Making Deep Neural Network Fooling Practical
2018 25TH IEEE INTERNATIONAL CONFERENCE ON IMAGE PROCESSING (ICIP)(2018)
摘要
With the success of deep neural networks (DNNs), the robustness of such models under adversarial or fooling attacks has become extremely important. It has been shown that a simple perturbation of the image, invisible to a human observer, is sufficient to fool a deep network. Building on top of such work, methods have been proposed to generate adversarial samples which are robust to natural perturbations (camera noise, rotation, shift, scaling etc.). In this paper, we review multiple such fooling algorithms and show that the generated adversarial samples exhibit distributions largely different from the true distribution of the training samples, and thus are easily detectable by a simple meta classifier. We argue that for truly practical DNN fooling, not only should the adversarial samples be robust against various distortions, but must also follow the training set distribution and be undetectable from such meta classifiers. Finally we propose a new adversarial sample generation technique that outperforms commonly known methods when evaluated simultaneously on robustness and detectability.
更多查看译文
关键词
Deep Network Fooling, Robustness of Adversarial Attacks
AI 理解论文
溯源树
样例
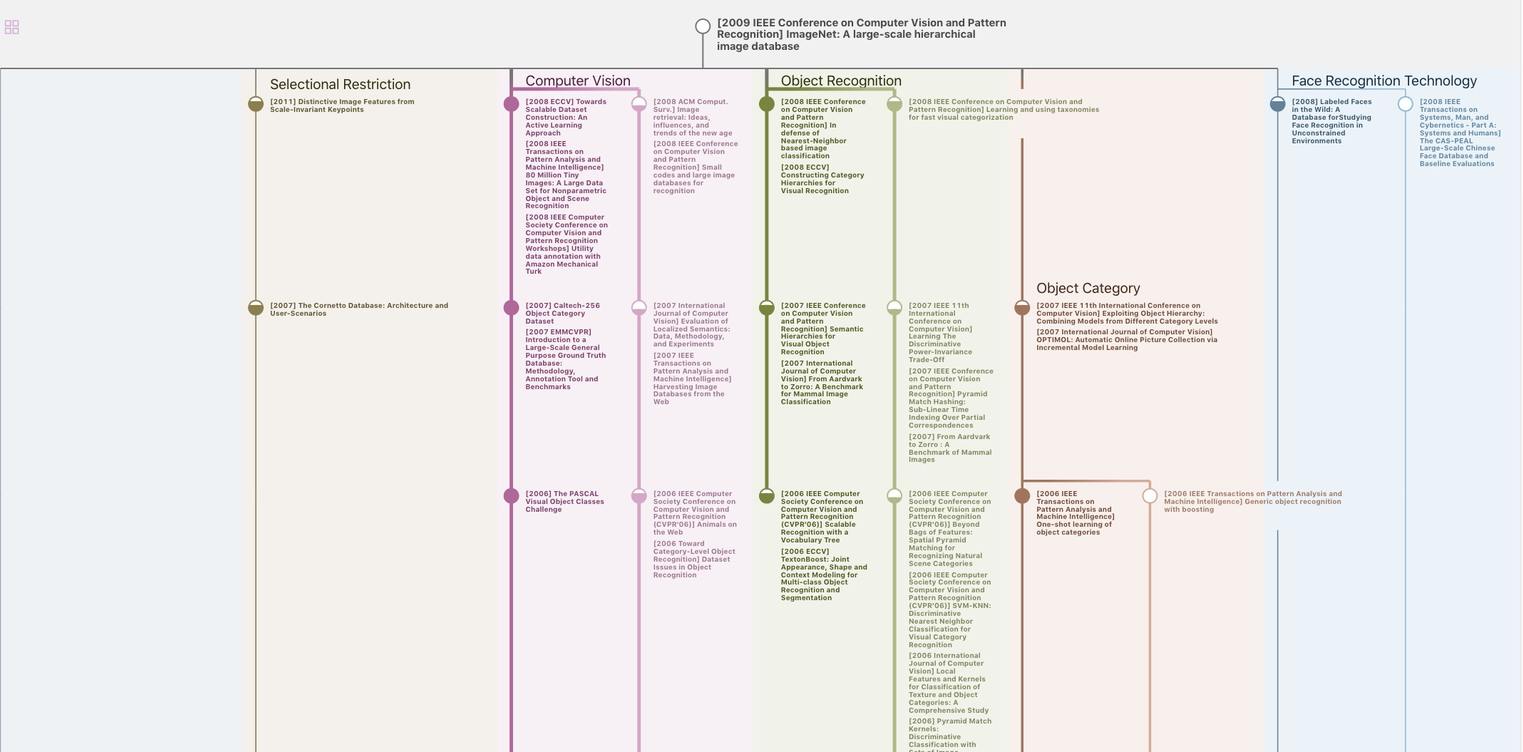
生成溯源树,研究论文发展脉络
Chat Paper
正在生成论文摘要