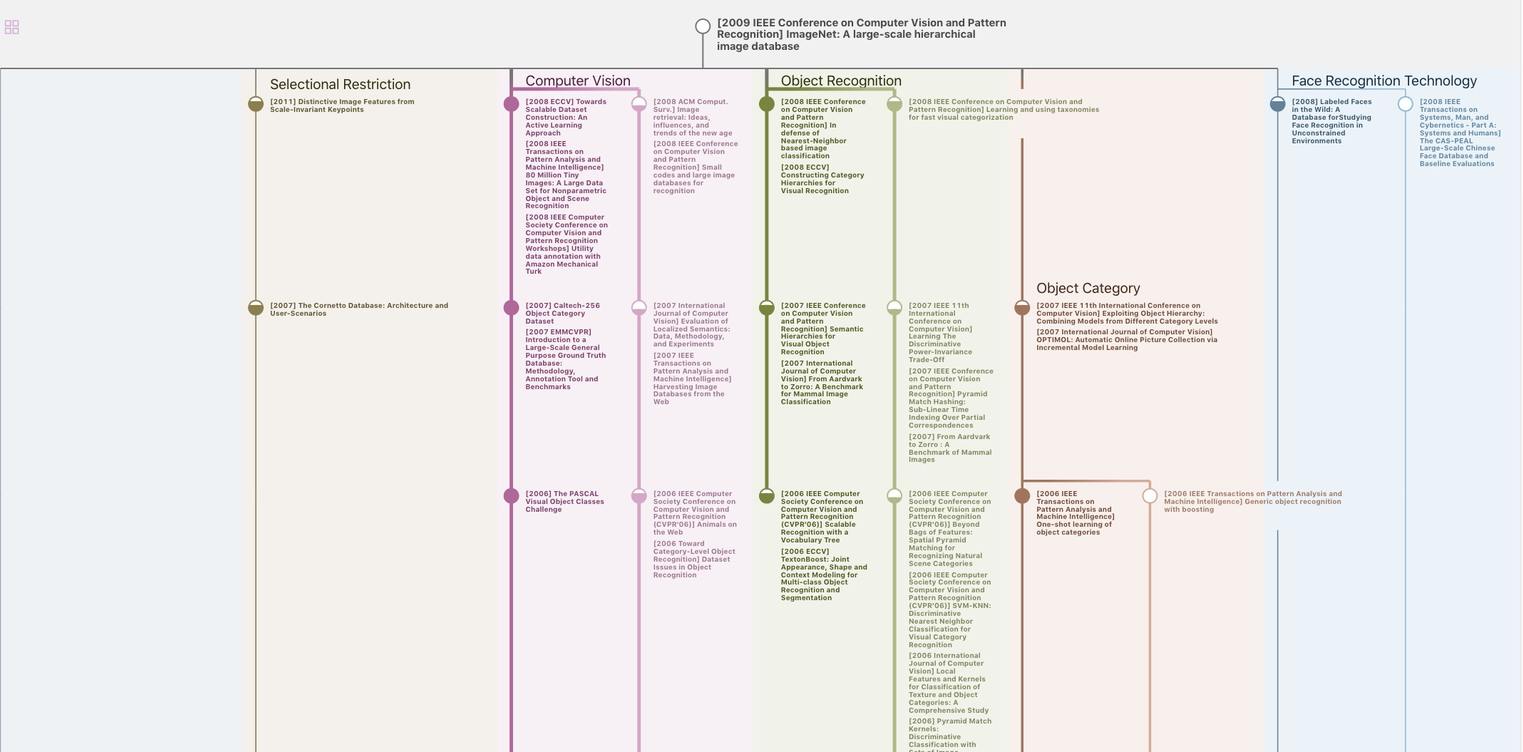
生成溯源树,研究论文发展脉络
Chat Paper
正在生成论文摘要