Maximum Likelihood Estimation Of Regularisation Parameters
2018 25TH IEEE INTERNATIONAL CONFERENCE ON IMAGE PROCESSING (ICIP)(2018)
摘要
This paper presents an empirical Bayesian method to estimate regularisation parameters in imaging inverse problems. The method calibrates regularisation parameters directly from the observed data by maximum marginal likelihood estimation, and is useful for inverse problems that are convex. A main novelty is that maximum likelihood estimation is performed efficiently by using a stochastic proximal gradient algorithm that is driven by two proximal Markov chain Monte Carlo samplers, intimately combining modern optimisation and sampling techniques. The proposed methodology is illustrated with an application to total-variation image deconvolution, where it compares favourably to alternative Bayesian and non-Bayesian approaches from the state of the art.
更多查看译文
关键词
Image processing, inverse problems, statistical inference, empirical Bayes, stochastic optimisation, Markov chain Monte Carlo methods, proximal algorithms
AI 理解论文
溯源树
样例
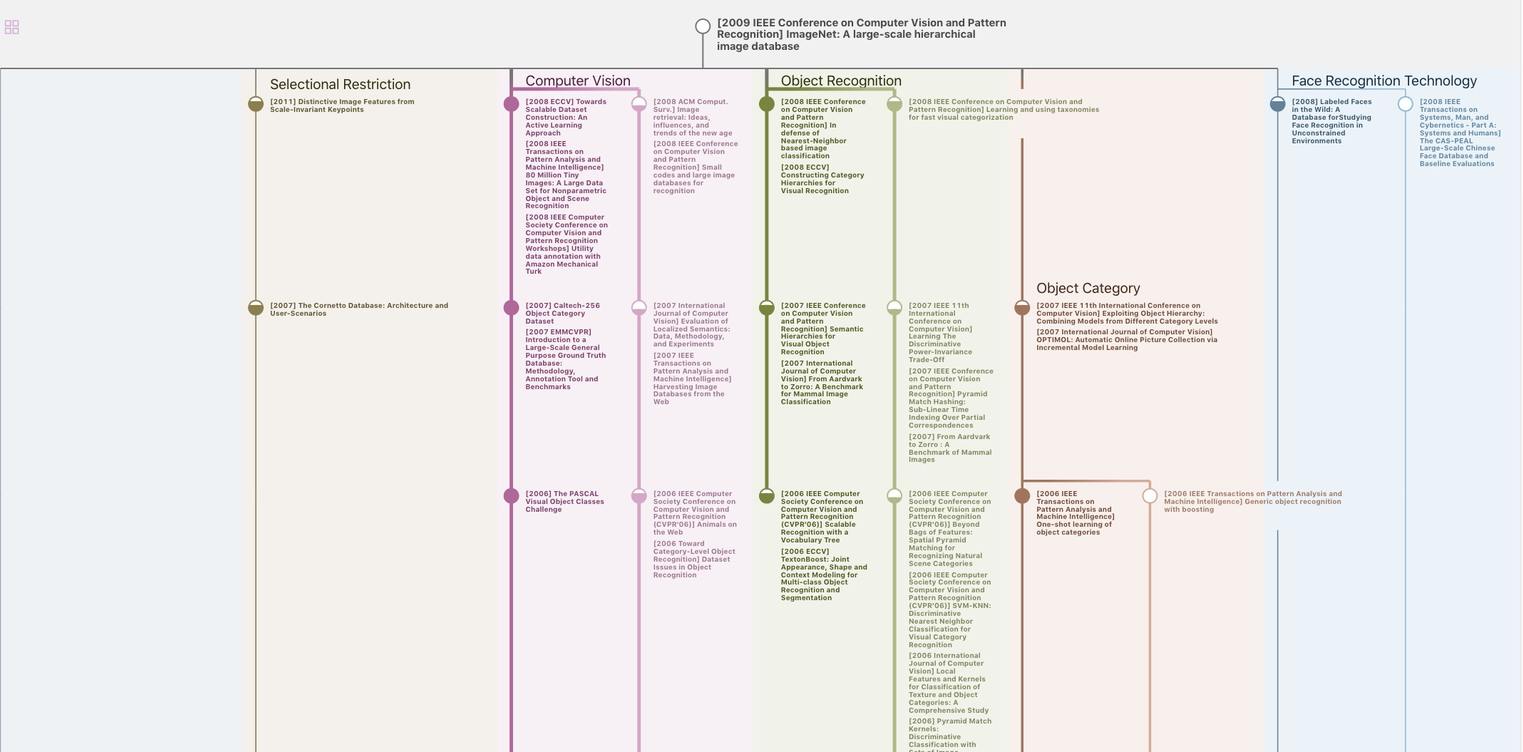
生成溯源树,研究论文发展脉络
Chat Paper
正在生成论文摘要