Efficiently summarizing attributed diffusion networks
Data Min. Knowl. Discov.(2018)
摘要
Given a large attributed social network, can we find a compact, diffusion-equivalent representation while keeping the attribute properties? Diffusion networks with user attributes such as friendship, email communication, and people contact networks are increasingly common-place in the real-world. However, analyzing them is challenging due to their large size. In this paper, we first formally formulate a novel problem of summarizing an attributed diffusion graph to preserve its attributes and influence-based properties. Next, we propose ANeTS, an effective sub-quadratic parallelizable algorithm to solve this problem: it finds the best set of candidate nodes and merges them to construct a smaller network of ‘super-nodes’ preserving the desired properties. Extensive experiments on diverse real-world datasets show that ANeTS outperforms all state-of-the-art baselines (some of which do not even finish in 14 days ). Finally, we show how ANeTS helps in multiple applications such as Topic-Aware viral marketing and sense-making of diverse graphs from different domains.
更多查看译文
关键词
Attributed graph,Summarization,Topic aware influence maximization
AI 理解论文
溯源树
样例
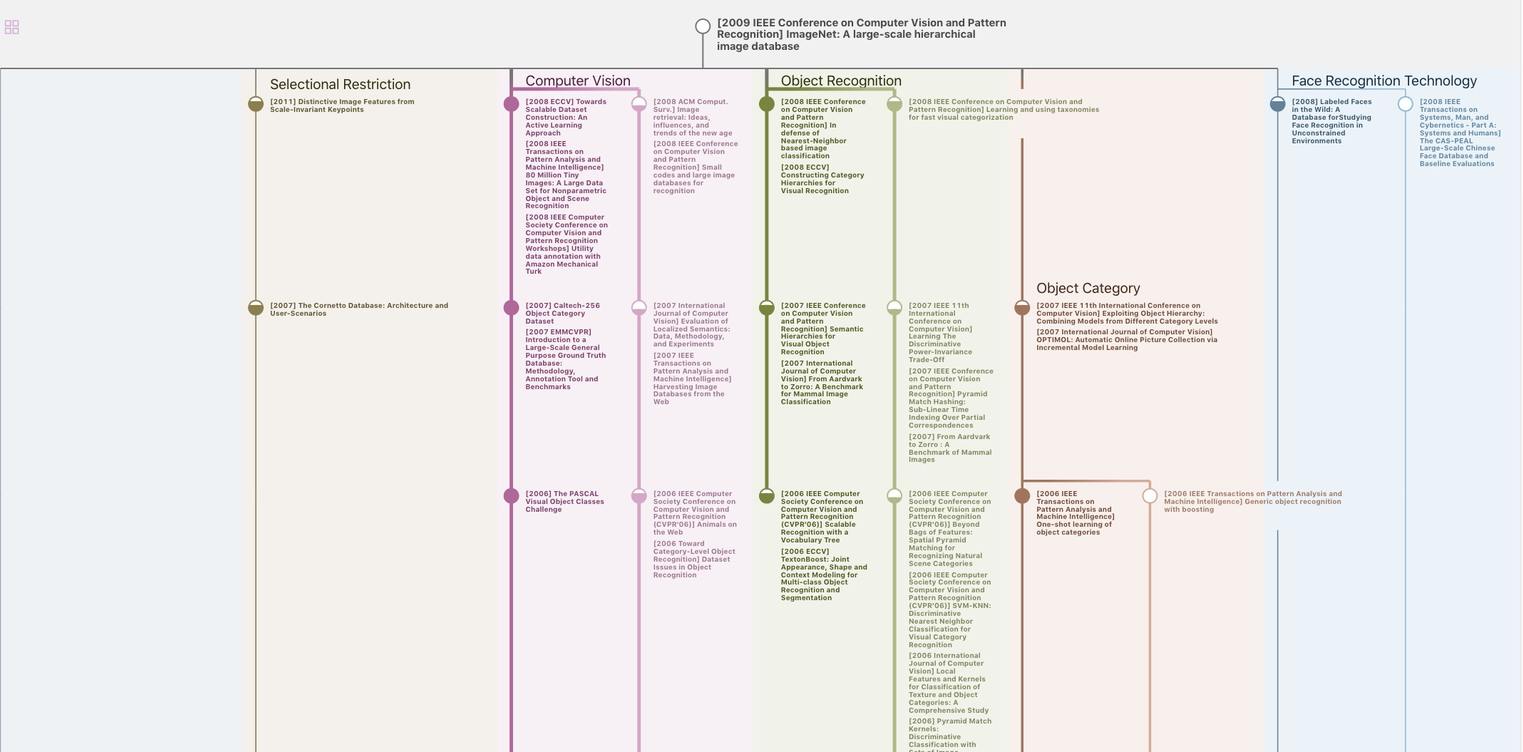
生成溯源树,研究论文发展脉络
Chat Paper
正在生成论文摘要