Towards Heart Sound Classification Without Segmentation Using Convolutional Neural Network
2017 COMPUTING IN CARDIOLOGY (CINC)(2017)
摘要
Heart sound classification is an effective and convenient method for the preliminary diagnosis of heart diseases, it provides physiology and pathology information to determine whether further expert diagnosis is needed. However, one of the most difficult problems for heart sound classification is heart sound segmentation. In this study, we proposed a method for heart sound classification without segmentation using convolutional neural network (CNN). In the proposed method, the heart cycles with different start positions are intercepted from the heart sound signals during the training phase. Then the spectrograms of the intercepted heart cycles are scaled to a fixed size and input to the designed CNN architecture. Thus, the trained CNN is able to generate features of different start positions in the testing phase. Therefore, heart sound segmentation is not necessary for prediction in the proposed method. At last, the classification task is completed by support vector machine (SVM). Moreover, the proposed method is evaluated on two public datasets offered by the PASCAL classifying heart sounds challenge. The results show that the proposed method is competitive compared with the methods using heart sound segmentation information, especially that the performance improvement is not obvious when the segmentation information is used in the testing phase of the proposed method.
更多查看译文
关键词
convolutional neural network,heart diseases,heart sound signals,intercepted heart cycles,heart sound segmentation information,heart sound classification,PASCAL
AI 理解论文
溯源树
样例
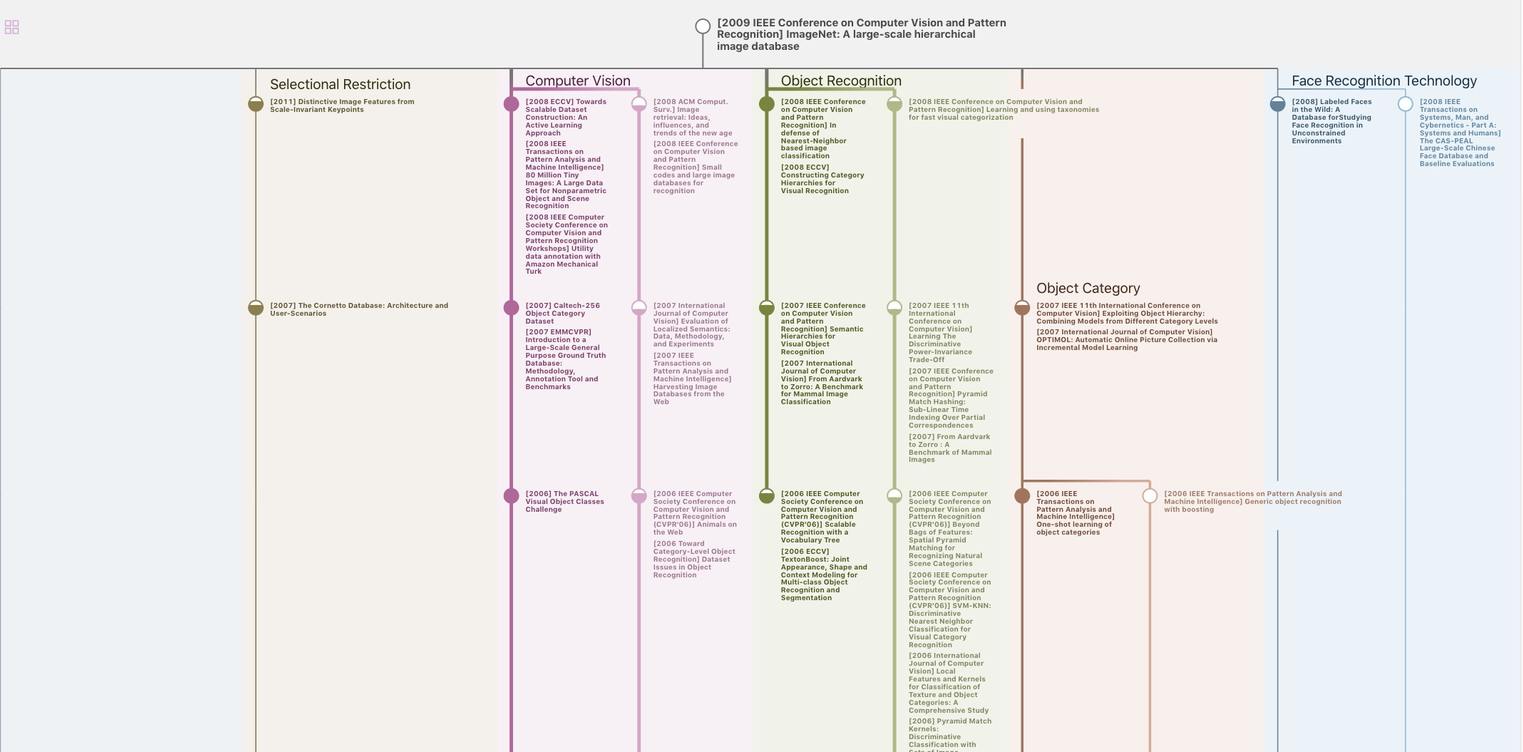
生成溯源树,研究论文发展脉络
Chat Paper
正在生成论文摘要