Learning Local And Deep Features For Efficient Cell Image Classification Using Random Forests
2018 25TH IEEE INTERNATIONAL CONFERENCE ON IMAGE PROCESSING (ICIP)(2018)
摘要
Automatic image classification systems for indirect immunofluorescence (IIF) labeling of human epithelial (HEp-2) cell specimens are needed to improve the efficient management of autoimmune diseases. In this paper, we propose to classify HEp-2 cell specimen imagery using a combination of local features and deep learning features extracted from the IIF images. Two local descriptors are used to capture texture information, namely: Rotation Invariant Co-occurrence among Local Binary Patterns (RIC-LBP) extending the LBP descriptor and Joint Motif Labels (JML) based on the Peano scan motif concept. Deep learning features are then extracted using the VGG-19 image classification network. Finally, all descriptors are combined using a late fusion approach with a Random Forests (RF) classifier with seven output classes. Experimental results show that our proposed framework achieves a mean class accuracy of 92.11% with five-fold cross validation using the RF classifier with 1000 trees on the HEp-2 specimen benchmark dataset, which outperforms the state-of-the-art accuracy on this dataset.
更多查看译文
关键词
Feature Extraction, Random Forests, Local Features, Deep Learning, Image Classification
AI 理解论文
溯源树
样例
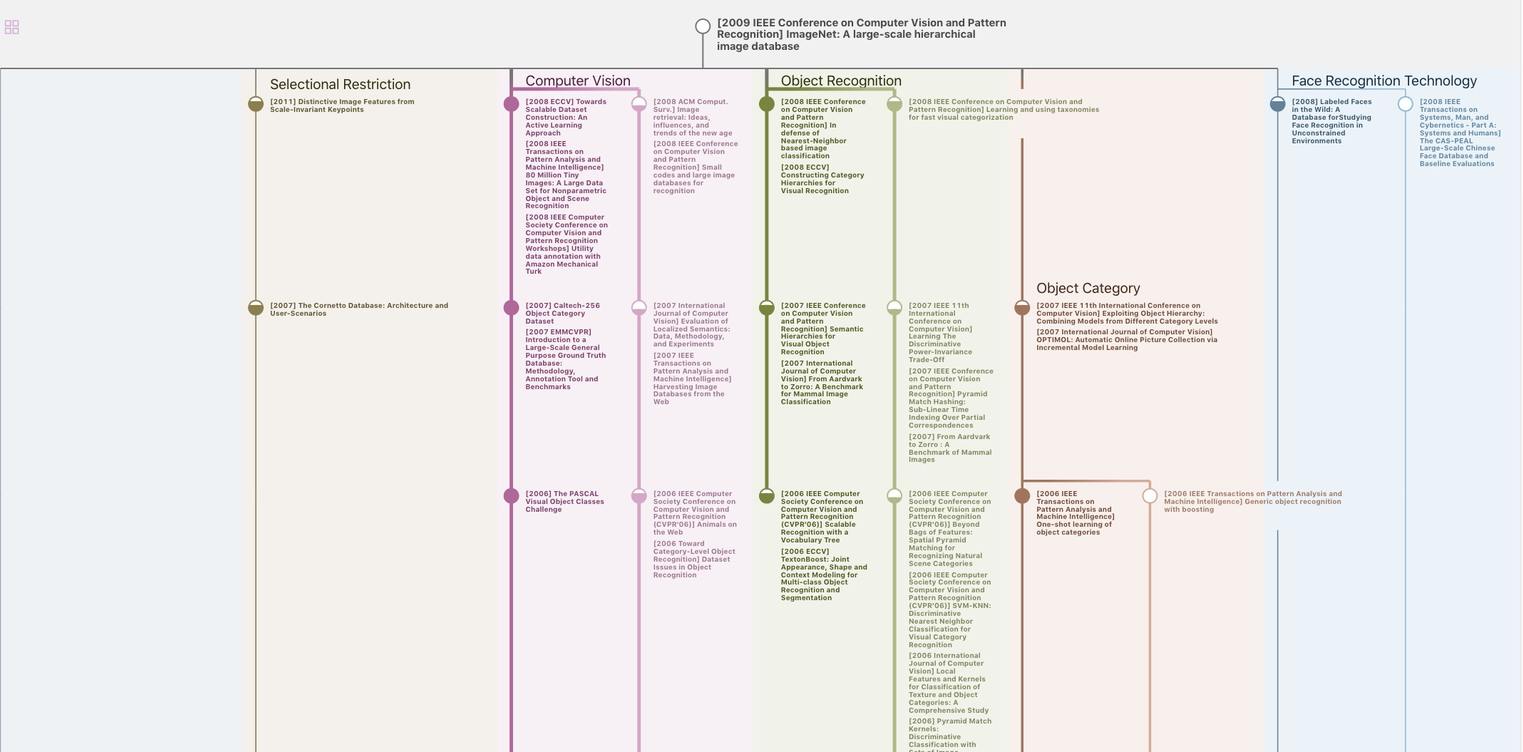
生成溯源树,研究论文发展脉络
Chat Paper
正在生成论文摘要