Decision Trees as Interpretable Bank Credit Scoring Models.
Communications in Computer and Information Science(2018)
摘要
We evaluate several approaches to classification of loan applications that provide their final results in the form of a single decision tree, i.e., in the form widely regarded as interpretable by humans. We apply state-of-the-art credit scoring-oriented classification algorithms, such as logistic regression, gradient boosting decision trees and random forests, as components of the proposed algorithms of decision tree building. We use four real-world loan default prediction data sets of different sizes. We evaluate the proposed methods using the area under the receiver operating characteristic curve (AUC) but we also measure the models' interpretability. We verify the significance of differences between AUC values observed when using the compared techniques by measuring Friedman's statistic and performing Nemenyi's post-hoc test.
更多查看译文
关键词
Data extraction and integration,Credit scoring,Expert systems and artificial intelligence,Big data,Data processing performance
AI 理解论文
溯源树
样例
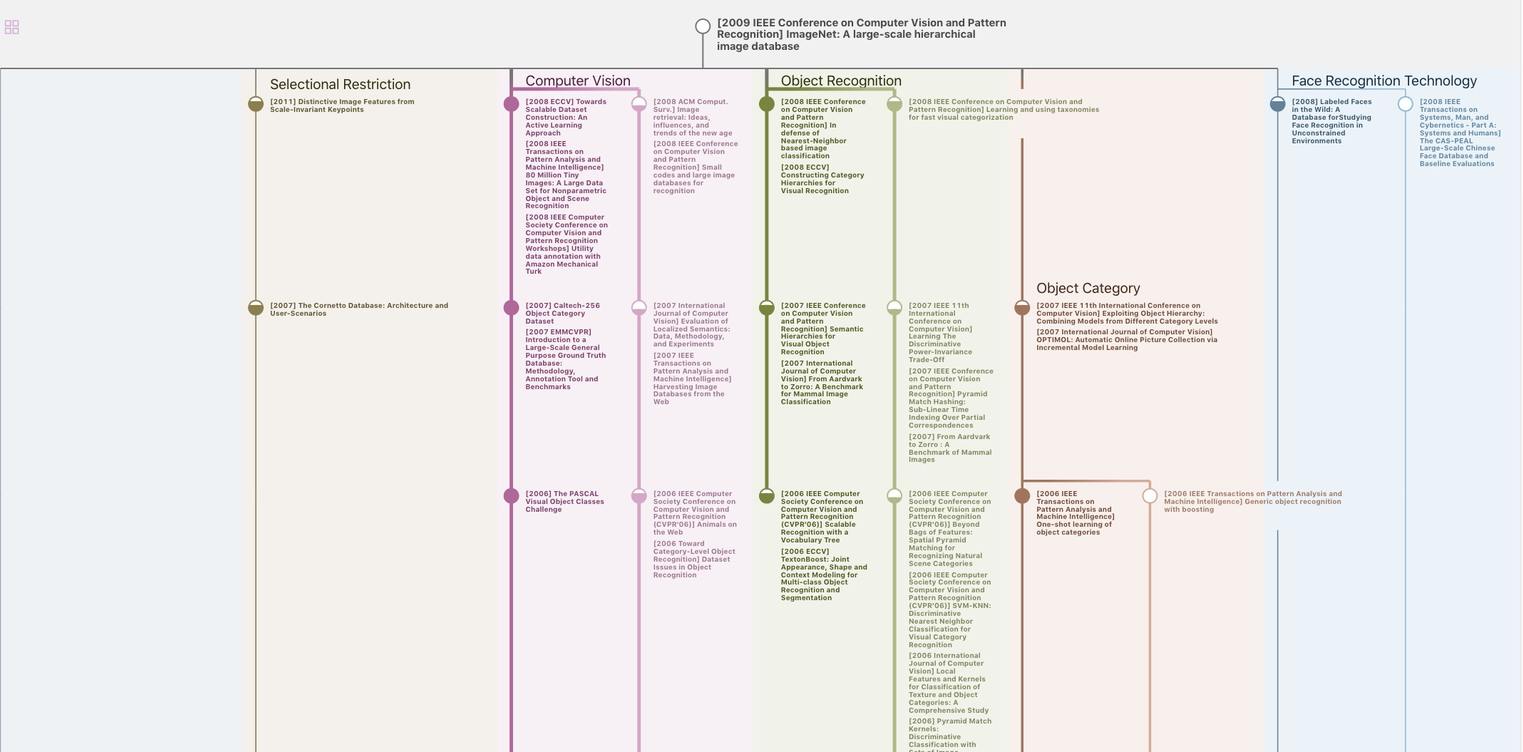
生成溯源树,研究论文发展脉络
Chat Paper
正在生成论文摘要