Deep multiscale convolutional feature learning for weakly supervised localization of chest pathologies in X-ray images
Lecture Notes in Computer Science(2018)
摘要
Localization of chest pathologies in chest X-ray images is a challenging task because of their varying sizes and appearances. We propose a novel weakly supervised method to localize chest pathologies using class aware deep multiscale feature learning. Our method leverages intermediate feature maps from CNN layers at different stages of a deep network during the training of a classification model using image level annotations of pathologies. During the training phase, a set of layer relevance weights are learned for each pathology class and the CNN is optimized to perform pathology classification by convex combination of feature maps from both shallow and deep layers using the learned weights. During the test phase, to localize the predicted pathology, the multiscale attention map is obtained by convex combination of class activation maps from each stage using the layer relevance weights learned during the training phase. We have validated our method using 112000 X-ray images and compared with the state-of-the-art localization methods. We experimentally demonstrate that the proposed weakly supervised method can improve the localization performance of small pathologies such as nodule and mass while giving comparable performance for bigger pathologies e.g., Cardiomegaly
更多查看译文
关键词
Weakly supervised learning,X-ray pathology classification
AI 理解论文
溯源树
样例
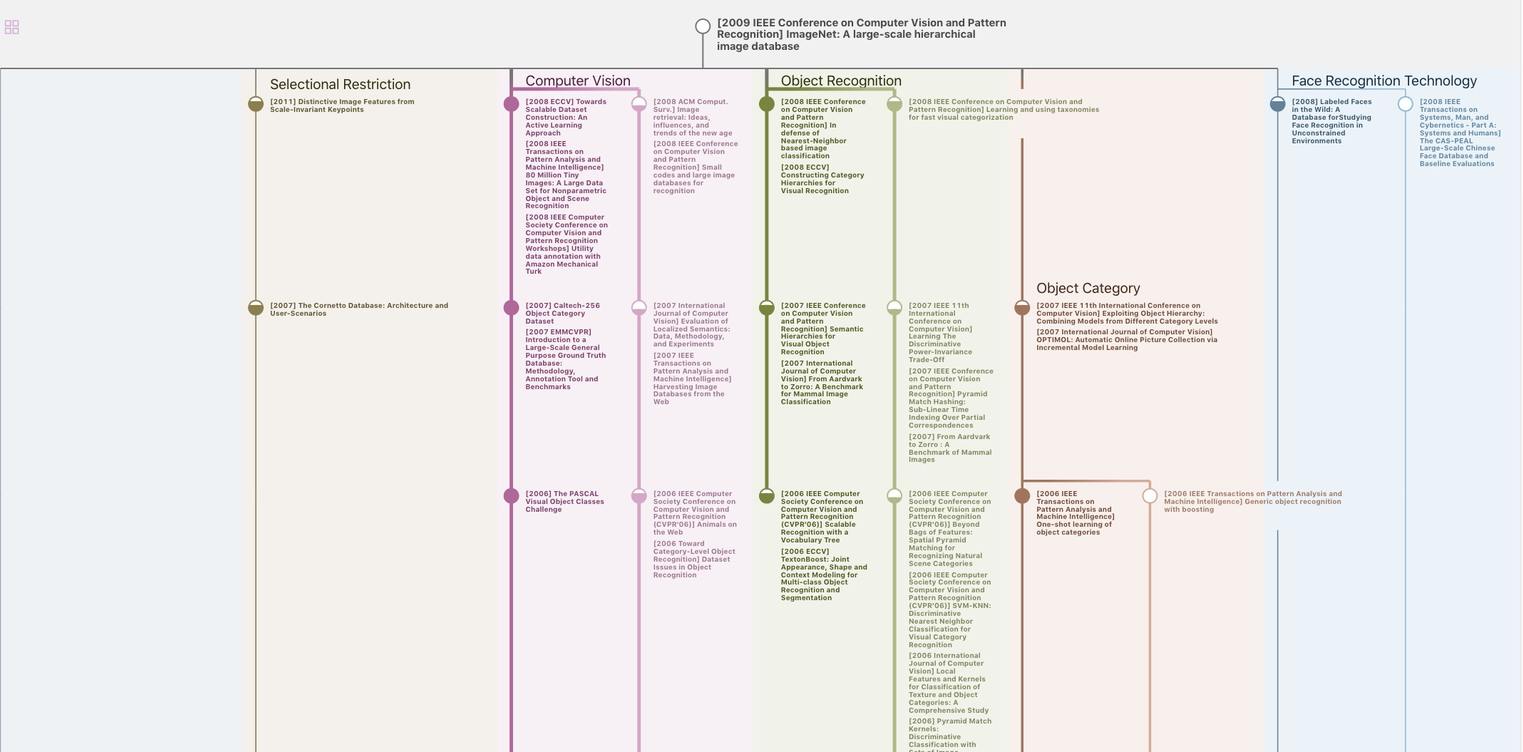
生成溯源树,研究论文发展脉络
Chat Paper
正在生成论文摘要