Graph-Laplacian Correlated Low-Rank Representation For Subspace Clustering
2018 25TH IEEE INTERNATIONAL CONFERENCE ON IMAGE PROCESSING (ICIP)(2018)
摘要
Subspace clustering seeks to segment a given unlabeled data into clusters with the hope of each cluster corresponding to a union of low-dimensional subspaces. Among them, low-rank representation (LRR) is a promising potential method which intends to build a good affinity matrix by using the self-expression of inputs. However, it completely ignores the important data locality. Although several works in this regard have considered the local geometric structure through the Laplacian regularizer, they also neglect the correlation of the data. In this paper, we propose a graph-Laplacian correlated low-rank representation model (GCLRR) to address such an issue. Particularly, GCLRR factorizes the self-expression as the product of two low-dimensional matrices, of which one is the latent representation of the self-expression. On the basis of the latent representation, the Laplacian regularizer is integrated with the orthogonal constraint together and behaves like the spectral clustering. Moreover, we devise a Frobenius norm based trace loss and use it to constrain both the latent representation and the self-expression to capture the correlation of the data. Our improved trace loss is more efficient than the original one. More importantly, GCLRR provides an effective unified framework to seamlessly integrate both aspects above. Then, we optimize GCLRR in the frame of alternating direction method (ADM) and fortunately derive the analytical solution to each subproblem. Experiments of motion segmentation and image clustering confirm the efficacy of the proposed GCLRR.
更多查看译文
关键词
Subspace clustering, graph-Laplacian regularizer, adaptive correlation, the self-expression
AI 理解论文
溯源树
样例
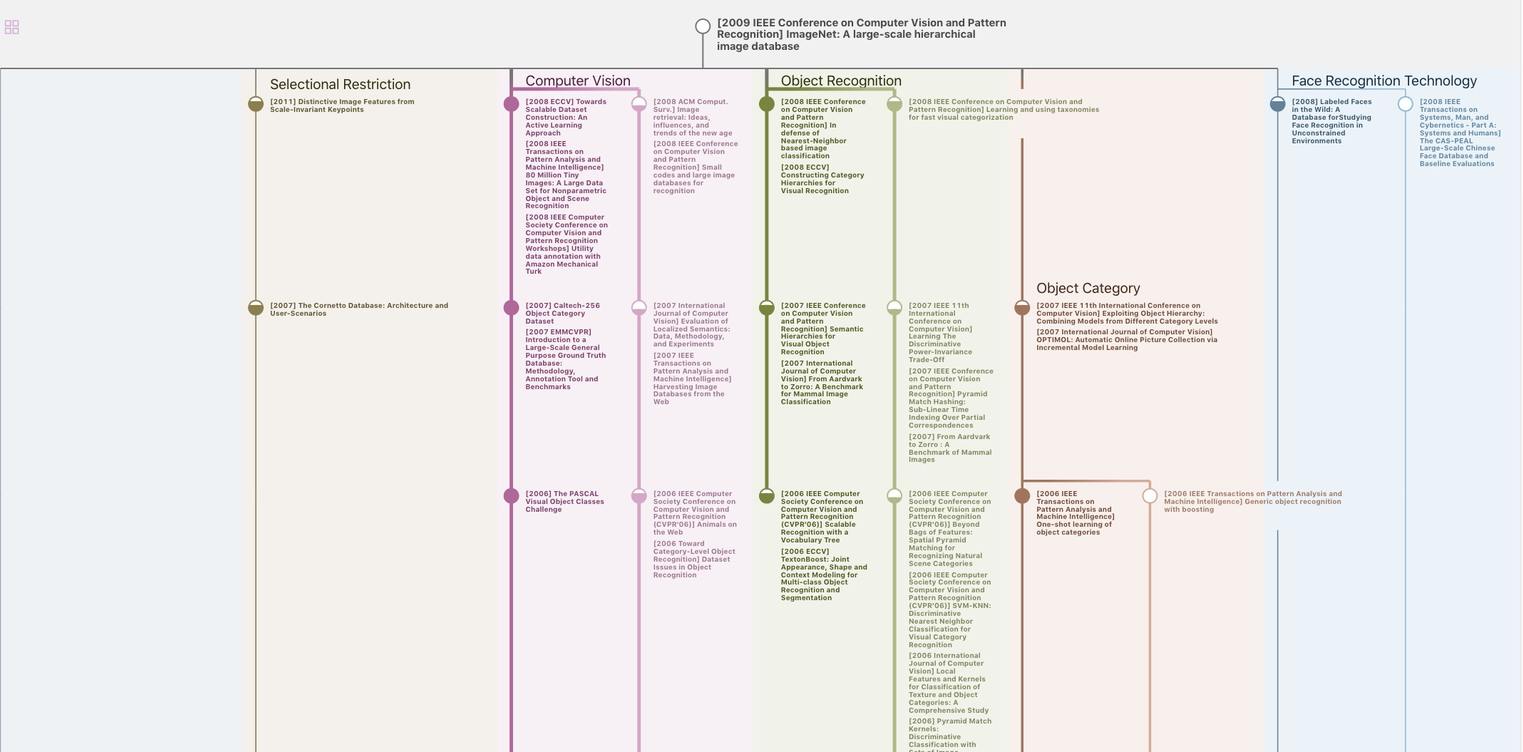
生成溯源树,研究论文发展脉络
Chat Paper
正在生成论文摘要