Semantic-Fusion Gans for Semi-Supervised Satellite Image Classification.
ICIP(2018)
摘要
Most of the public satellite image datasets contain only a small number of annotated images. The lack of a sufficient quantity of labeled data for training is a bottleneck for the use of modern deep-learning based classification approaches in this domain. In this paper we propose a semi -supervised approach to deal with this problem. We use the discriminator $(D)$ of a Generative Adversarial Network (GAN) as the final classifier, and we train $D$ using both labeled and unlabeled data. The main novelty we introduce is the representation of the visual information fed to $D$ by means of two different channels: the original image and its “semantic” representation, the latter being obtained by means of an external network trained on ImageNet. The two channels are fused in $D$ and jointly used to classify fake images, real labeled and real unlabeled images. We show that using only 100 labeled images, the proposed approach achieves an accuracy close to 69% and a significant improvement with respect to other GAN-based semi-supervised methods. Although we have tested our approach only on satellite images, we do not use any domain-specific knowledge. Thus, our method can be applied to other semi-supervised domains.
更多查看译文
关键词
semi-supervised learning, generative adversarial networks, satellite image classification
AI 理解论文
溯源树
样例
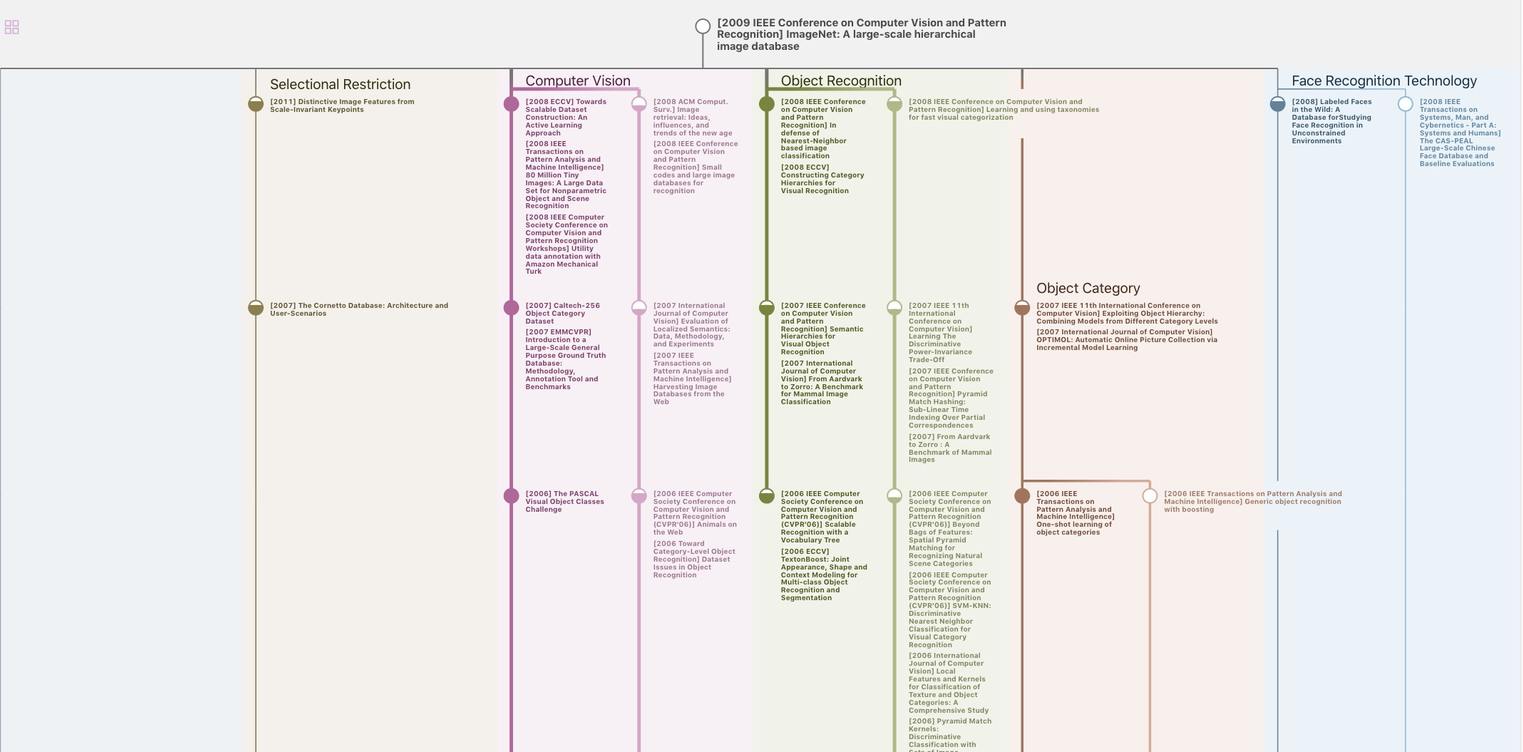
生成溯源树,研究论文发展脉络
Chat Paper
正在生成论文摘要