Detecting Anomalous Behavior of Black-Box Services Modeled with Distance-Based Online Clustering.
IEEE CLOUD(2018)
摘要
Reliable deployment of services is especially challenging in virtualized infrastructures, where the deep tech-nological stack and the multitude of components necessitate automatic anomaly detection and remediation mechanisms. Traditional monitoring solutions observe the system and generate alarms when the collected metrics exceed predefined thresholds. The fixed thresholds rely on expert knowledge and can lead to numerous false alarms, while abnormal behavior that spans over multiple metrics, components, or system layers, may not be detected. We propose to use an unsupervised online clustering algorithm to create a model of the normal behavior of each monitored component with minimal human interaction and no impact on the monitored system. When an anomaly is detected, a human administrator or automatic remediation system can subsequently revert the component into a normal state. An experimental evaluation resulted in a high accuracy of our approach, indicating that it is suitable for anomaly detection in productive systems.
更多查看译文
关键词
cloud computing, service virtualization, anomaly detection, machine learning
AI 理解论文
溯源树
样例
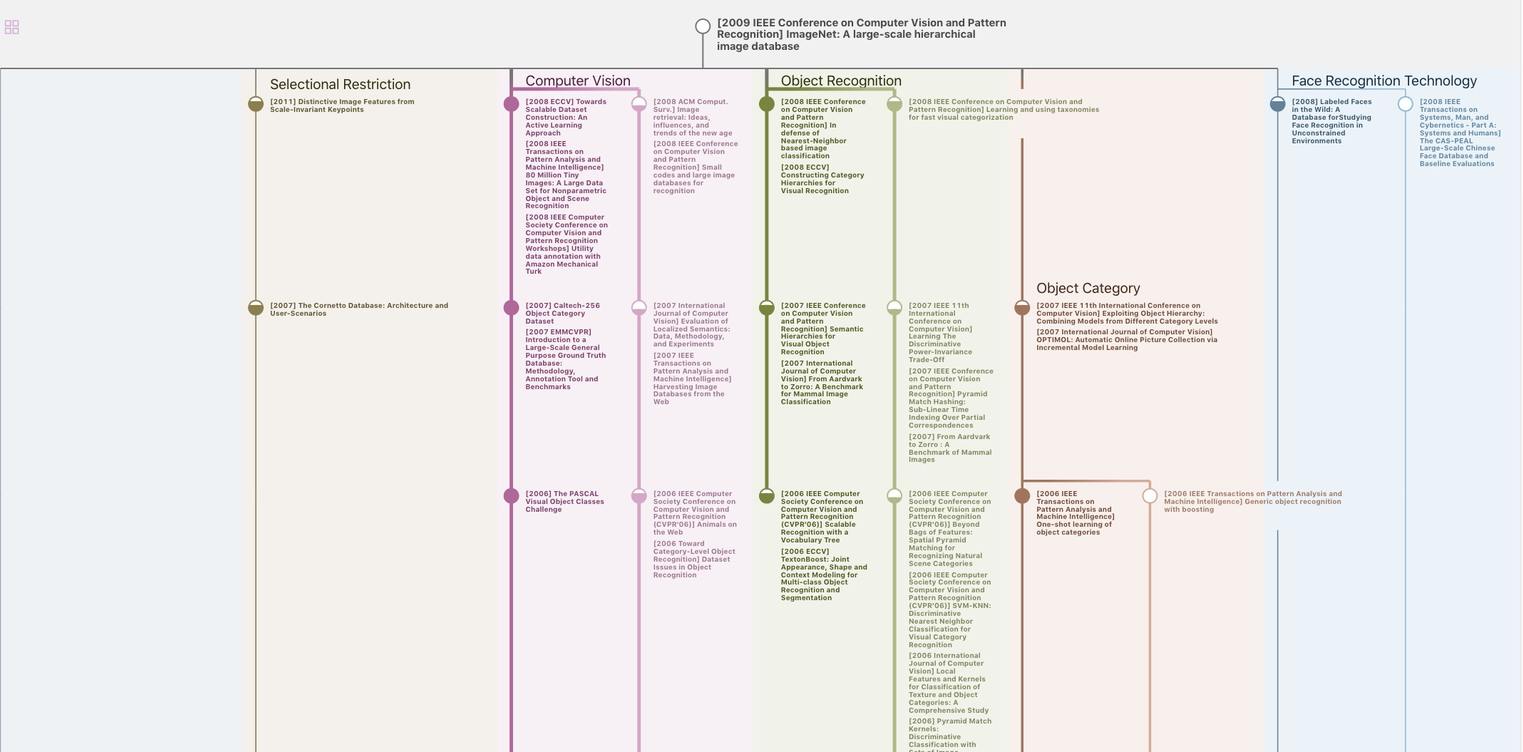
生成溯源树,研究论文发展脉络
Chat Paper
正在生成论文摘要