On the Convergence of Adaptive Gradient Methods for Nonconvex Optimization.
arXiv: Learning(2018)
摘要
Adaptive gradient methods are workhorses in deep learning. However, the convergence guarantees of adaptive gradient methods for nonconvex optimization have not been sufficiently studied. In this paper, we provide a sharp analysis of a recently proposed adaptive gradient method namely partially adaptive momentum estimation method (Padam) (Chen and Gu, 2018), which admits many existing adaptive gradient methods such as AdaGrad, RMSProp and AMSGrad as special cases. Our analysis shows that, for smooth nonconvex functions, Padam converges to a first-order stationary point at the rate of $Obig((sum_{i=1}^d|mathbf{g}_{1:T,i}|_2)^{1/2}/T^{3/4} + d/Tbig)$, where $T$ is the number of iterations, $d$ is the dimension, $mathbf{g}_1,ldots,mathbf{g}_T$ are the stochastic gradients, and $mathbf{g}_{1:T,i} = [g_{1,i},g_{2,i},ldots,g_{T,i}]^top$. Our theoretical result also suggests that in order to achieve faster convergence rate, it is necessary to use Padam instead of AMSGrad. This is well-aligned with the empirical results of deep learning reported in Chen and Gu (2018).
更多查看译文
AI 理解论文
溯源树
样例
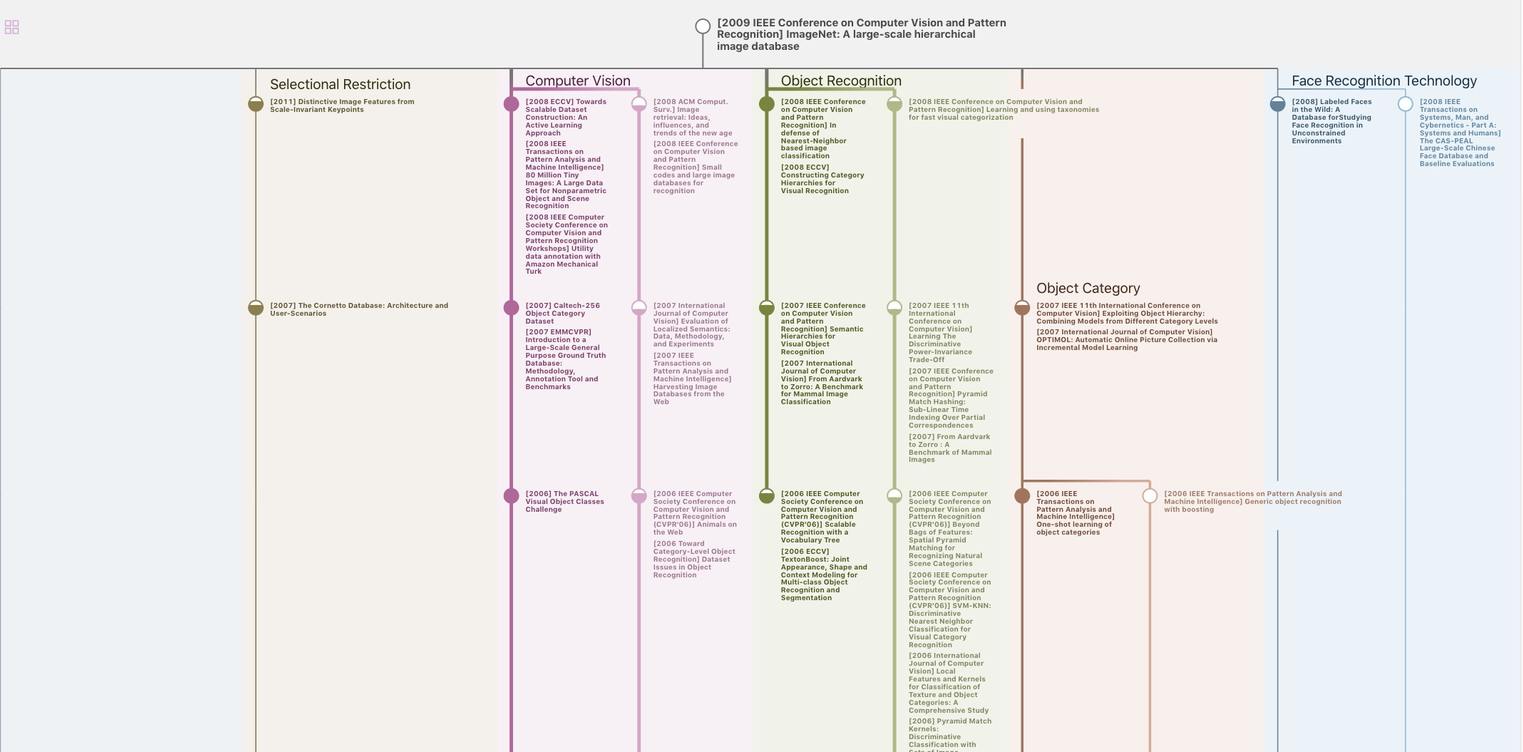
生成溯源树,研究论文发展脉络
Chat Paper
正在生成论文摘要