Probabilistic Verb Selection for Data-to-Text Generation.
TACL(2018)
摘要
In data-to-text Natural Language Generation (NLG) systems, computers need to find the right words to describe phenomena seen in the data. This paper focuses on the problem of choosing appropriate verbs to express the direction and magnitude of a percentage change (e.g., in stock prices). Rather than simply using the same verbs again and again, we present a principled data-driven approach to this problem based on Shannonu0027s noisy-channel model so as to bring variation and naturalness into the generated text. Our experiments on three large-scale real-world news corpora demonstrate that the proposed probabilistic model can be learned to accurately imitate human authorsu0027 pattern of usage around verbs, outperforming the state-of-the-art method significantly.
更多查看译文
AI 理解论文
溯源树
样例
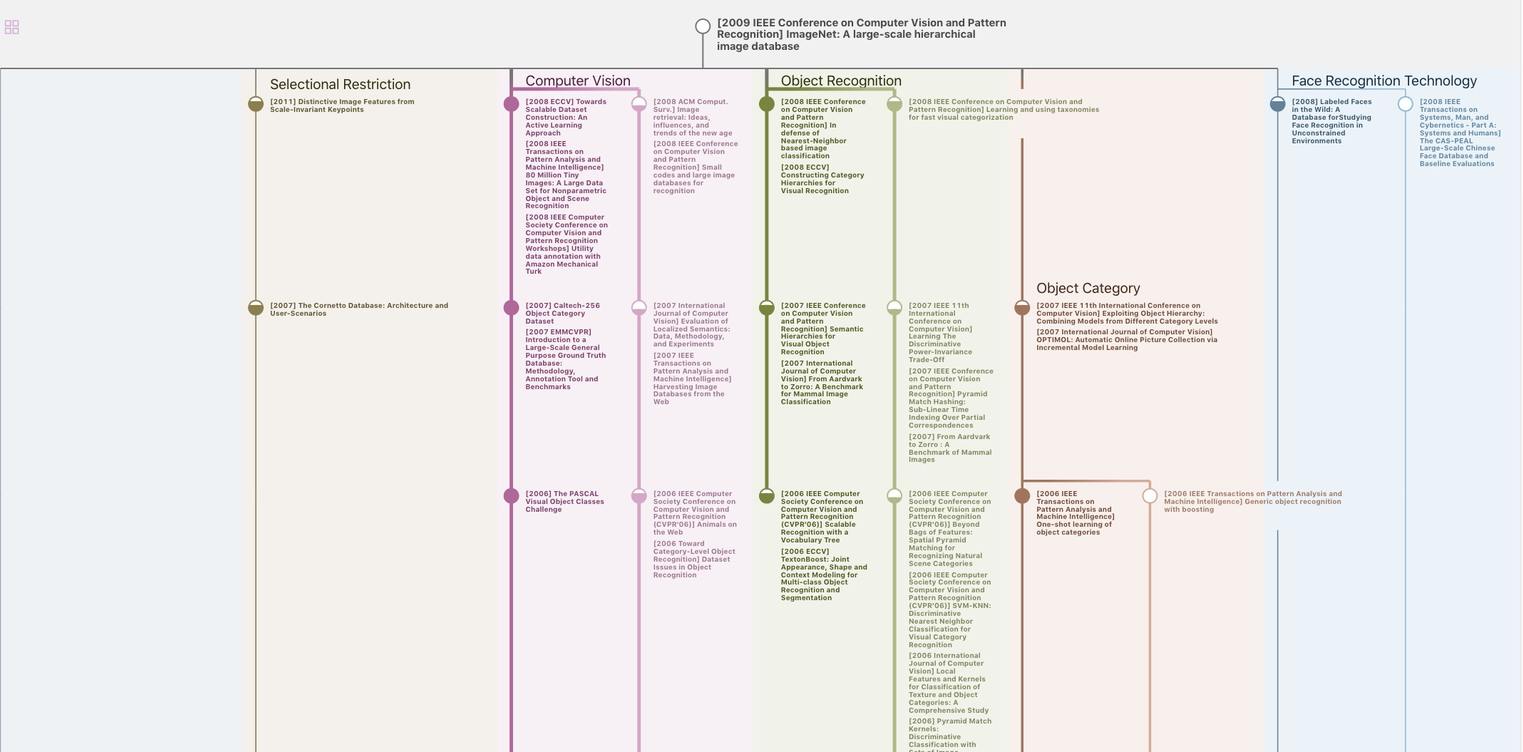
生成溯源树,研究论文发展脉络
Chat Paper
正在生成论文摘要