ParCorr: efficient parallel methods to identify similar time series pairs across sliding windows
Data Min. Knowl. Discov.(2018)
摘要
Consider the problem of finding the highly correlated pairs of time series over a time window and then sliding that window to find the highly correlated pairs over successive co-temporous windows such that each successive window starts only a little time after the previous window. Doing this efficiently and in parallel could help in applications such as sensor fusion, financial trading, or communications network monitoring, to name a few. We have developed a parallel incremental random vector/sketching approach to this problem and compared it with the state-of-the-art nearest neighbor method iSAX. Whereas iSAX achieves 100% recall and precision for Euclidean distance, the sketching approach is, empirically, at least 10 times faster and achieves 95% recall and 100% precision on real and simulated data. For many applications this speedup is worth the minor reduction in recall. Our method scales up to 100 million time series and scales linearly in its expensive steps (but quadratic in the less expensive ones).
更多查看译文
关键词
Time series analysis,Data stream processing,Distributed computing,Data mining
AI 理解论文
溯源树
样例
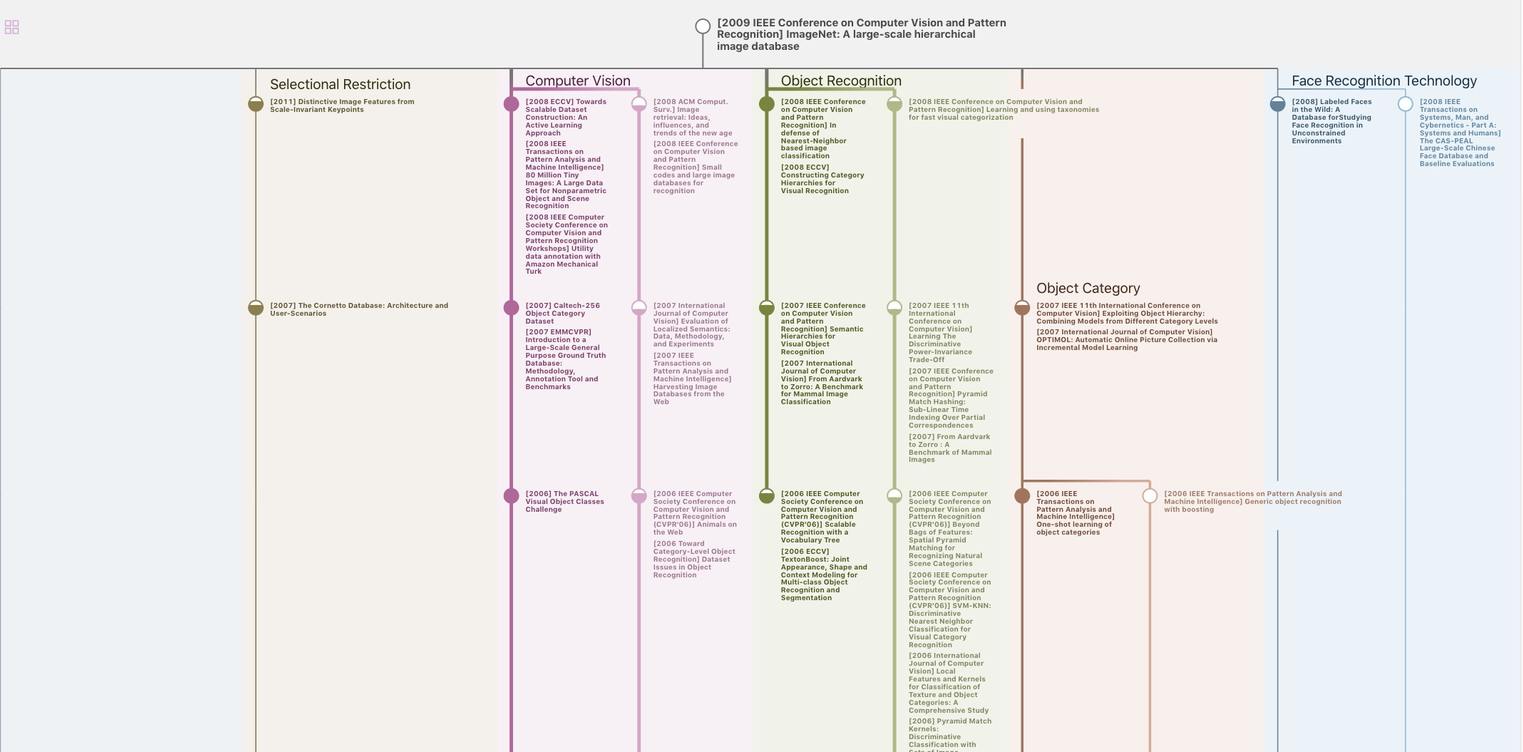
生成溯源树,研究论文发展脉络
Chat Paper
正在生成论文摘要