Dense Chained Attention Network For Scene Text Recognition
2018 25TH IEEE INTERNATIONAL CONFERENCE ON IMAGE PROCESSING (ICIP)(2018)
摘要
Reading text in the wild is a challenging task in computer vision. Scene text suffers from various background noise, including shadow, irrelevant symbols and background texture. In order to reduce the disturbance of background noise, we propose a dense chained attention network with stacked attention modules for scene text recognition. Each attention module learns the attention map that is adapted to corresponding features to enhance the foreground text and suppress the background noise. Besides, the attention branch is designed with the convolution-deconvolution structure which rapidly captures global information to guide the discriminative feature selection. We stack multiple attention modules to gradually refine the attention maps and capture both the low-level appearance feature and the high-level semantic information. Extensive experiments on the standard benchmarks, the Street View Text, IIIT5K, and ICDAR datasets validate the superiority of the proposed method. The dense chained attention network achieves state-of-the-art or highly competitive recognition performance.
更多查看译文
关键词
text recognition, attention, convolution-deconvolution
AI 理解论文
溯源树
样例
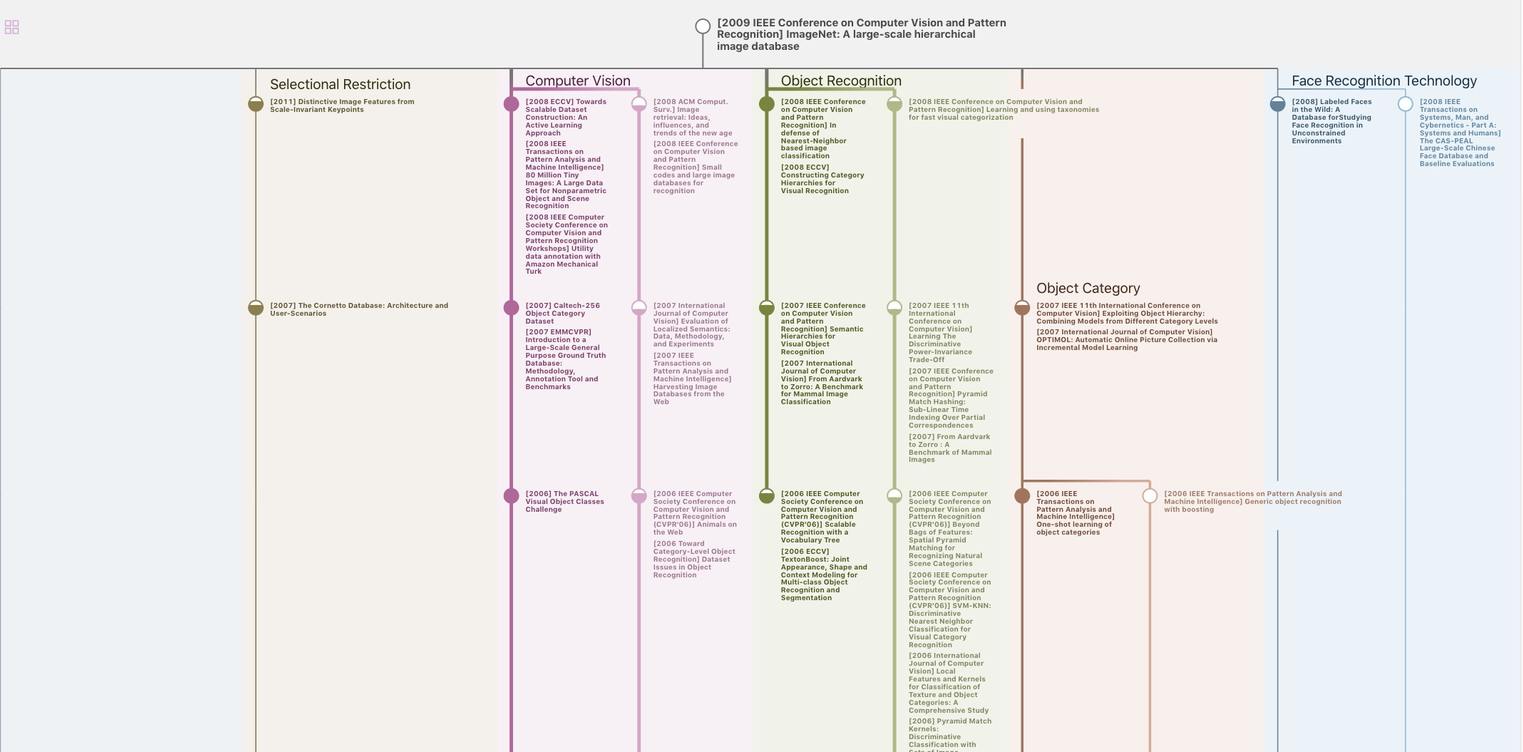
生成溯源树,研究论文发展脉络
Chat Paper
正在生成论文摘要